Joint Acoustic Factor Learning For Robust Deep Neural Network Based Automatic Speech Recognition
2016 IEEE International Conference on Acoustics, Speech and Signal Processing (ICASSP)(2016)
摘要
Deep neural networks (DNNs) for acoustic modeling have been shown to provide impressive results on many state-of-the-art automatic speech recognition (ASR) applications. However, DNN performance degrades due to mismatches in training and testing conditions and thus adaptation is necessary. In this paper, we explore the use of discriminative auxiliary input features obtained using joint acoustic factor learning for DNN adaptation. These features are derived from a bottleneck (BN) layer of a DNN and are referred to as BN vectors. To derive these BN vectors, we explore the use of two types of joint acoustic factor learning which capture speaker and auxiliary information such as noise, phone and articulatory information of speech. In this paper, we show that these BN vectors can be used for adaptation and thereby improve the performance of an ASR system. We also show that the performance can be further improved on augmenting these BN vectors to conventional i-vectors. In this paper, experiments are performed on Aurora-4, REVERB challenge and AMI databases.
更多查看译文
关键词
deep neural networks,joint factor learning,adaptation,bottleneck vectors,robust speech recognition
AI 理解论文
溯源树
样例
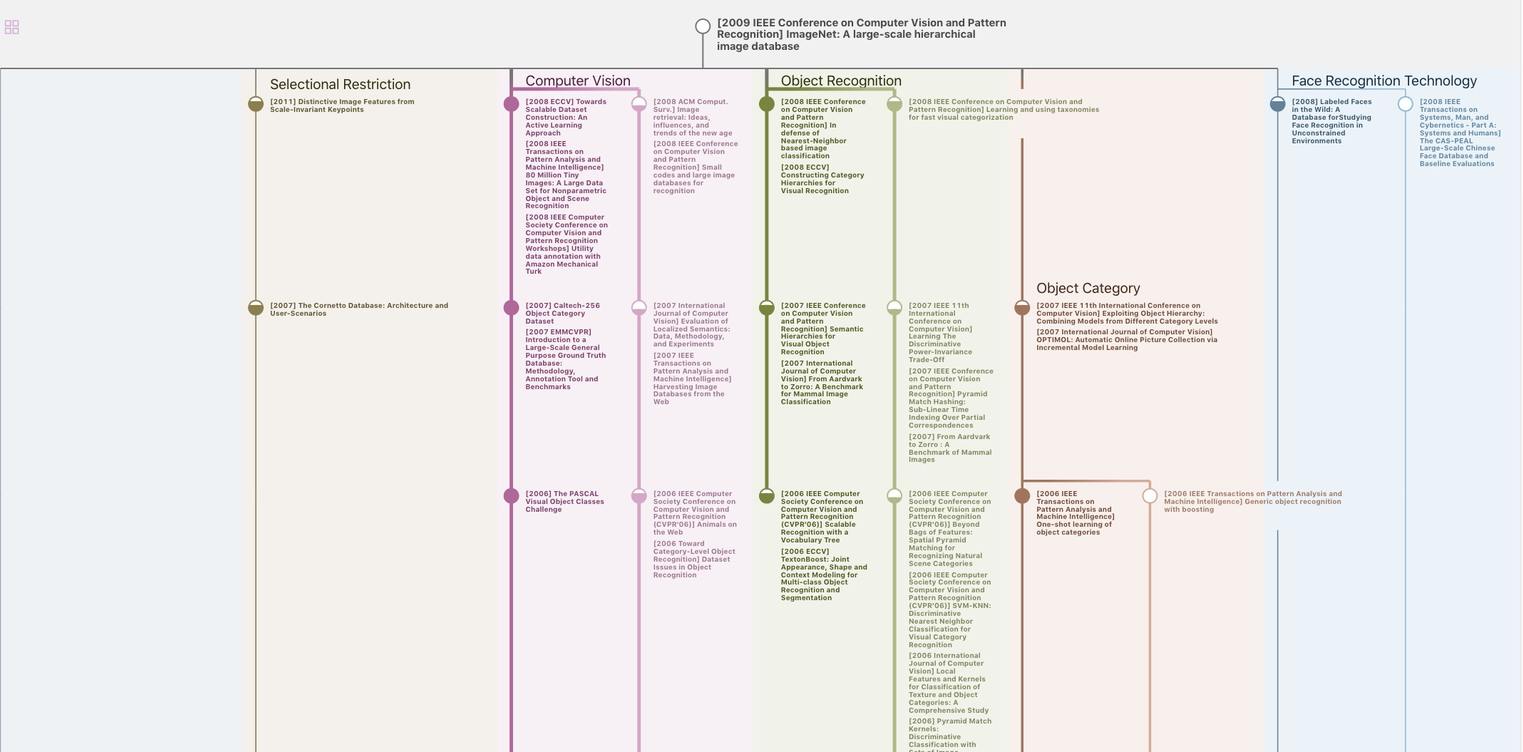
生成溯源树,研究论文发展脉络
Chat Paper
正在生成论文摘要