How Neural Network Features And Depth Modify Statistical Properties Of Hmm Acoustic Models
2016 IEEE International Conference on Acoustics, Speech and Signal Processing (ICASSP)(2016)
摘要
Tandem neural network features, especially ones trained with more than one hidden layer, have improved word recognition performance, but why these features improve automatic speech recognition systems is not completely understood. In this work, we study how neural network features cope with the mismatch between the underlying stochastic process inherent in speech, and the models we use to represent that process. We use a novel resampling framework, which re-samples test set data to match the conditional independence assumptions of the acoustic model, and measure performance as we break those assumptions. We discover that depth provides modest robustness to data/model mismatch at the state level, and compared to standard MFCC features, neural network features actually fix poor duration modeling assumptions of the HMM. The duration modeling problem is also fixed by the language model, suggesting that the dictionary and language model make very strong implicit assumptions about phone length, which may now need to be revisited.
更多查看译文
关键词
Neural Networks,Deep Learning,Tandem Features,Hidden Markov Models
AI 理解论文
溯源树
样例
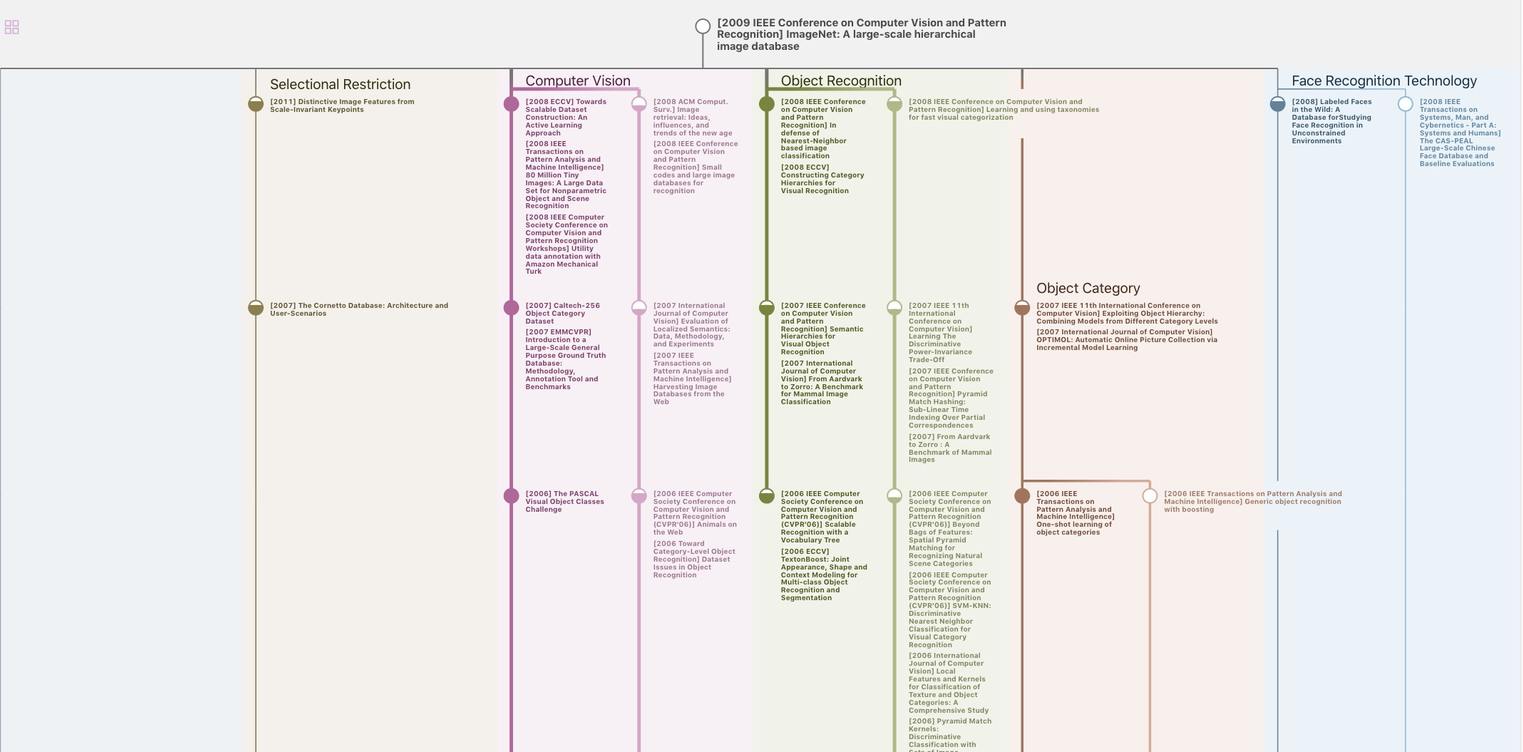
生成溯源树,研究论文发展脉络
Chat Paper
正在生成论文摘要