Improving Language Estimation With The Paragraph Vector Model For Ad-Hoc Retrieval
SIGIR '16: The 39th International ACM SIGIR conference on research and development in Information Retrieval Pisa Italy July, 2016(2016)
摘要
Incorporating topic level estimation into language models has been shown to be beneficial for information retrieval (IR) models such as cluster-based retrieval and LDA-based document representation. Neural embedding models, such as paragraph vector (PV) models, on the other hand have shown their effectiveness and efficiency in learning semantic representations of documents and words in multiple Natural Language Processing (NLP) tasks. However, their effectiveness in information retrieval is mostly unknown. In this paper, we study how to effectively use the PV model to improve ad-hoc retrieval. We propose three major improvements over the original PV model to adapt it for the IR scenario: (1) we use a document frequency-based rather than the corpus frequency-based negative sampling strategy so that the importance of frequent words will not be suppressed excessively; (2) we introduce regularization over the document representation to prevent the model overfitting short documents along with the learning iterations; and (3) we employ a joint learning objective which considers both the document-word and word-context associations to produce better word probability estimation. By incorporating this enhanced PV model into the language modeling framework, we show that it can significantly outperform the state-of-the-art topic enhanced language models.
更多查看译文
关键词
Retrieval Model,Language Model,Paragraph Vector
AI 理解论文
溯源树
样例
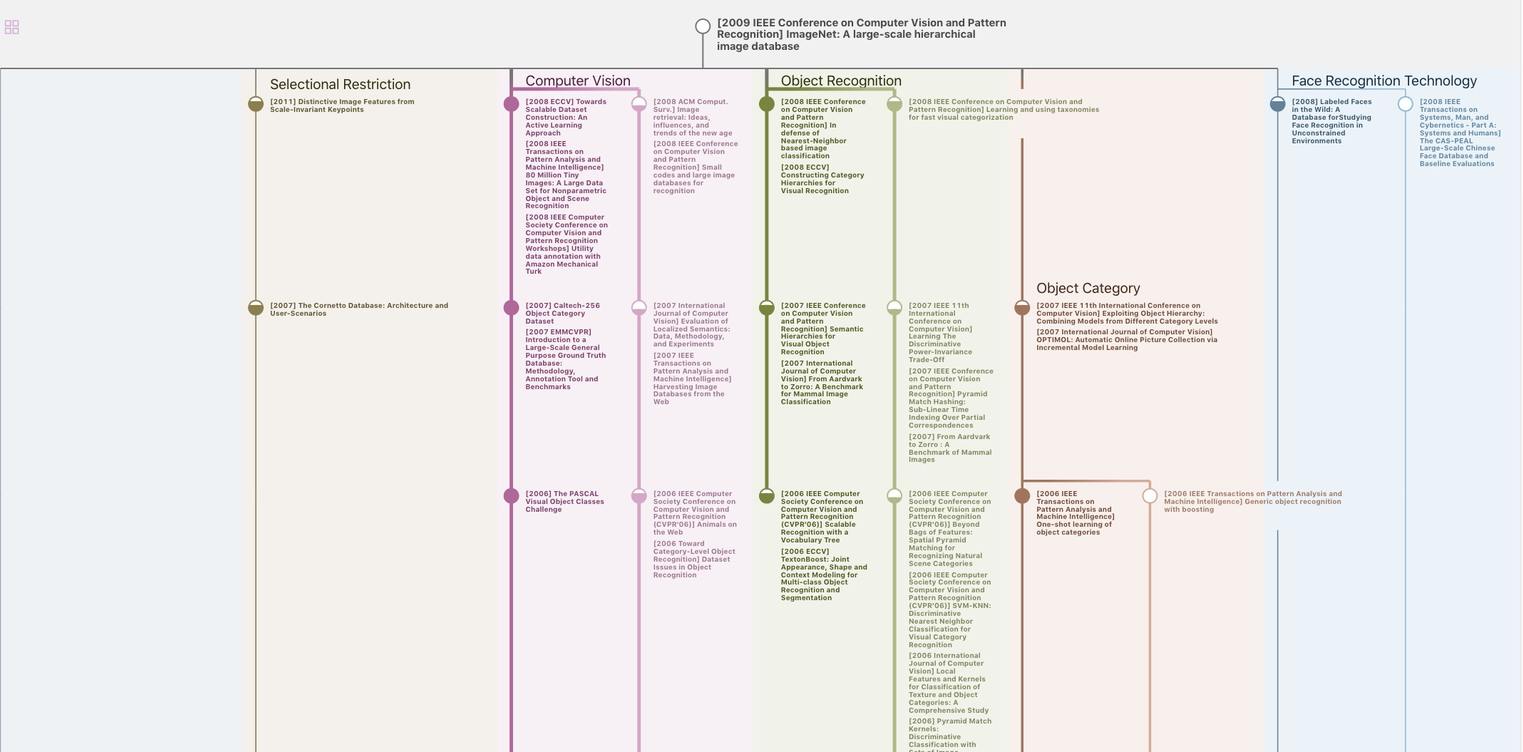
生成溯源树,研究论文发展脉络
Chat Paper
正在生成论文摘要