Helmholtz principle based supervised and unsupervised feature selection methods for text mining.
Inf. Process. Manage.(2016)
摘要
We propose new supervised and unsupervised feature selection methods, called Meaning Based Feature Selection (MBFS), for feature selection in text classification.We adapt and use the meaning measure as a new method for feature selection.Meaning measure is based on the Helmholtz principle from the Gestalt theory of human perception.MBFS methods are compared with nine different and well-known feature selection methods on six different datasets.Experimental results show that MBFS methods are effective feature selection methods and have higher speed than several widely used feature selection methods. One of the important problems in text classification is the high dimensionality of the feature space. Feature selection methods are used to reduce the dimensionality of the feature space by selecting the most valuable features for classification. Apart from reducing the dimensionality, feature selection methods have potential to improve text classifiers' performance both in terms of accuracy and time. Furthermore, it helps to build simpler and as a result more comprehensible models. In this study we propose new methods for feature selection from textual data, called Meaning Based Feature Selection (MBFS) which is based on the Helmholtz principle from the Gestalt theory of human perception which is used in image processing. The proposed approaches are extensively evaluated by their effect on the classification performance of two well-known classifiers on several datasets and compared with several feature selection algorithms commonly used in text mining. Our results demonstrate the value of the MBFS methods in terms of classification accuracy and execution time.
更多查看译文
关键词
Feature selection,Attribute selection,Machine learning,Text mining,Text classification,Helmholtz principle
AI 理解论文
溯源树
样例
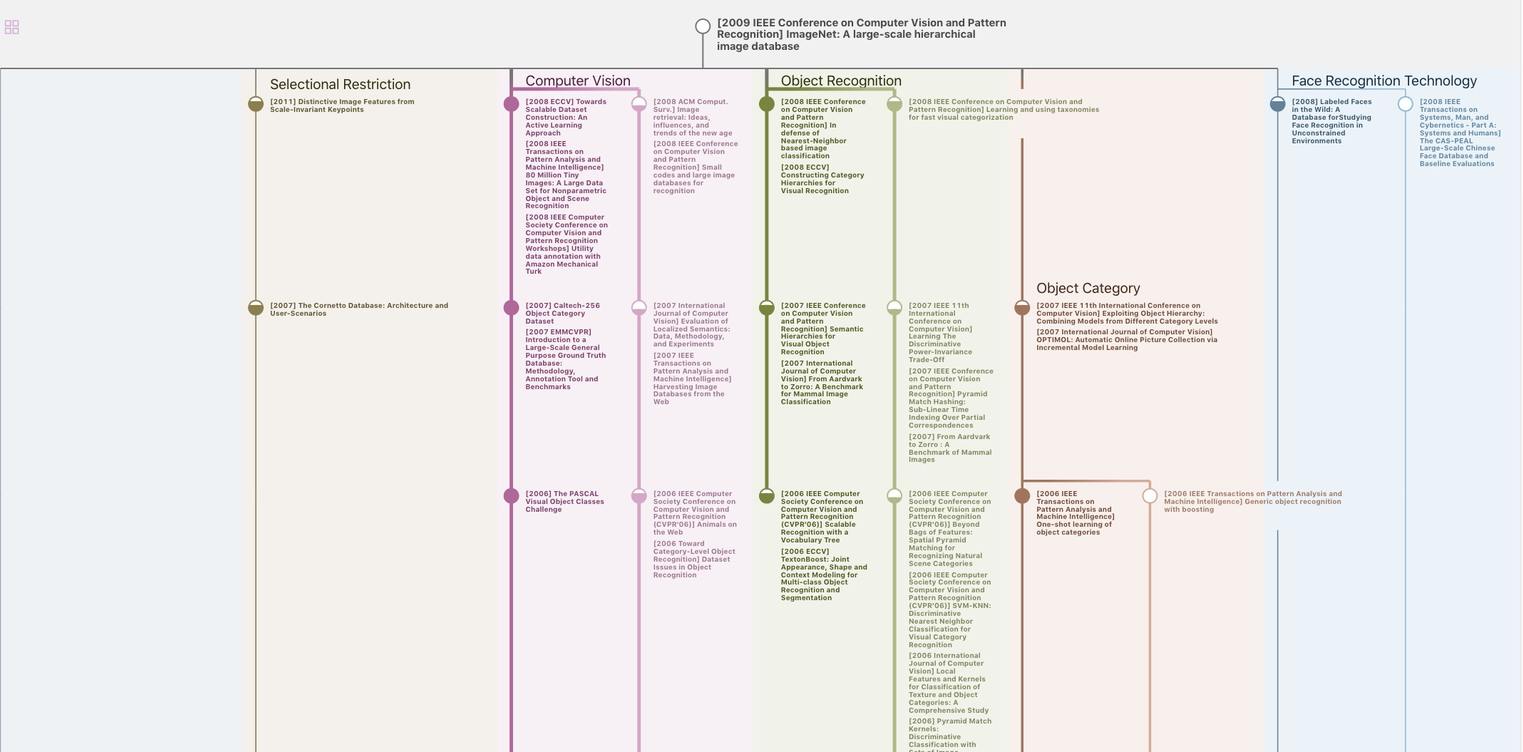
生成溯源树,研究论文发展脉络
Chat Paper
正在生成论文摘要