Differentially-Private Mining of Representative Travel Patterns
2016 17th IEEE International Conference on Mobile Data Management (MDM)(2016)
摘要
Mobile users participate in numerous social media applications that revolve around user locations, and receive customized services and recommendations tailored to their whereabouts. Large amounts of trajectory data become available as a byproduct of such services. Studying such data reveals travel patterns which can benefit transportation planning, public safety, etc. However, disclosing such data may lead to serious breaches of privacy. We propose a privacy-preserving approach to mining representative travel patterns using differential privacy (DP). Our solution consists of a sampling algorithm based on the exponential mechanism (EM) of DP which uses public road network information to increase sanitization accuracy. Extensive experimental results on realistic workloads show that the proposed protection technique preserves data precision and is computationally efficient.
更多查看译文
关键词
differentially-private mining,representative travel patterns,social media applications,user locations,customized services,trajectory data,privacy-preserving approach,representative travel pattern mining,exponential mechanism,sampling algorithm,EM,DP,public road network information,sanitization accuracy,protection technique,data precision,mobile users
AI 理解论文
溯源树
样例
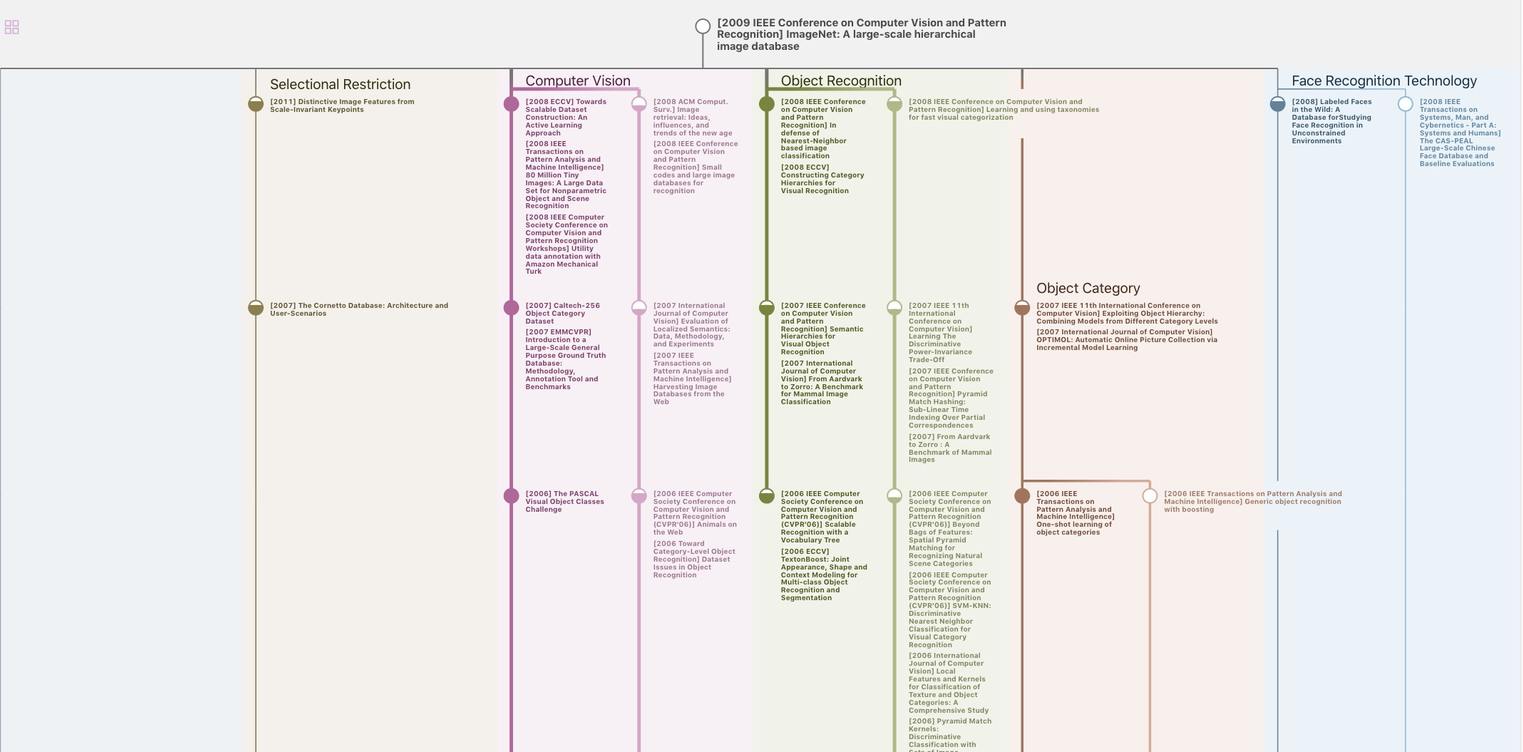
生成溯源树,研究论文发展脉络
Chat Paper
正在生成论文摘要