Accelerated Diffusion-Based Recommendation Algorithm on Tripartite Graphs with GPU Clusters
International Journal of Networked and Distributed Computing(2016)
摘要
Exorbitant computation cost hinders the practical application of recommendation algorithm, especially in time-critical application scenario. Although experiments show that recommendation algorithm based on an integrated diffusion on user-item-tag tripartite graphs can significantly improve accuracy, diversification and novelty of recommendation, it is also very time-consuming. Therefore, a parallel solution is frequently needed to improve the execution speed of the algorithm. This paper explicitly presents the parallel implementation and optimizations of diffusion-based recommendation on weighted tripartite graphs algorithm using Compute Unified Device Architecture and related optimization solutions including accelerated memory access with shared memory, stream scheduling and GPU clusters optimization. Compared to the algorithm running on a single CPU core, the unoptimized GPU kernel can achieve 153.9 speedup on average with the input dataset consists of 30000 records on GTX 980. With shared memory applied, the time cost on device memory access saves about 50
更多查看译文
关键词
Diffusion-Based Recommendation Algorithms on Tripartite Graphs,CUDA,Stream Scheduling,Shared Memory,GPU Clusters
AI 理解论文
溯源树
样例
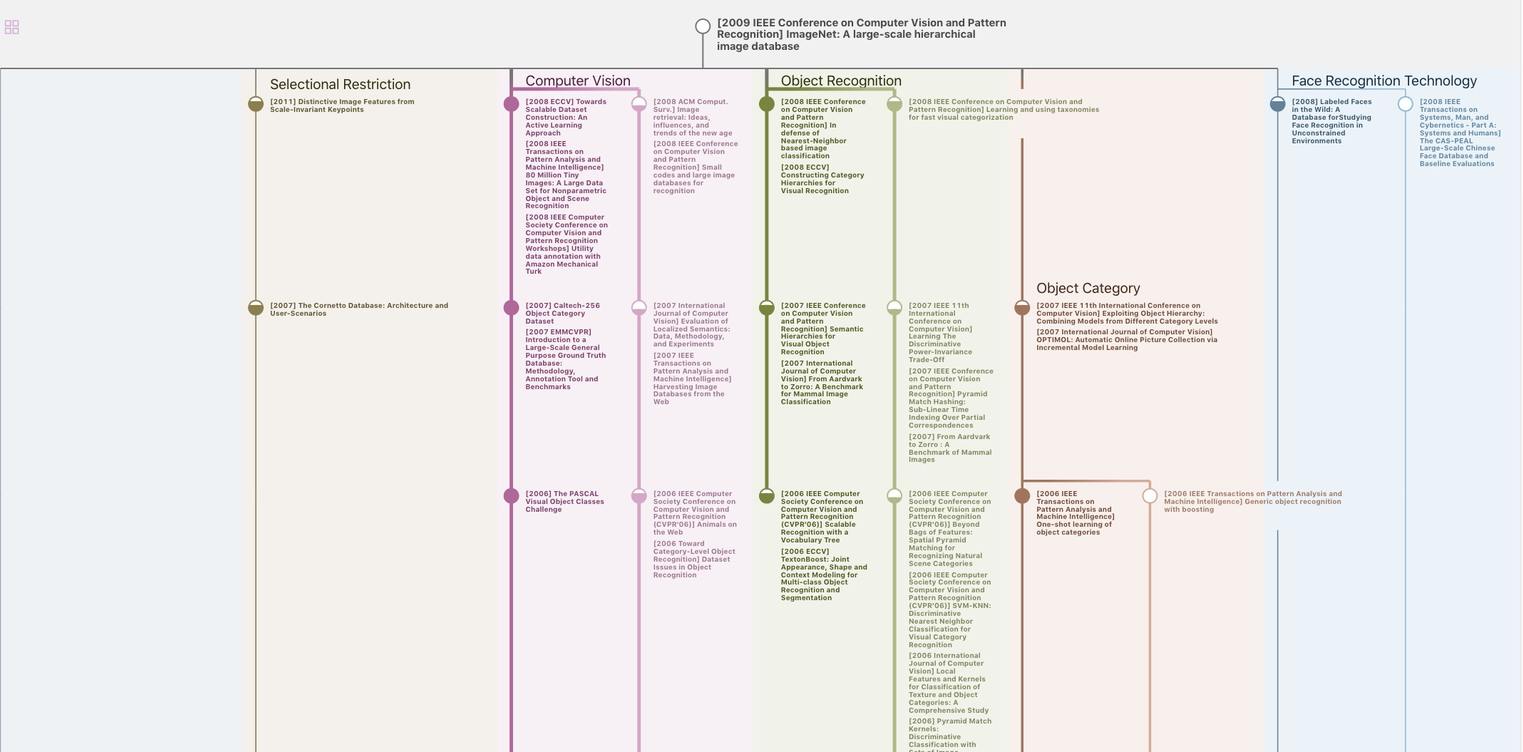
生成溯源树,研究论文发展脉络
Chat Paper
正在生成论文摘要