Measuring The Distance Between Finite Markov Decision Processes
AAMAS '16: Proceedings of the 2016 International Conference on Autonomous Agents & Multiagent Systems(2016)
摘要
Markov decision processes (MDPs) have been studied for many decades. Recent research in using transfer learning methods to solve MDPs has shown that knowledge learned from one MDP may be used to solve a similar MDP better. In this paper, we propose two metrics for measuring the distance between finite MDPs. Our metrics are based on the Hausdorff metric which measures the distance between two subsets of a metric space and the Kantorovich metric for measuring the distance between probabilistic distributions. Our metrics can be used to compute the distance between reinforcement learning tasks that are modeled as MDPs. The second contribution of this paper is that we apply the metrics to direct transfer learning by finding the similar source tasks. Our third contribution is that we propose two knowledge transfer methods which transfer value functions of the selected source tasks to the target task. Extensive experimental results show that our metrics are effective in finding similar tasks and significantly improve the performance of transfer learning with the transfer methods.
更多查看译文
关键词
Markov decision process,Kantorovich metric,Hausdorff metric,reinforcement learning,transfer learning
AI 理解论文
溯源树
样例
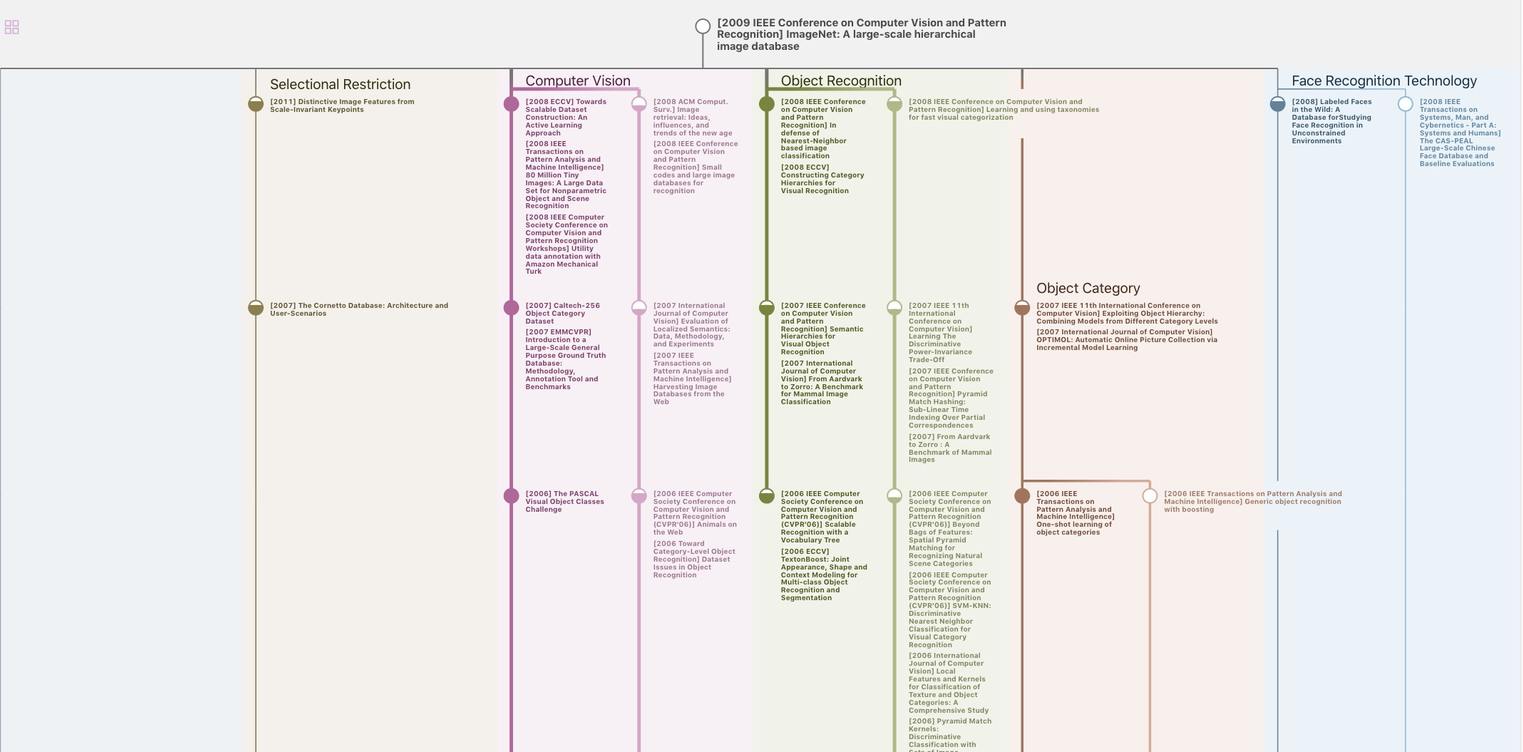
生成溯源树,研究论文发展脉络
Chat Paper
正在生成论文摘要