Back to the Future: Radial Basis Function Network Revisited
IEEE Transactions on Pattern Analysis and Machine Intelligence(2020)
摘要
Radial Basis Function (RBF) networks are a classical family of algorithms for supervised learning. The most popular approach for training RBF networks has relied on kernel methods using regularization based on a norm in a Reproducing Kernel Hilbert Space (RKHS), which is a principled and empirically successful framework. In this paper we aim to revisit some of the older approaches to training the RBF networks from a more modern perspective. Specifically, we analyze two common regularization procedures, one based on the square norm of the coefficients in the network and another one using centers obtained by
$k$
-means clustering. We show that both of these RBF methods can be recast as certain data-dependent kernels. We provide a theoretical analysis of these methods as well as a number of experimental results, pointing out very competitive experimental performance as well as certain advantages over the standard kernel methods in terms of both flexibility (incorporating of unlabeled data) and computational complexity. Finally, our results shed light on some impressive recent successes of using soft
$k$
-means features for image recognition and other tasks.
更多查看译文
关键词
Kernel,Radial basis function networks,Training,Standards,Loss measurement,Training data,Supervised learning
AI 理解论文
溯源树
样例
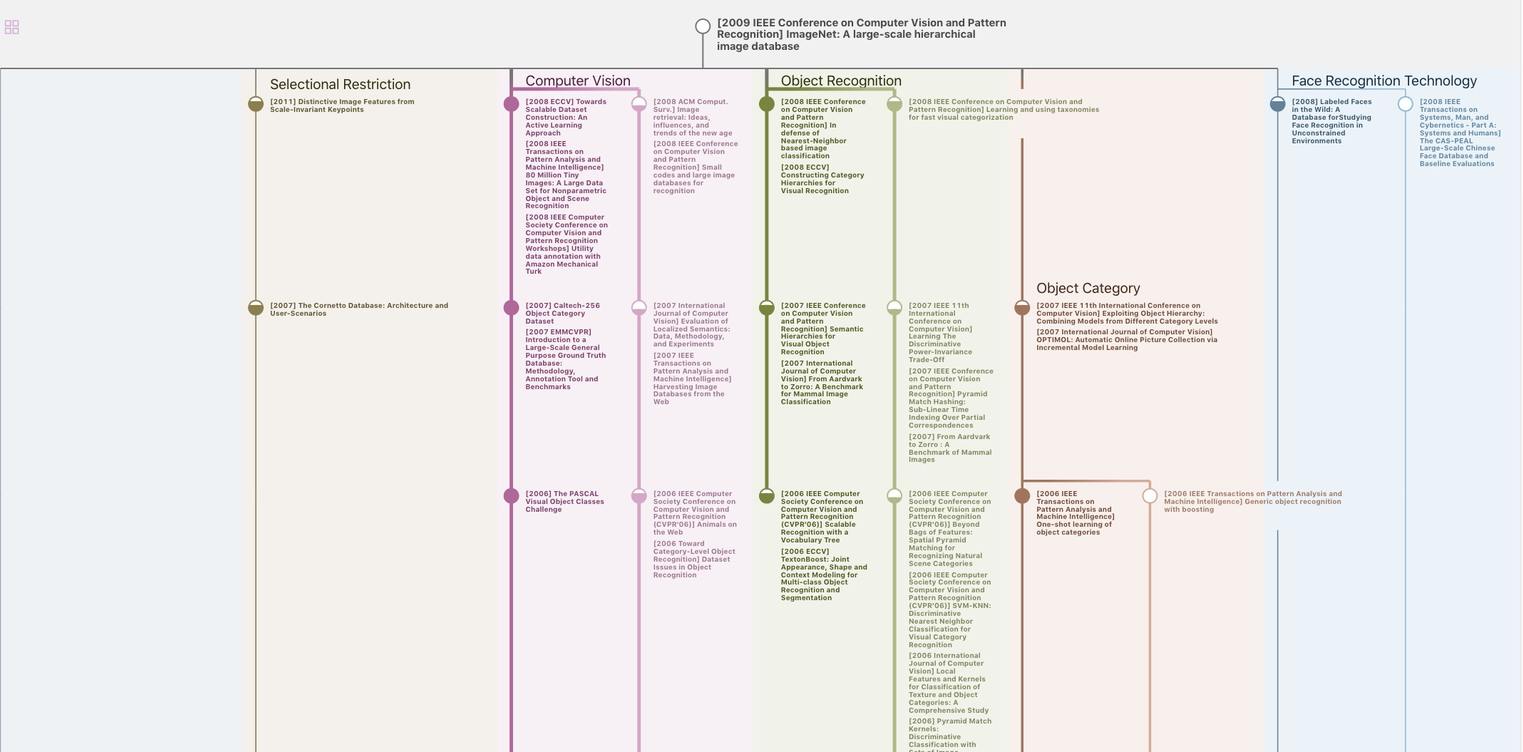
生成溯源树,研究论文发展脉络
Chat Paper
正在生成论文摘要