Boosted Local Classifiers For Visual Tracking
2016 IEEE INTERNATIONAL CONFERENCE ON MULTIMEDIA & EXPO (ICME)(2016)
摘要
Most existing discriminative tracking methods model a target object as a whole and train a tracker based on holistic templates, which cannot effectively deal with partial occlusions. Instead, in this paper, by treating the target as a collection of local patches, we propose a novel tracking approach based on boosted local classifiers. Initially, a set of local patches are sampled to train a set of local classifiers, and the weight of each classifier is given based on the estimated error. In addition, the positive examples and negative examples are sampled for model update with two constraints during the tracking process, which helps obtain more negatives for updating the appearance model and improve the updating efficiency. With updating the weights of local classifiers based on the temporal stability, the tracker can effectively handle partial occlusions. Extensive experiments on various challenging image sequences demonstrate the superiority to several state-ofthe-art methods.
更多查看译文
关键词
boosted local classifiers,partial occlusions,temporal stability
AI 理解论文
溯源树
样例
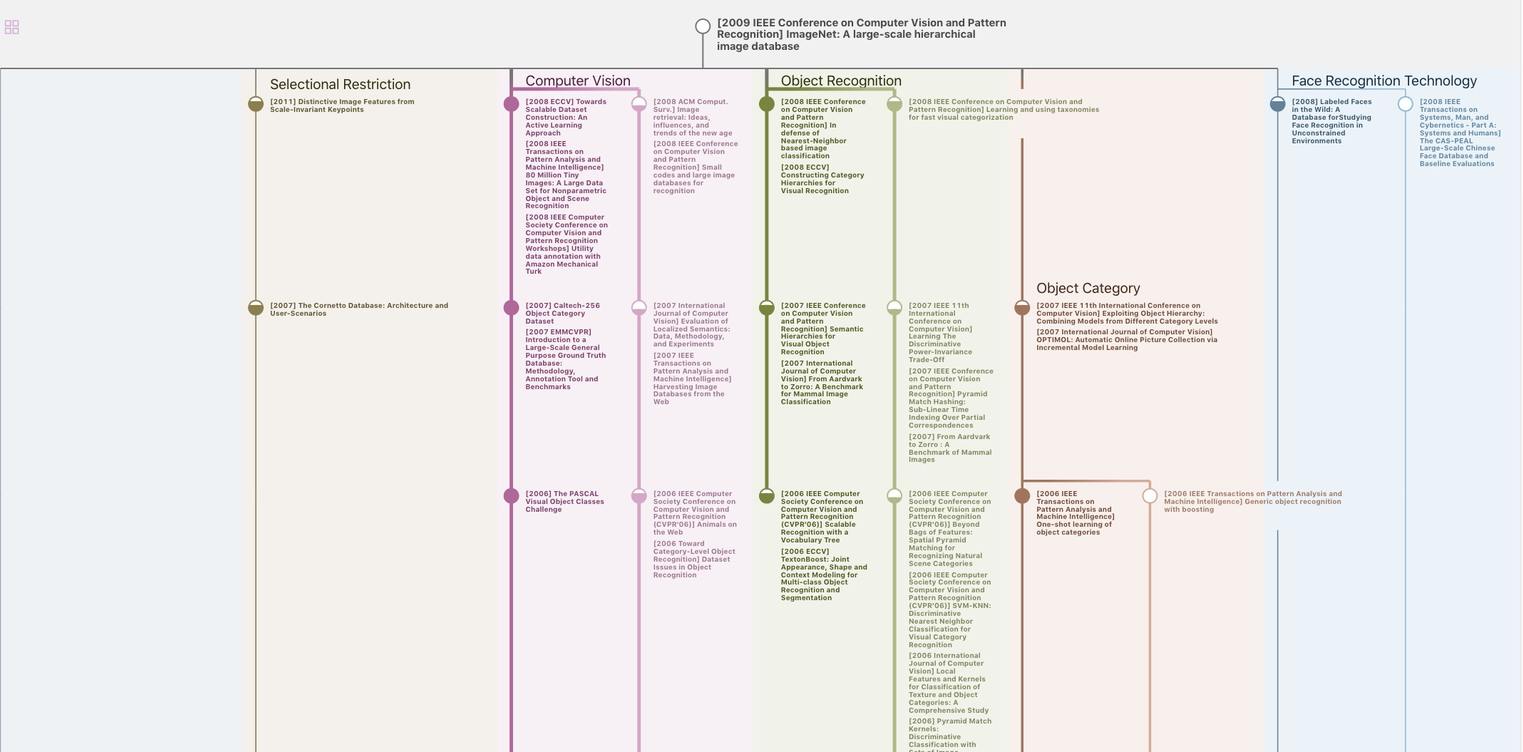
生成溯源树,研究论文发展脉络
Chat Paper
正在生成论文摘要