Heteroscedastic Sequences: Beyond Gaussianity.
ICML'16: Proceedings of the 33rd International Conference on International Conference on Machine Learning - Volume 48(2016)
摘要
We address the problem of sequential prediction in the heteroscedastic setting, when both the signal and its variance are assumed to depend on explanatory variables. By applying regret minimization techniques, we devise an efficient online learning algorithm for the problem, without assuming that the error terms comply with a specific distribution. We show that our algorithm can be adjusted to provide confidence bounds for its predictions, and provide an application to ARCH models. The theoretical results are corroborated by an empirical study.
更多查看译文
AI 理解论文
溯源树
样例
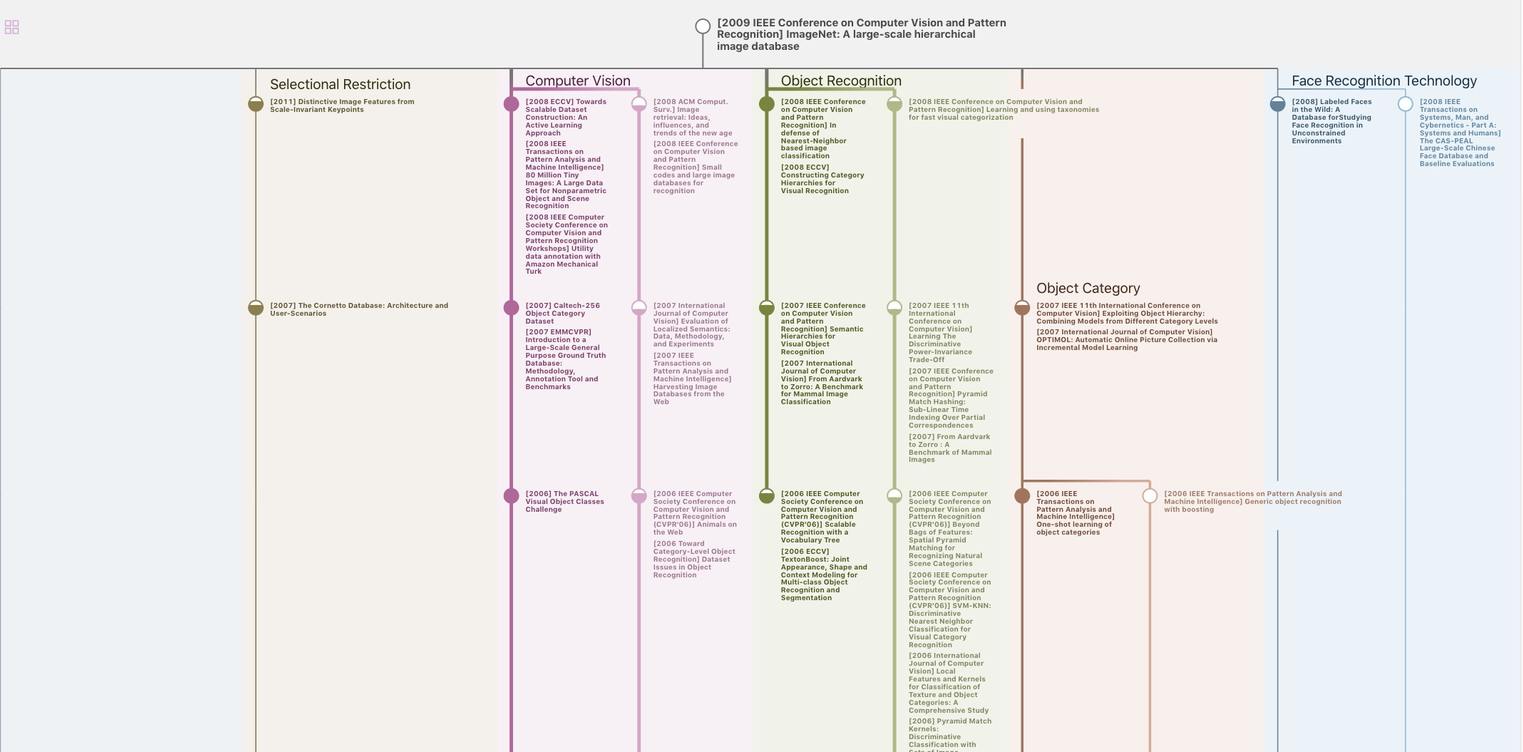
生成溯源树,研究论文发展脉络
Chat Paper
正在生成论文摘要