Learning from redundant but inconsistent reference data: anatomical views and measurements for fetal brain screening.
Proceedings of SPIE(2016)
摘要
In a fetal brain screening examination, a standardized set of anatomical views is inspected and certain biometric measurements are taken in these views. Acquisition of recommended planes requires a certain level of operator expertise. 3D ultrasound has the potential to reduce the manual task to only capture a volume containing the head and to subsequently determine the standard 2D views and measurements automatically. For this purpose, a segmentation model of the fetal brain was created and trained with expert annotations. It was found that the annotations show a considerable intra- and inter-observer variability. To handle the variability, we propose a method to train the model with redundant but inconsistent reference data from many expert users. If the outlier-cleaned average of all reference annotations is considered as ground truth, errors of the automatic view detection are lower than the errors of all individual users and errors of the measurements are in the same range as user error. The resulting functionality allows the completely automated estimation of views and measurements in 3D fetal ultrasound images.
更多查看译文
关键词
Model-based segmentation,ultrasound,fetal screening,fetal brain,automatic measurements
AI 理解论文
溯源树
样例
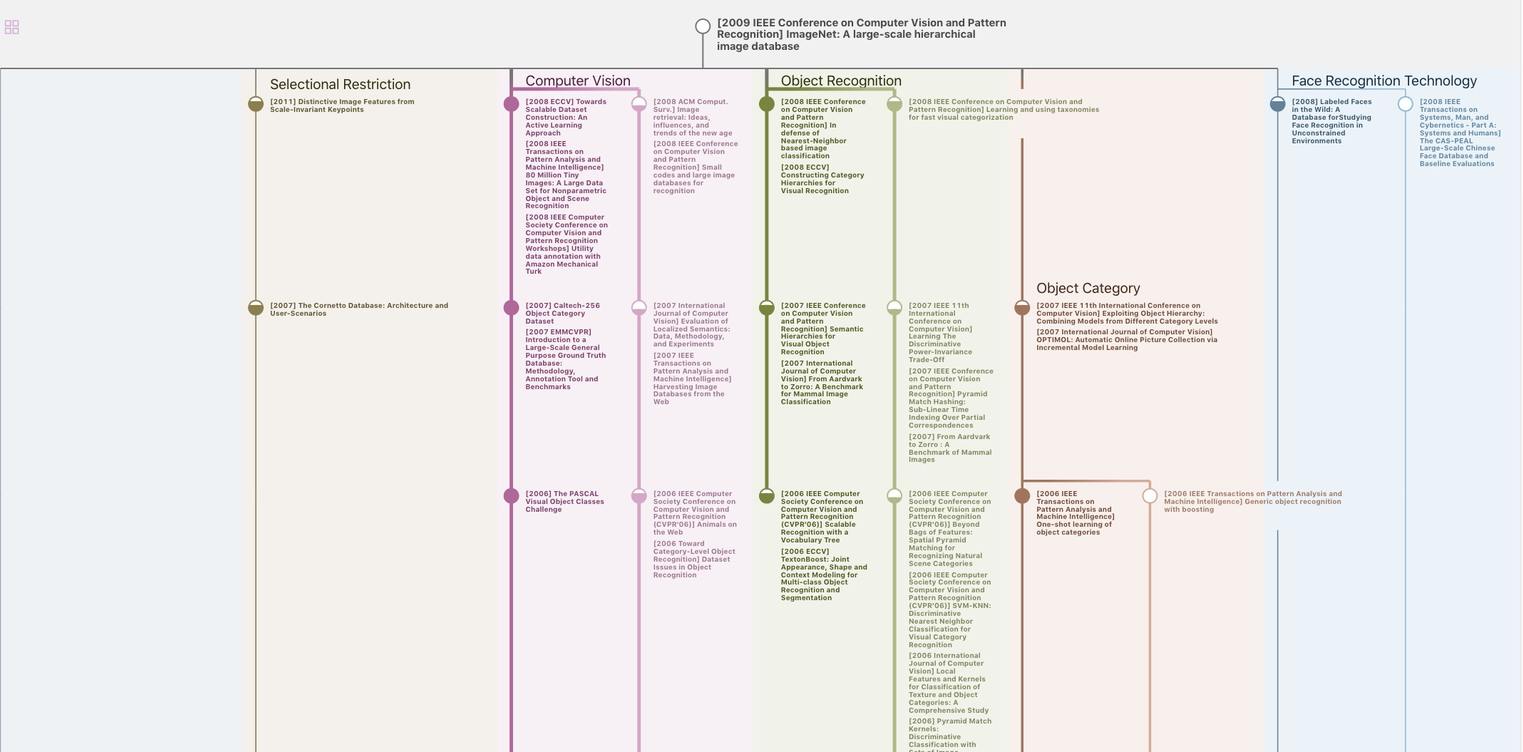
生成溯源树,研究论文发展脉络
Chat Paper
正在生成论文摘要