The Stability And Usability Of Statistical Topic Models
ACM Transactions on Interactive Intelligent Systems(2016)
摘要
Statistical topic models have become a useful and ubiquitous tool for analyzing large text corpora. One common application of statistical topic models is to support topic-centric navigation and exploration of document collections. Existing work on topic modeling focuses on the inference of model parameters so the resulting model fits the input data. Since the exact inference is intractable, statistical inference methods, such as Gibbs Sampling, are commonly used to solve the problem. However, most of the existing work ignores an important aspect that is closely related to the end user experience: topic model stability. When the model is either re-trained with the same input data or updated with new documents, the topic previously assigned to a document may change under the new model, which may result in a disruption of end users' mental maps about the relations between documents and topics, thus undermining the usability of the applications. In this article, we propose a novel user-directed non-disruptive topic model update method that balances the tradeoff between finding the model that fits the data and maintaining the stability of the model from end users' perspective. It employs a novel constrained LDA algorithm to incorporate pairwise document constraints, which are converted from user feedback about topics, to achieve topic model stability. Evaluation results demonstrate the advantages of our approach over previous methods.
更多查看译文
关键词
Statistical topic model,non-disruptive topic model update,LDA,usability,stability,constrained topic model,text analytics
AI 理解论文
溯源树
样例
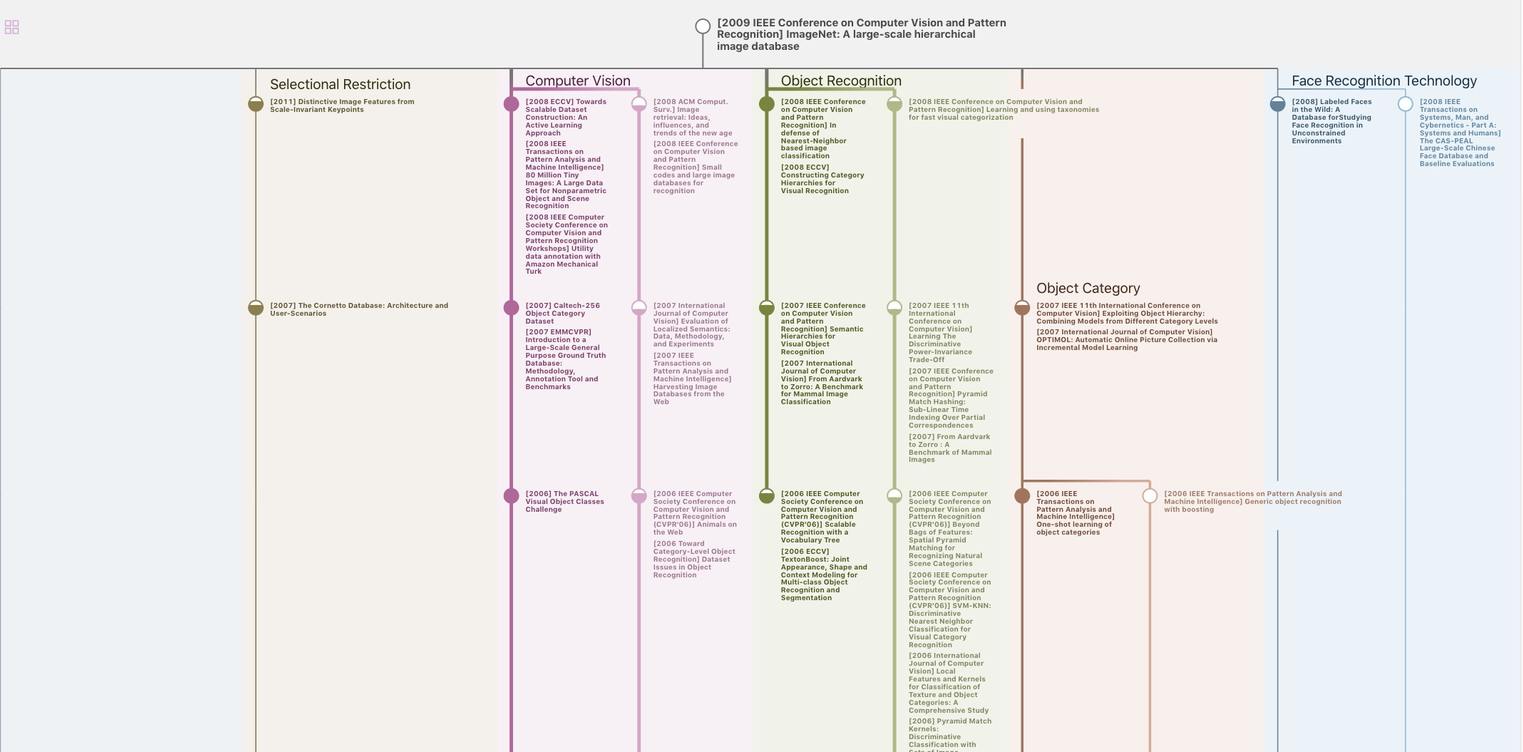
生成溯源树,研究论文发展脉络
Chat Paper
正在生成论文摘要