Learning Decision Trees from Data Streams with Concept Drift.
ICCS(2016)
摘要
This paper addresses a data mining task of classifying data stream with concept drift. The proposed algorithm, named Concept-adapting Evolutionary Algorithm For Decision Tree does not require any knowledge of the environment such as numbers and rates of drifts. The novelty of the approach is combining tree learner and evolutionary algorithm, where the decision tree is learned incrementally and all information is stored in an internal structure of the trees population. The proposed algorithm is experimentally compared with state-of-the-art stream methods on several real live and synthetic datasets. Results indicate its high performance in term of accuracy and processing time.
更多查看译文
关键词
decision tree, adaptive learning, data stream, concept drift
AI 理解论文
溯源树
样例
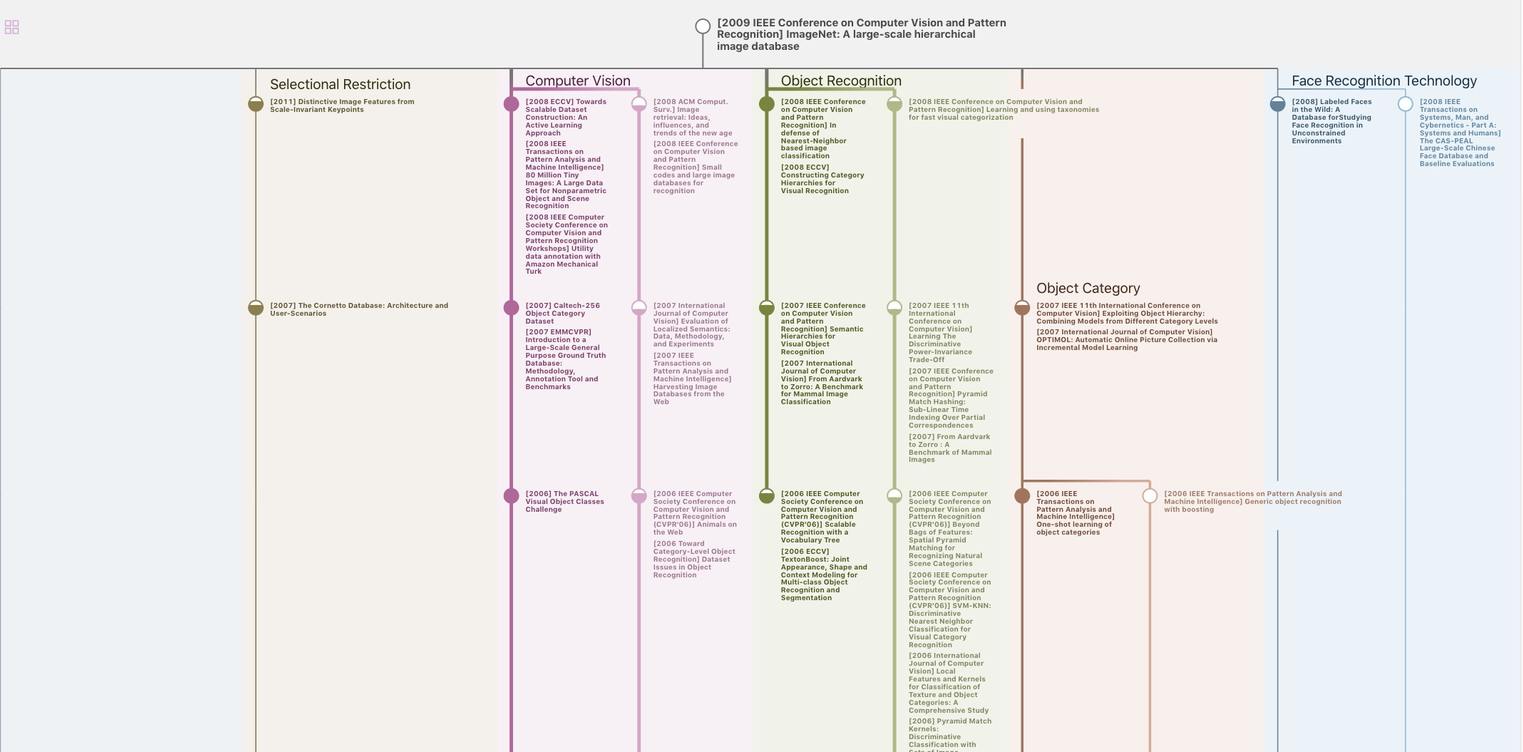
生成溯源树,研究论文发展脉络
Chat Paper
正在生成论文摘要