Ridesharing Recommendation: Whether And Where Should I Wait?
WEB-AGE INFORMATION MANAGEMENT, PT I(2016)
摘要
Ridesharing brings significant social and environmental benefits, e.g., saving energy consumption and satisfying people's commute demand. In this paper, we propose a recommendation framework to predict and recommend whether and where should the users wait to rideshare. In the framework, we utilize a large-scale GPS data set generated by over 7,000 taxis in a period of one month in Nanjing, China to model the arrival patterns of occupied taxis from different sources. The underlying road network is first grouped into a number of road clusters. GPS data are categorized to different clusters according to where their sources are located. Then we use a kernel density estimation approach to personalize the arrival pattern of taxis departing from each cluster rather than a universal distribution for all clusters. Given a query, we compute the potential of ridesharing and where should the user wait by investigating the probabilities of possible destinations based on ridesharing requirements. Users are recommended to take a taxi directly if the potential to rideshare with others is not high enough. Experimental results show that the accuracy about whether ridesharing or not and the ridesharing successful ratio are respectively about 3 times and at most 40% better than the naive "stay-as-where-you-are" strategy. This shows that about 500 users can save 4-8 min with our recommendation. Given 9 RMB as the starting taxi fare and suppose users can save half of the total fare by ridesharing, users can save 10.828-44.062 RMB.
更多查看译文
关键词
Recommender System, Road Segment, Kernel Density Estimation, Successful Ratio, Taxi Driver
AI 理解论文
溯源树
样例
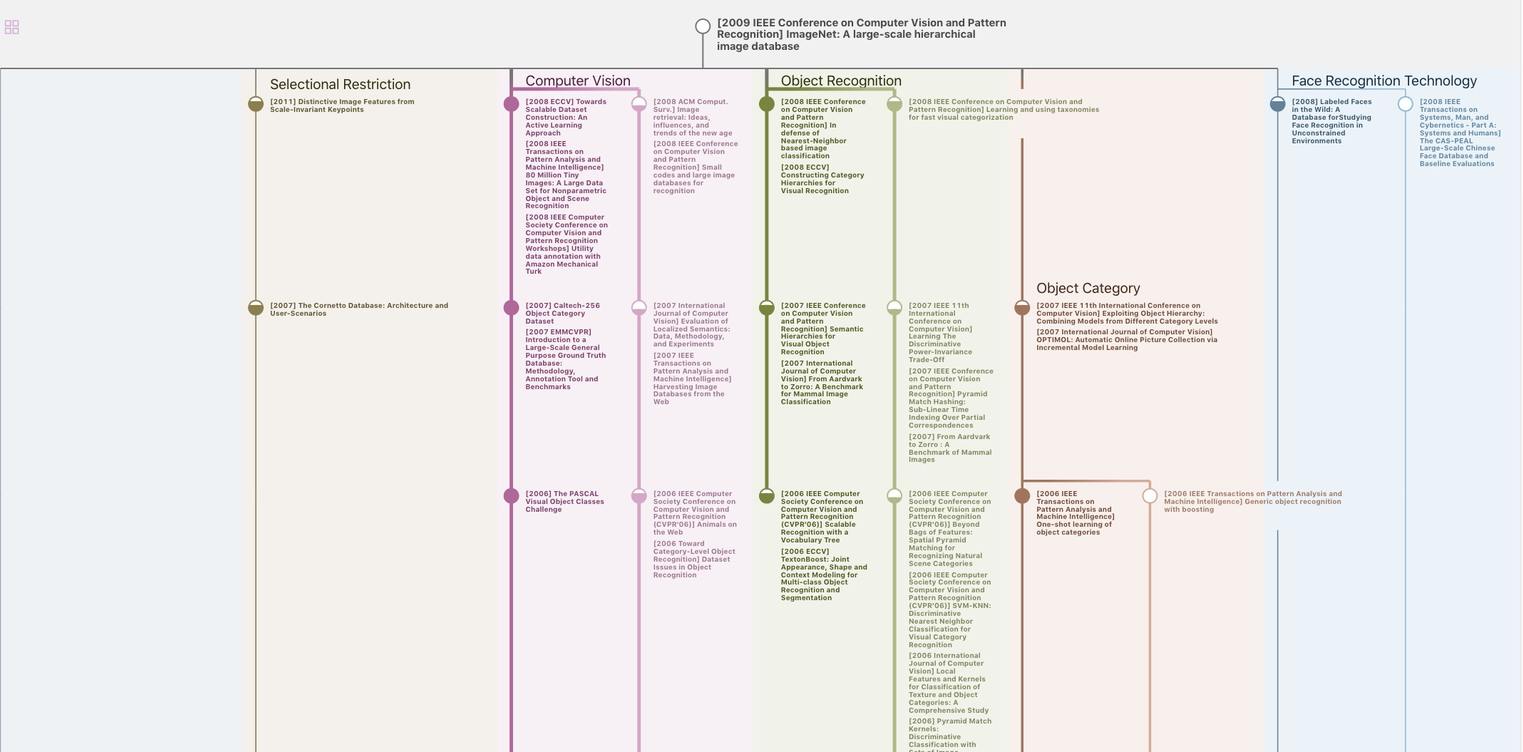
生成溯源树,研究论文发展脉络
Chat Paper
正在生成论文摘要