A learning-based, fully automatic liver tumor segmentation pipeline based on sparsely annotated training data.
Proceedings of SPIE(2016)
摘要
Current fully automatic liver tumor segmentation systems are designed to work on a single CT-image. This hinders these systems from the detection of more complex types of liver tumor. We therefore present a new algorithm for liver tumor segmentation that allows incorporating different CT scans and requires no manual interaction. We derive a liver segmentation with state-of-the-art shape models which are robust to initialization. The tumor segmentation is then achieved by classifying all voxels into healthy or tumorous tissue using Extremely Randomized Trees with an auto-context learning scheme. Using DALSA enables us to learn from only sparse annotations and allows a fast set-up for new image settings. We validate the quality of our algorithm with exemplary segmentation results.
更多查看译文
关键词
Machine Learning,Random Forest,Liver Tumor Segmentation,Auto-Context
AI 理解论文
溯源树
样例
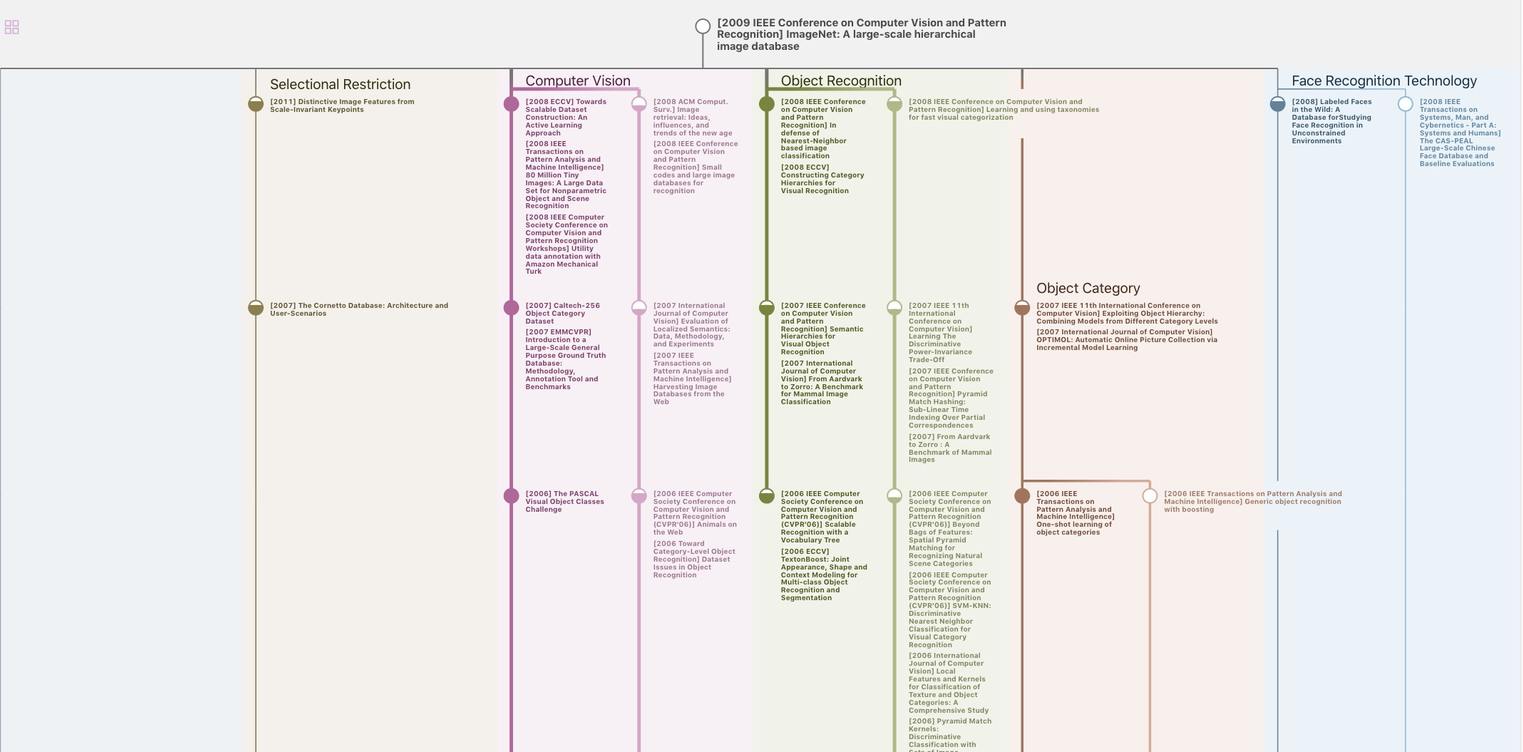
生成溯源树,研究论文发展脉络
Chat Paper
正在生成论文摘要