Sequence Training Of Multi-Task Acoustic Models Using Meta-State Labels
2016 IEEE International Conference on Acoustics, Speech and Signal Processing (ICASSP)(2016)
摘要
In this paper, we describe a multi-task learning approach for acoustic modeling where the multiple output layers are used to predict context-dependent (CD) states from different state inventories. Unlike the traditional multitask learning approach which defines a primary and secondary output layers but discards the secondary output after training, we propose to use all output layers for recognition. This can be achieved by designing a decoding network operating on tuples of CD states and combining the scores of the different outputs during search. To support training such models using a sequence-based criterion, we propose to replace the multiple output layers with a single layer encoding the CD state tuples as "meta-states". Experimental results are given on a large Voice Search task evaluated on children's speech.
更多查看译文
关键词
system combination,multitask learning,sequence training,children's speech
AI 理解论文
溯源树
样例
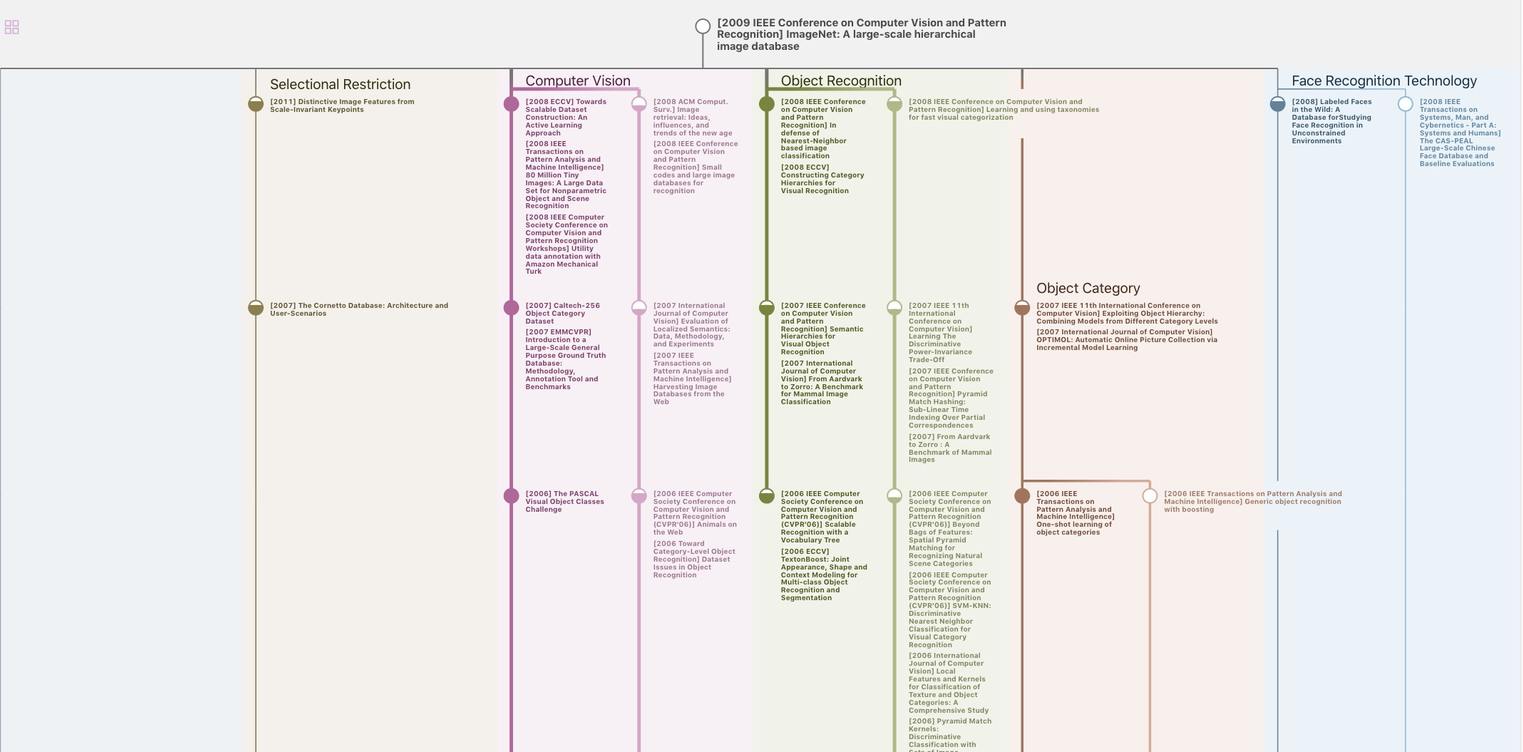
生成溯源树,研究论文发展脉络
Chat Paper
正在生成论文摘要