Supervised Term Weights for Biomedical Text Classification: Improvements in Nearest Centroid Computation.
CIBB(2015)
摘要
Maintaining accessibility of biomedical literature databases has led to development of text classification systems to assist human indexers by recommending thematic categories to biomedical articles. These systems rely on using machine learning methods to learn the association between the document terms and predefined categories. The accuracy of a text classification method depends on the metric used in order to assign a weight to each term. Weighting metrics can be classified as supervised or unsupervised according to whether they use prior information on the number of documents belonging to each category. In this paper, we propose two supervised weighting metrics (One-way Klosgen and Loevinger) which both improve the quality of biomedical document classification. We also show that by using moment generating function centroids, an alternative to the traditional arithmetical average centroids, a nearest centroid classifier with Loevinger metric performs significantly better than SVM on a biomedical text classification task.
更多查看译文
关键词
Support Vector Machine, Text Classification, Term Frequency, Term Weighting, Support Vector Machine Classification
AI 理解论文
溯源树
样例
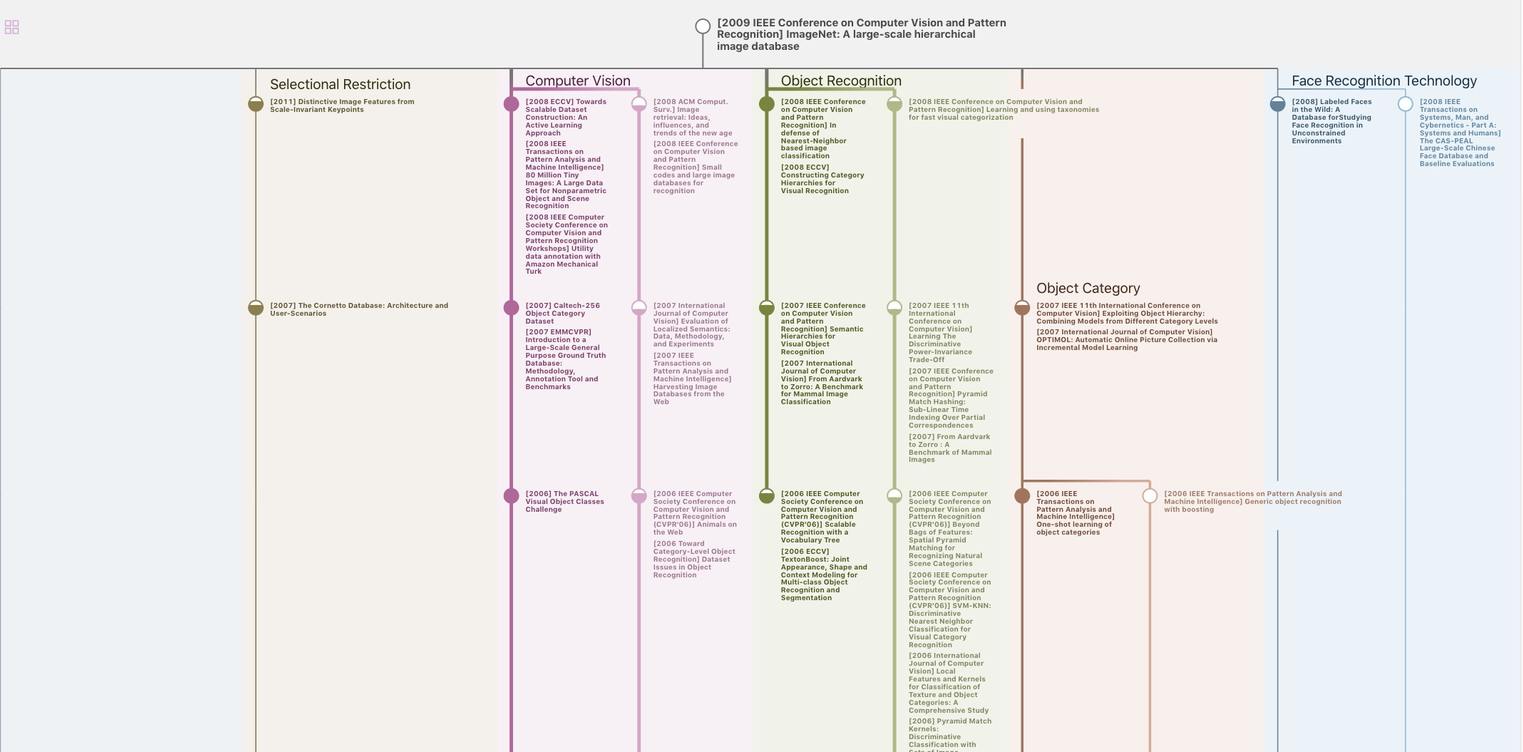
生成溯源树,研究论文发展脉络
Chat Paper
正在生成论文摘要