Adaptive Bi-Objective Genetic Programming For Data-Driven System Modeling
INTELLIGENT COMPUTING METHODOLOGIES, ICIC 2016, PT III(2016)
摘要
We propose in this paper a modification of one of the modern state-of-the-art genetic programming algorithms used for data-driven modeling, namely the Bi-objective Genetic Programming (BioGP). The original method is based on a concurrent minimization of both the training error and complexity of multiple candidate models encoded as Genetic Programming trees. Also, BioGP is empowered by a predator-prey co-evolutionary model where virtual predators are used to suppress solutions (preys) characterized by a poor trade-off error vs complexity. In this work, we incorporate in the original BioGP an adaptive mechanism that automatically tunes the mutation rate, based on a characterization of the current population (in terms of entropy) and on the information that can be extracted from it. We show through numerical experiments on two different datasets from the energy domain that the proposed method, named BioAGP (where "A" stands for "Adaptive"), performs better than the original BioGP, allowing the search to maintain a good diversity level in the population, without affecting the convergence rate.
更多查看译文
关键词
Multi-objective evolutionary algorithms,Adaptive genetic programming,Machine learning,Home automation,Energy efficiency
AI 理解论文
溯源树
样例
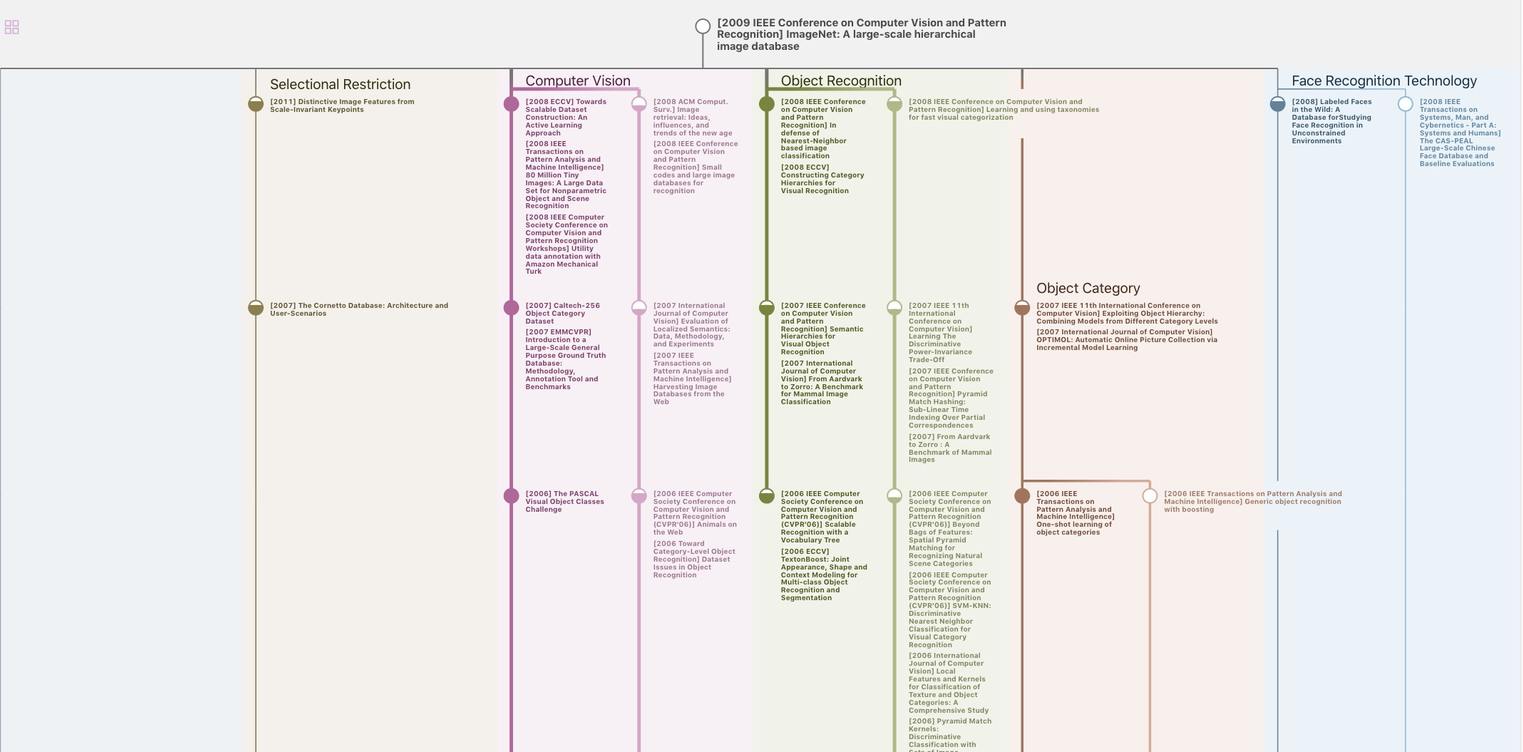
生成溯源树,研究论文发展脉络
Chat Paper
正在生成论文摘要