Workload-driven learning of mallows mixtures with pairwise preference data
WebDB(2016)
摘要
In this paper we present a framework for learning mixtures of Mallows models from large samples of incomplete preferences. The problem we address is of significant practical importance in social choice, recommender systems, and other domains where it is required to aggregate, or otherwise analyze, preferences of a heterogeneous user base. We improve on state-of-the-art methods for learning mixtures of Mallows models with pairwise preference data. Exact sampling from the Mallows posterior in presence of arbitrary pairwise evidence is known to be intractable even for a single Mallows. This motivated to the development of an approximate sampler called AMP. In this paper we propose AMPx, an ensemble method for approximate sampling from the Mallows posterior that combines AMP with frequency-based estimation of posterior probabilities. We experimentally demonstrate that AMPx achieves faster convergence and higher accuracy than AMP alone. We also adapt state-of-the-art clustering techniques that have not been used in this setting, for learning parameters of the Mallows mixture, and show experimentally that mixture parameters can be learned accurately and efficiently.
更多查看译文
AI 理解论文
溯源树
样例
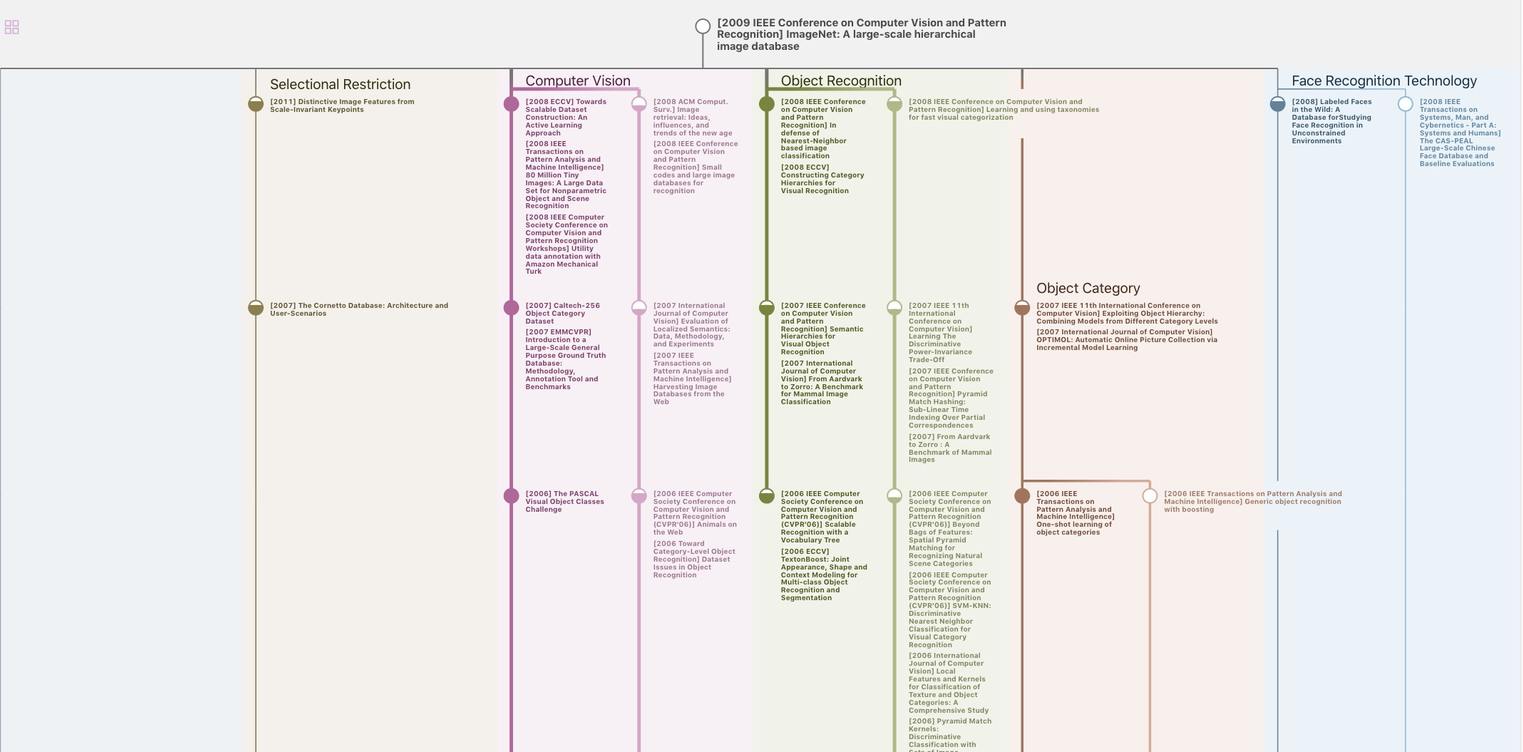
生成溯源树,研究论文发展脉络
Chat Paper
正在生成论文摘要