Slide-specific models for segmentation of differently stained digital histopathology whole slide images.
Proceedings of SPIE(2016)
摘要
The automatic analysis of whole slide images (WSIs) of stained histopathology tissue sections plays a crucial role in the discovery of predictive biomarkers in the field on immuno-oncology by enabling the quantification of the phenotypic information contained in the tissue sections. The automatic detection of cells and nuclei, while being one of the major steps of such analysis, remains a difficult problem because of the low visual differentiation of high pleomorphic and densely cluttered objects and of the diversity of tissue appearance between slides. The key idea of this work is to take advantage of well-differentiated objects in each slide to learn about the appearance of the tissue and in particular about the appearance of low-differentiated objects. We detect well-differentiated objects on a automatically selected set of representative regions, learn slide-specific visual context models, and finally use the resulting posterior maps to perform the final detection steps on the whole slide. The accuracy of the method is demonstrated against manual annotations on a set of differently stained images.
更多查看译文
关键词
Digital pathology,machine learning,nuclei segmentation,cell segmentation
AI 理解论文
溯源树
样例
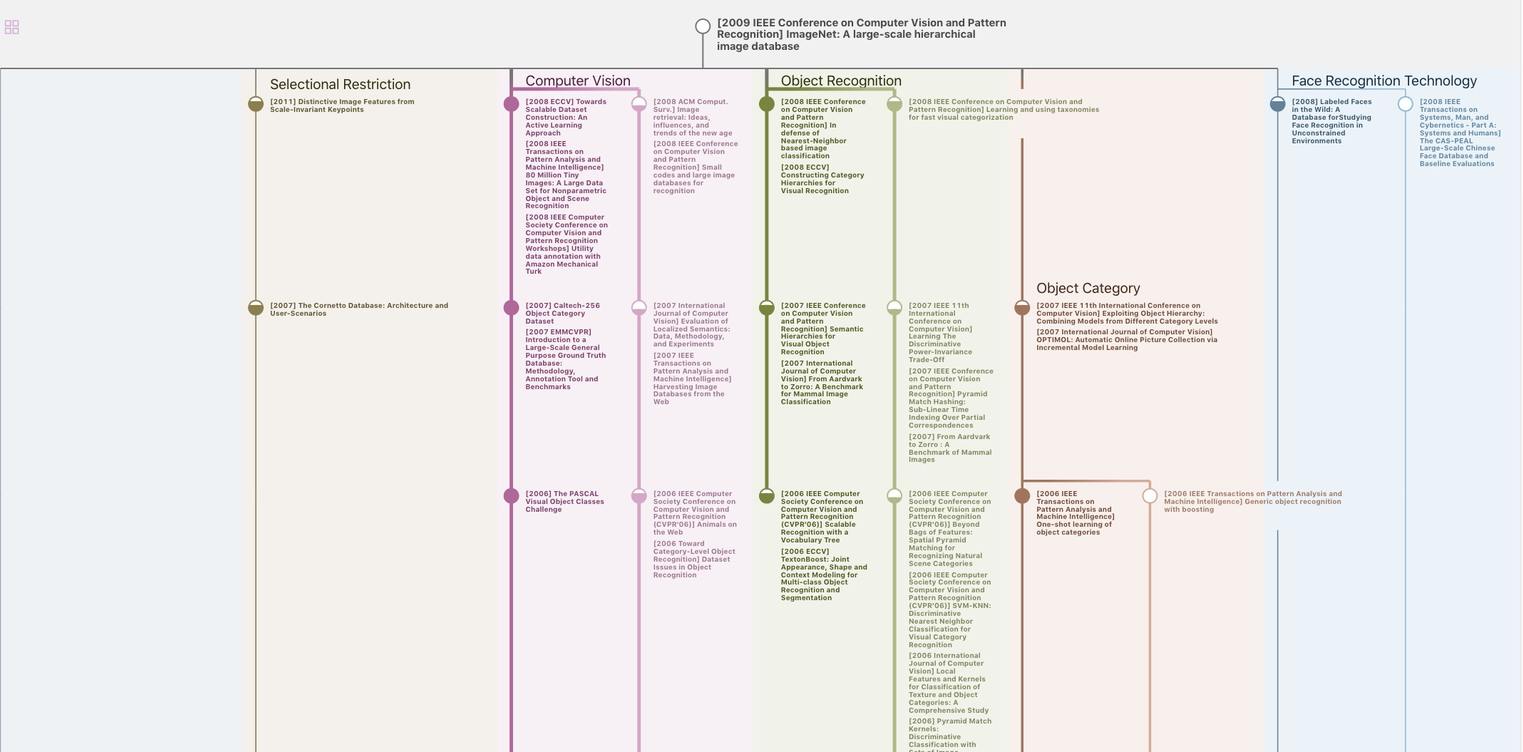
生成溯源树,研究论文发展脉络
Chat Paper
正在生成论文摘要