Wearable Seizure Detection Using Convolutional Neural Networks With Transfer Learning
2016 IEEE International Symposium on Circuits and Systems (ISCAS)(2016)
摘要
The ability to accurately and robustly detect seizures in an ambulatory setting using scalp-based EEG has been the focus of much research over the last several decades. However, its numerous challenges and obstacles have impeded the realization of a definitive solution. This work aims to build upon our existing research and apply newer advanced machine learning and hardware techniques to this issue. The novelty proposed is two-fold. First, we utilize max-pooling convolutional neural networks (MPCNN) to perform end-to-end learning. Second, we demonstrate that transfer-learning can be used to teach MPCNNs generalized features of both normal and epileptiform patterns from a pool of subjects' raw EEG data. Using this hybrid approach, the system is able to detect all 184 seizure onsets from 24 cases with average latency of 1.47 seconds and 3.2 false-alarms/day. To demonstrate the full system, the entire design is efficiently implemented onto a highly parallel, highly granular embedded SoC (NVIDIA Jetson TK1). When utilizing the GPU, the system is able to classify 15-second segments in 308 mu s and last over 80 hours.
更多查看译文
关键词
wearable seizure detection,transfer learning,scalp based EEG,machine learning,max pooling convolutional neural networks,end-to-end learning
AI 理解论文
溯源树
样例
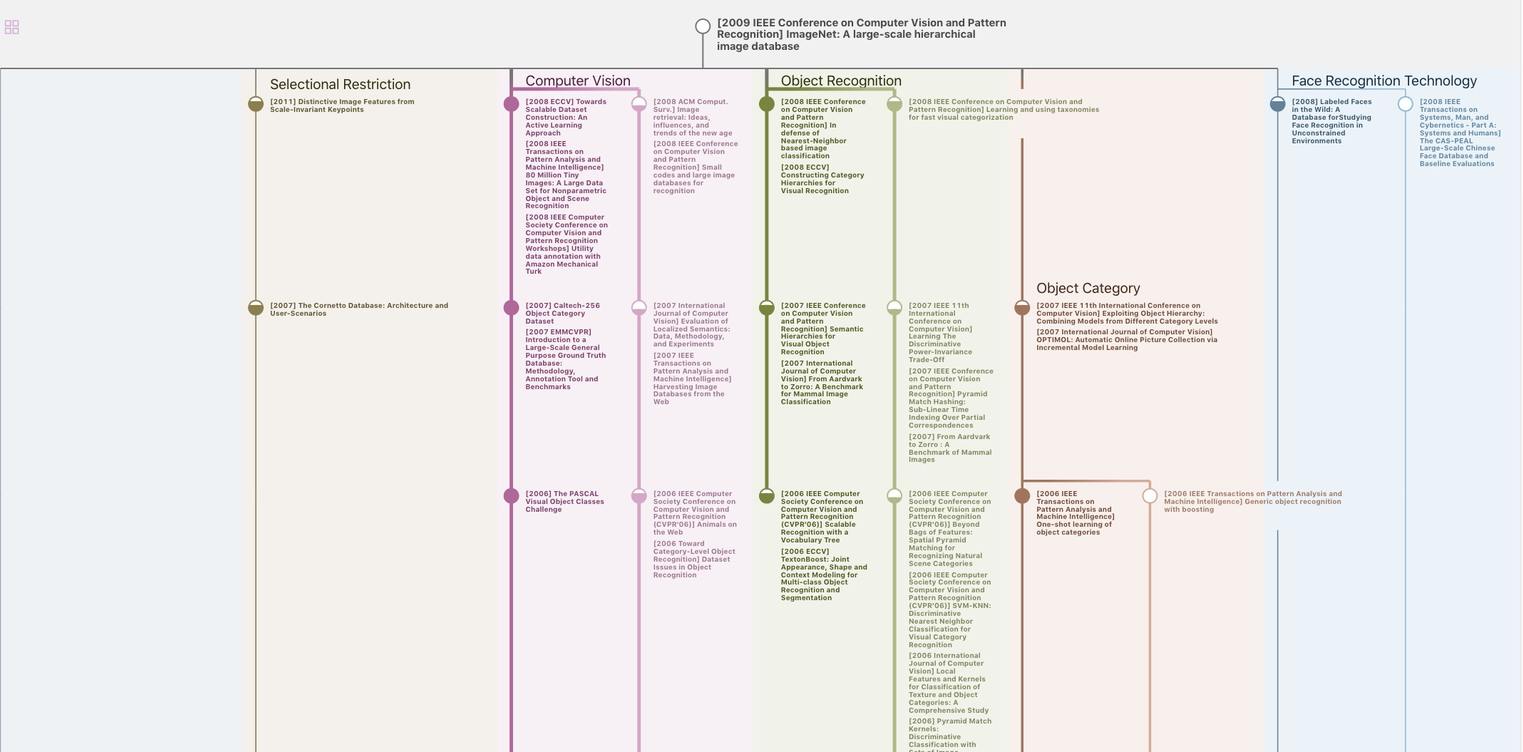
生成溯源树,研究论文发展脉络
Chat Paper
正在生成论文摘要