Discovering Relations to Augment a Web-scale Knowledge Base Constructed from the Web
WIMS(2016)
摘要
We propose a method for automatically discovering new knowledge from a knowledge base consisting of entity-relation-entity triples, constructed automatically from the Web. This method infers new relations between entity pairs by exploiting the structure of massive graphs, which can be obtained by linking existing entity-relation-entity triples. After identifying an unlinked entity pair likely to have a strong association from the graphs and a connected component around it, the method learns a new relation name from a similar structure. More precisely, it retrieves other connected components containing a similar structure and finds a new relation between the entities. The process can be considered a simple form of analogical reasoning, followed by identifying an additional relation in the searched connected component in the knowledge base. We used two datasets to evaluate the performance of this method. The ReVerb dataset, a large-scale knowledge base constructed from an Open Information Extraction method, was used to demonstrate its scalability and accuracy in comparison with SHERLOCK and other baselines. Our evaluation with the YAGO dataset showed the proposed method is applicable to other types of knowledge bases that are not from the Open Information Extraction paradigm.
更多查看译文
AI 理解论文
溯源树
样例
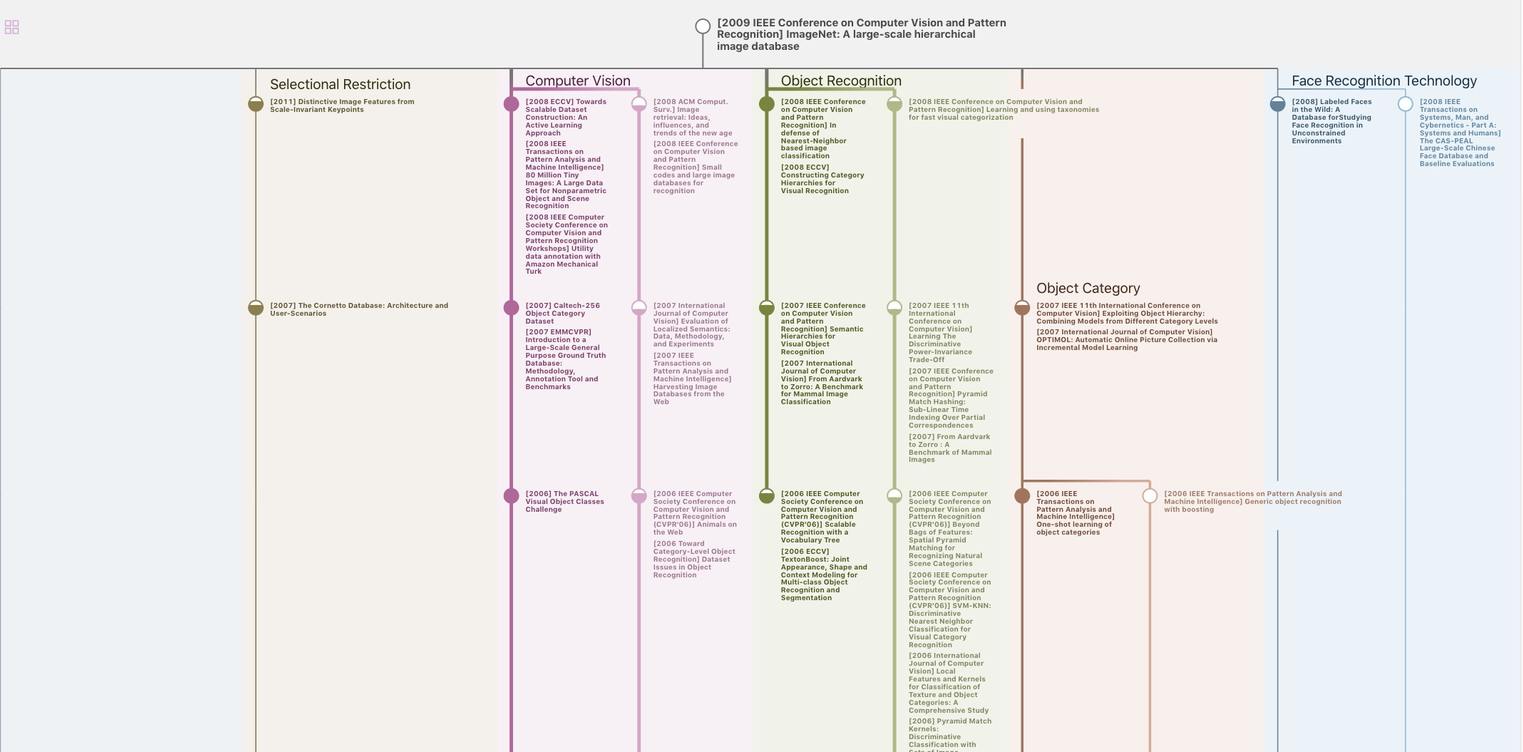
生成溯源树,研究论文发展脉络
Chat Paper
正在生成论文摘要