Data Classification Using Carbon-Nanotubes And Evolutionary Algorithms
PARALLEL PROBLEM SOLVING FROM NATURE - PPSN XIV(2016)
摘要
The potential of Evolution in Materio (EiM) for machine learning problems is explored here. This technique makes use of evolutionary algorithms (EAs) to influence the processing abilities of an un-configured physically rich medium, via exploitation of its physical properties. The EiM results reported are obtained using particle swarm optimisation (PSO) and differential evolution (DE) to exploit the complex voltage/current relationship of a mixture of single walled carbon nanotubes (SWCNTs) and liquid crystals (LCs). The computational problem considered is simple binary data classification. Results presented are consistent and reproducible. The evolutionary process based on EAs has the capacity to evolve the material to a state where data classification can be performed. Finally, it appears that through the use of smooth signal inputs, PSO produces classifiers out of the SWCNT/LC substrate which generalise better than those evolved with DE.
更多查看译文
关键词
Particle Swarm Optimisation,Differential Evolution,Particle Swarm Optimisation Algorithm,Single Walled Carbon Nanotubes,Computation Input
AI 理解论文
溯源树
样例
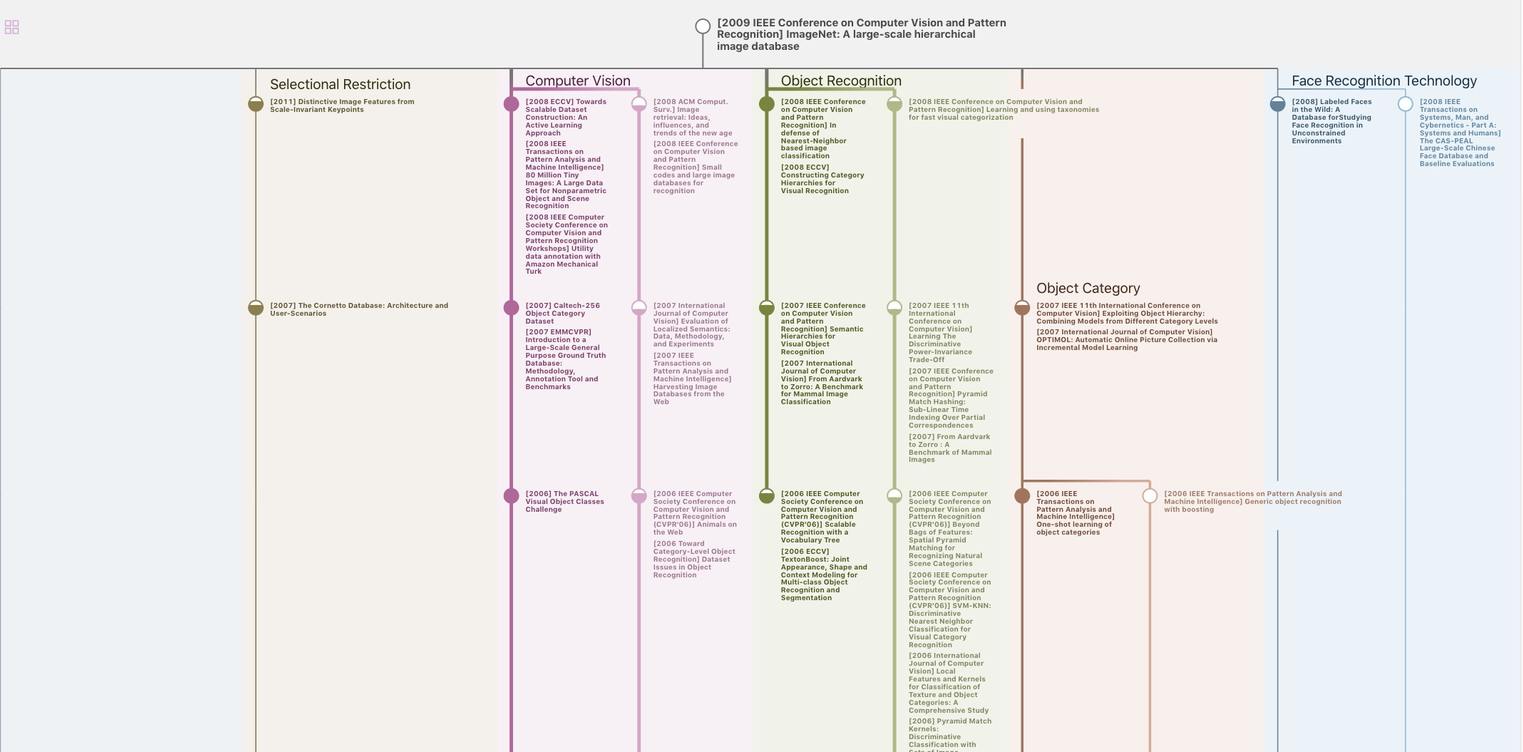
生成溯源树,研究论文发展脉络
Chat Paper
正在生成论文摘要