A Metropolis-within-Gibbs sampler to infer task-based functional brain connectivity.
ICASSP(2016)
摘要
Examining the dynamic aspects of functional networks in the brain is imperative in order to obtain a thorough description and to gain a better insight into its several features. Present methods of analysing brain data in task-conditions mainly include concatenation followed by temporal correlation. We employ Markov Chain Monte Carlo methods, namely Metropolis within Gibbs sampling, on a stochastic model to infer dynamic functional connectivity in such conditions. By using a Bayesian probabilistic framework, distributional estimates of the linkage strengths are obtained as opposed to point estimates, and the uncertainty of the existence of such links is accounted for. The methodology is applied to fMRI data from a finger opposition paradigm with task and fixation conditions, investigating the dynamics of the well characterised somato-motor network while using the visual network as a control case.
更多查看译文
关键词
Gibbs sampling,Metropolis-Hastings,ROI,fMRI,functional connectivity
AI 理解论文
溯源树
样例
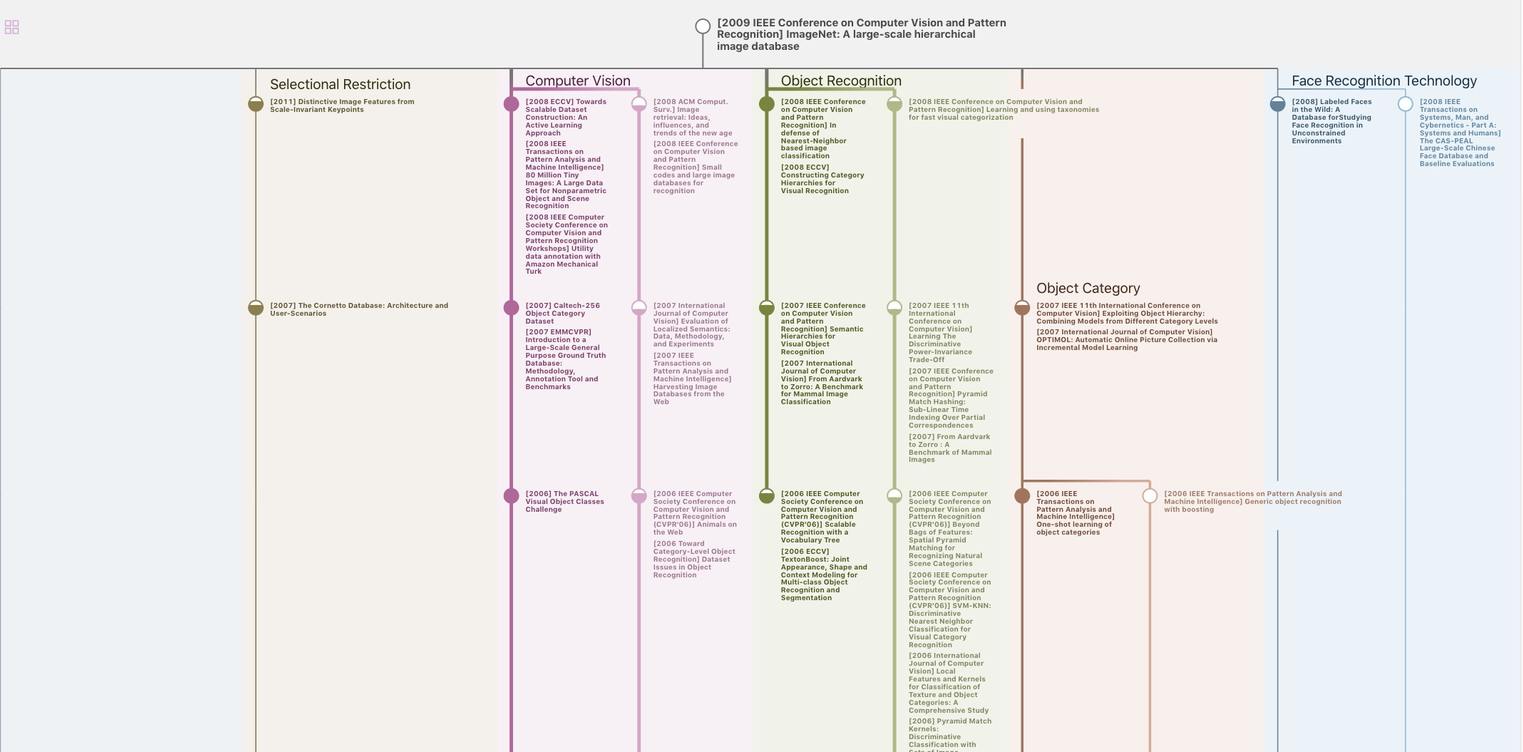
生成溯源树,研究论文发展脉络
Chat Paper
正在生成论文摘要