Blind Deconvolution Of Sparse But Filtered Pulses With Linear State Space Models
2016 IEEE International Conference on Acoustics, Speech and Signal Processing (ICASSP)(2016)
摘要
The paper considers the problem of joint system identification and input signal estimation of an unknown linear system from noisy observations of the output signal. The input signal is assumed to be sparse, and each individual input pulse may affect the system in its own (and unknown) way. Based on ideas from sparse Bayesian learning, we derive an efficient expectation maximization (EM) algorithm for jointly estimating all unknown quantities. Unlike related prior work, the proposed algorithm does not alternate between estimating the input signal and estimating the system parameters; instead, all unknown quantities are jointly updated in each EM step. We give closed-form expressions for these EM updates, which can be efficiently computed by Gaussian message passing.
更多查看译文
关键词
linear state space models,sparse Bayesian learning,expectation maximization
AI 理解论文
溯源树
样例
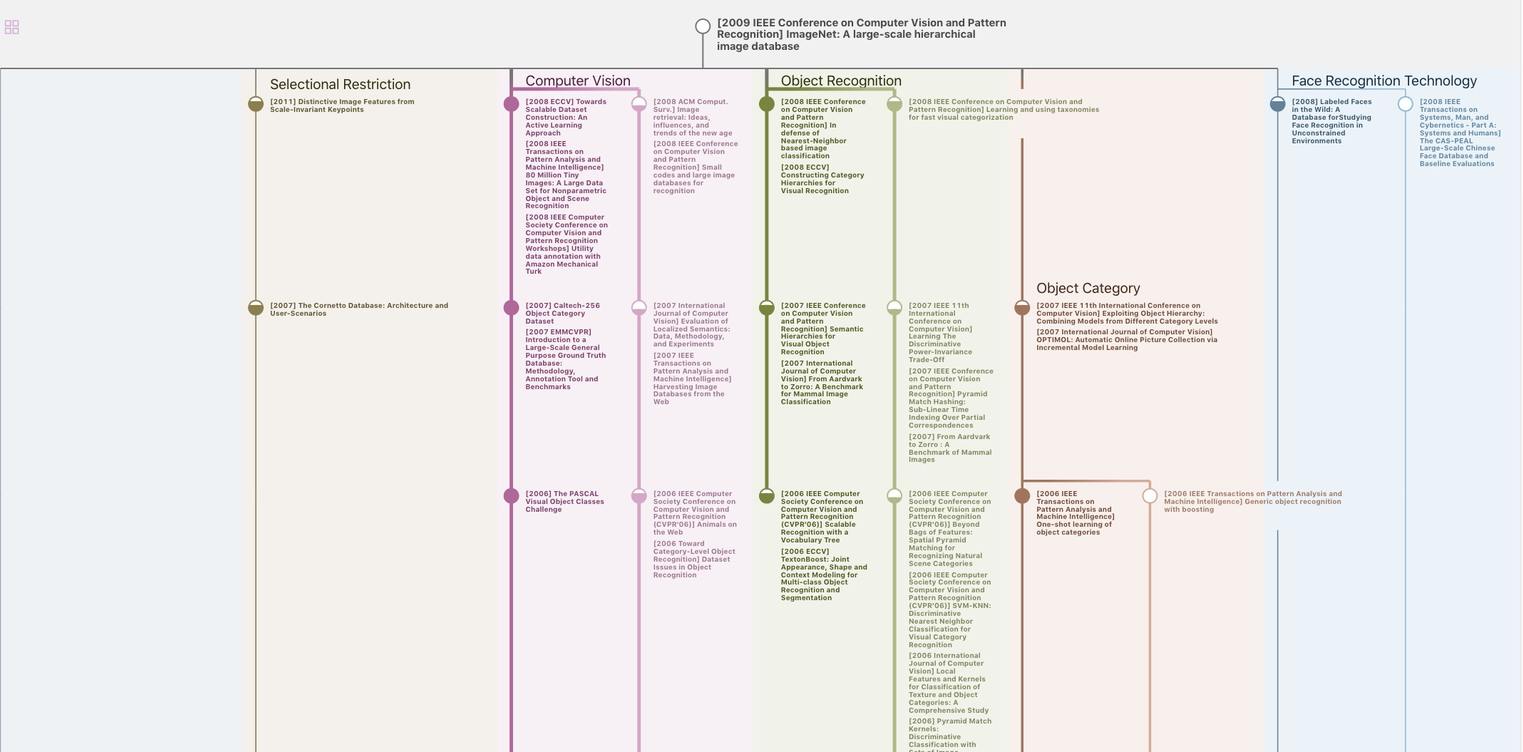
生成溯源树,研究论文发展脉络
Chat Paper
正在生成论文摘要