Distributed Generalized Likelihood Ratio Tests : Fundamental Limits And Tradeoffs
2016 IEEE International Conference on Acoustics, Speech and Signal Processing (ICASSP)(2016)
摘要
This paper focuses on the problem of distributed composite hypothesis testing in a network of sparsely interconnected agents, in which only a small section of the field modeling parametric alternatives is observable at each agent. A recursive generalized likelihood ratio test (GLRT) type algorithm in a distributed setup of the consensus-plus-innovations form is proposed, in which the agents update their parameter estimates and decision statistics by simultaneously processing the latest sensed information (innovations) and information obtained from neighboring agents (consensus). This paper characterizes the conditions and the testing algorithm design parameters which ensure that the probabilities of decision errors decay to zero asymptotically in the large sample limit. Finally, simulation studies are presented which illustrate the findings.
更多查看译文
关键词
Distributed Inference,Consensus Algorithms,Generalized Likelihood Ratio Tests,Hypothesis Testing,Large Deviations Analysis
AI 理解论文
溯源树
样例
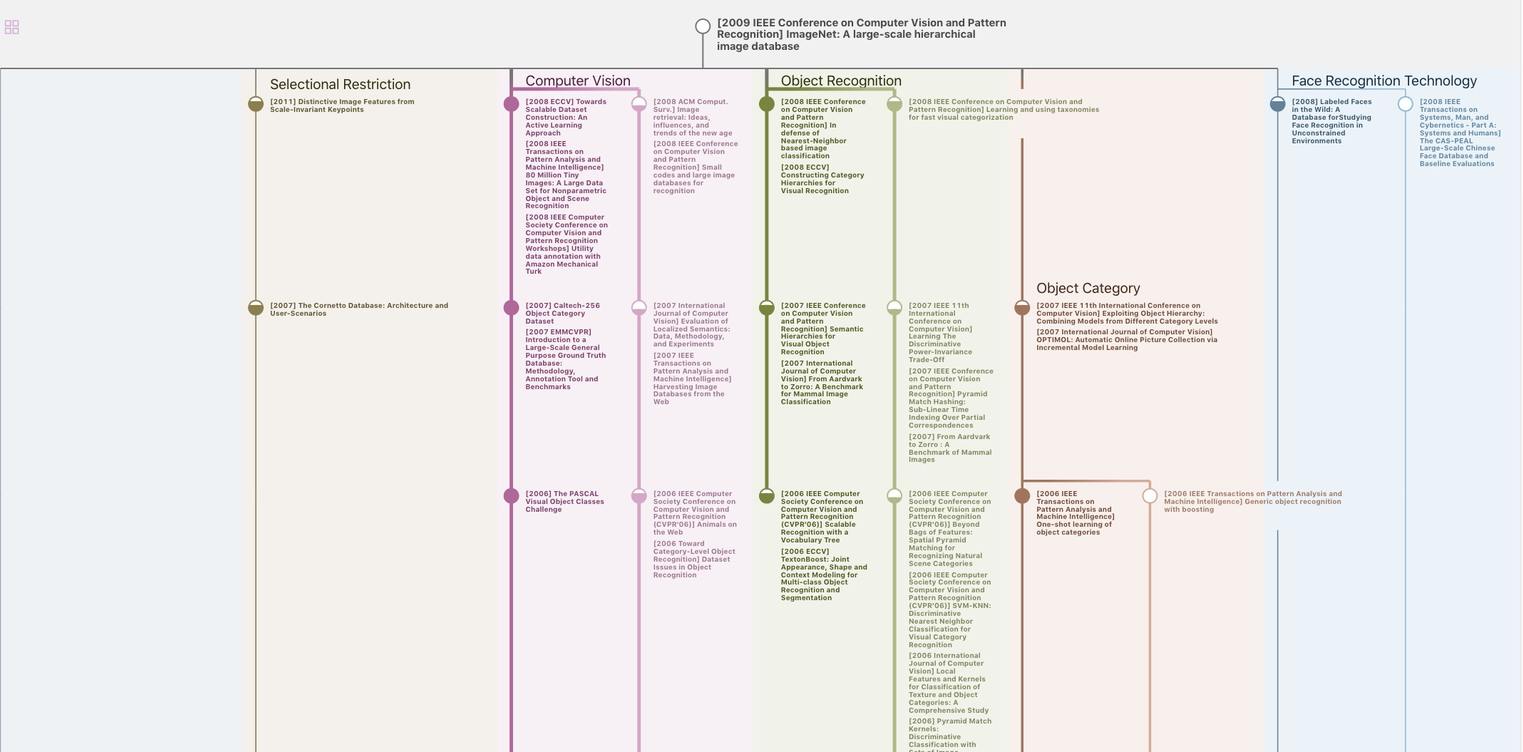
生成溯源树,研究论文发展脉络
Chat Paper
正在生成论文摘要