Integration Of Unsupervised And Supervised Criteria For Deep Neural Networks Training
ARTIFICIAL NEURAL NETWORKS AND MACHINE LEARNING - ICANN 2016, PT II(2016)
摘要
Training Deep Neural Networks has been a difficult task for a long time. Recently diverse approaches have been presented to tackle these difficulties, showing that deep models improve the performance of shallow ones in some areas like signal processing, signal classification or signal segmentation, whatever type of signals, e.g. video, audio or images. One of the most important methods is greedy layer-wise unsupervised pre-training followed by a fine-tuning phase. Despite the advantages of this procedure, it does not fit some scenarios where real time learning is needed, as for adaptation of some time-series models. This paper proposes to couple both phases into one, modifying the loss function to mix together the unsupervised and supervised parts. Benchmark experiments with MNIST database prove the viability of the idea for simple image tasks, and experiments with time-series forecasting encourage the incorporation of this idea into on-line learning approaches. The interest of this method in time-series forecasting is motivated by the study of predictive models for domotic houses with intelligent control systems.
更多查看译文
关键词
Deep learning, Stacked auto-encoders, Supervised learning, Time-series forecasting
AI 理解论文
溯源树
样例
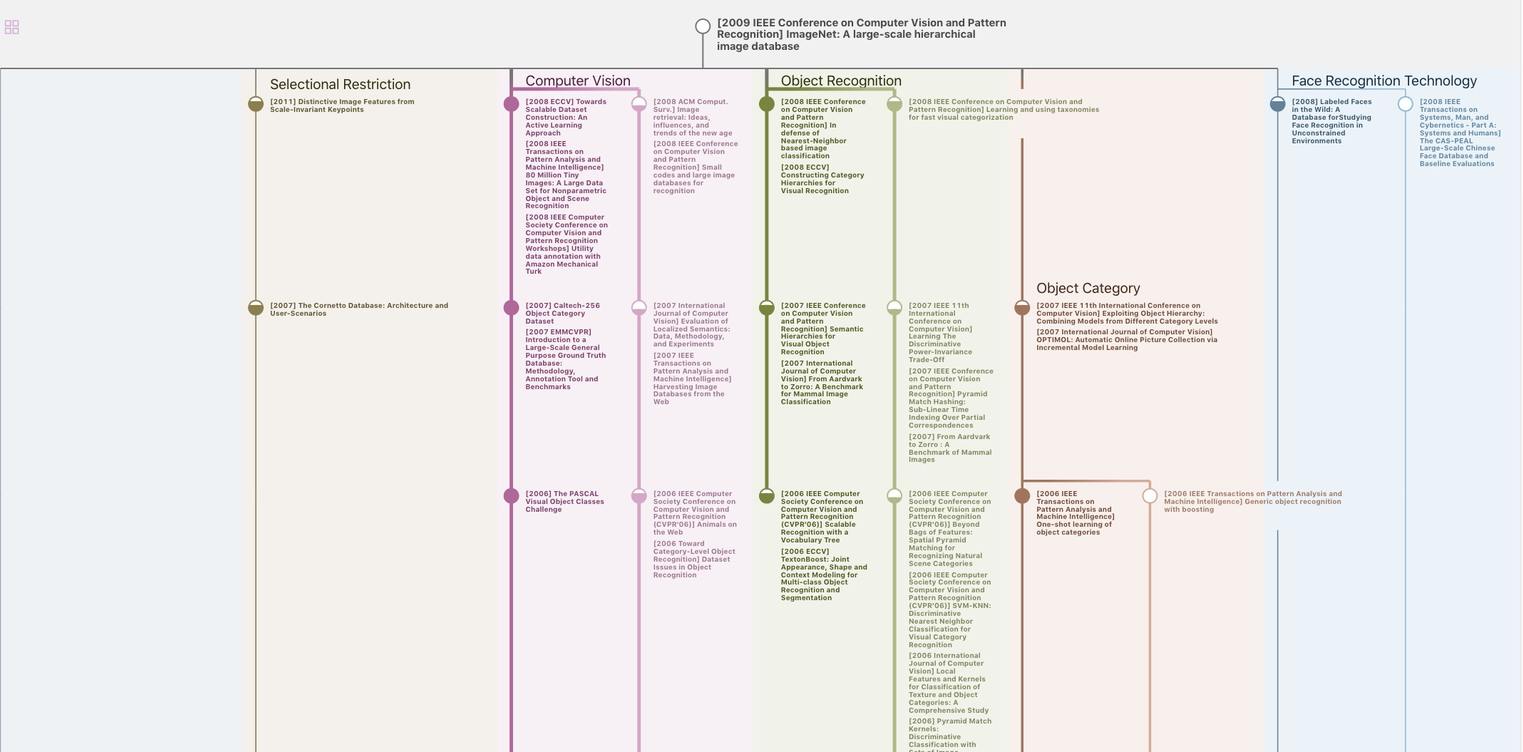
生成溯源树,研究论文发展脉络
Chat Paper
正在生成论文摘要