Crime Rate Inference with Big Data
KDD(2016)
摘要
Crime is one of the most important social problems in the country, affecting public safety, children development, and adult socioeconomic status. Understanding what factors cause higher crime is critical for policy makers in their efforts to reduce crime and increase citizens' life quality. We tackle a fundamental problem in our paper: crime rate inference at the neighborhood level. Traditional approaches have used demographics and geographical influences to estimate crime rates in a region. With the fast development of positioning technology and prevalence of mobile devices, a large amount of modern urban data have been collected and such big data can provide new perspectives for understanding crime. In this paper, we used large-scale Point-Of-Interest data and taxi flow data in the city of Chicago, IL in the USA. We observed significantly improved performance in crime rate inference compared to using traditional features. Such an improvement is consistent over multiple years. We also show that these new features are significant in the feature importance analysis.
更多查看译文
AI 理解论文
溯源树
样例
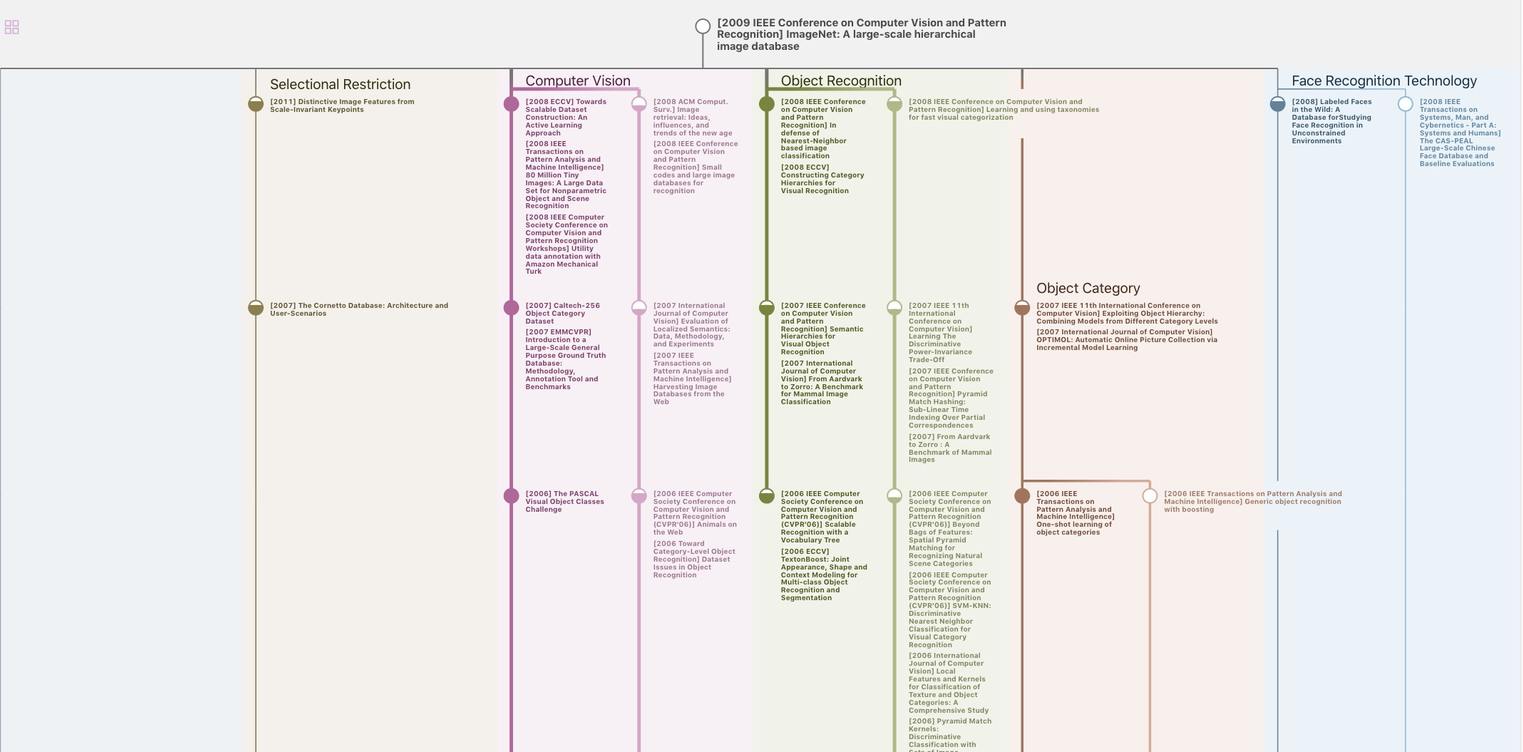
生成溯源树,研究论文发展脉络
Chat Paper
正在生成论文摘要