An Empirical Study on Recommendation with Multiple Types of Feedback
KDD(2016)
摘要
User feedback like clicks and ratings on recommended items provides important information for recommender systems to predict users' interests in unseen items. Most systems rely on models trained using a single type of feedback, e.g., ratings for movie recommendation and clicks for online news recommendation. However, in addition to the primary feedback, many systems also allow users to provide other types of feedback, e.g., liking or sharing an article, or hiding all articles from a source. These additional feedback potentially provides extra information for the recommendation models. To optimize user experience and business objectives, it is important for a recommender system to use both the primary feedback and additional feedback. This paper presents an empirical study on various training methods for incorporating multiple user feedback types based on LinkedIn recommendation products. We study three important problems that we face at LinkedIn: (1) Whether to send an email based on clicks and complaints, (2) how to rank updates in LinkedIn feeds based on clicks and hides and (3) how jointly optimize for viral actions and clicks in LinkedIn feeds. Extensive offline experiments on historical data show the effectiveness of these methods in different situations. Online A/B testing results further demonstrate the impact of these methods on LinkedIn production systems.
更多查看译文
关键词
Recommender System,Personalized Recommendation,Multi-objective Optimization
AI 理解论文
溯源树
样例
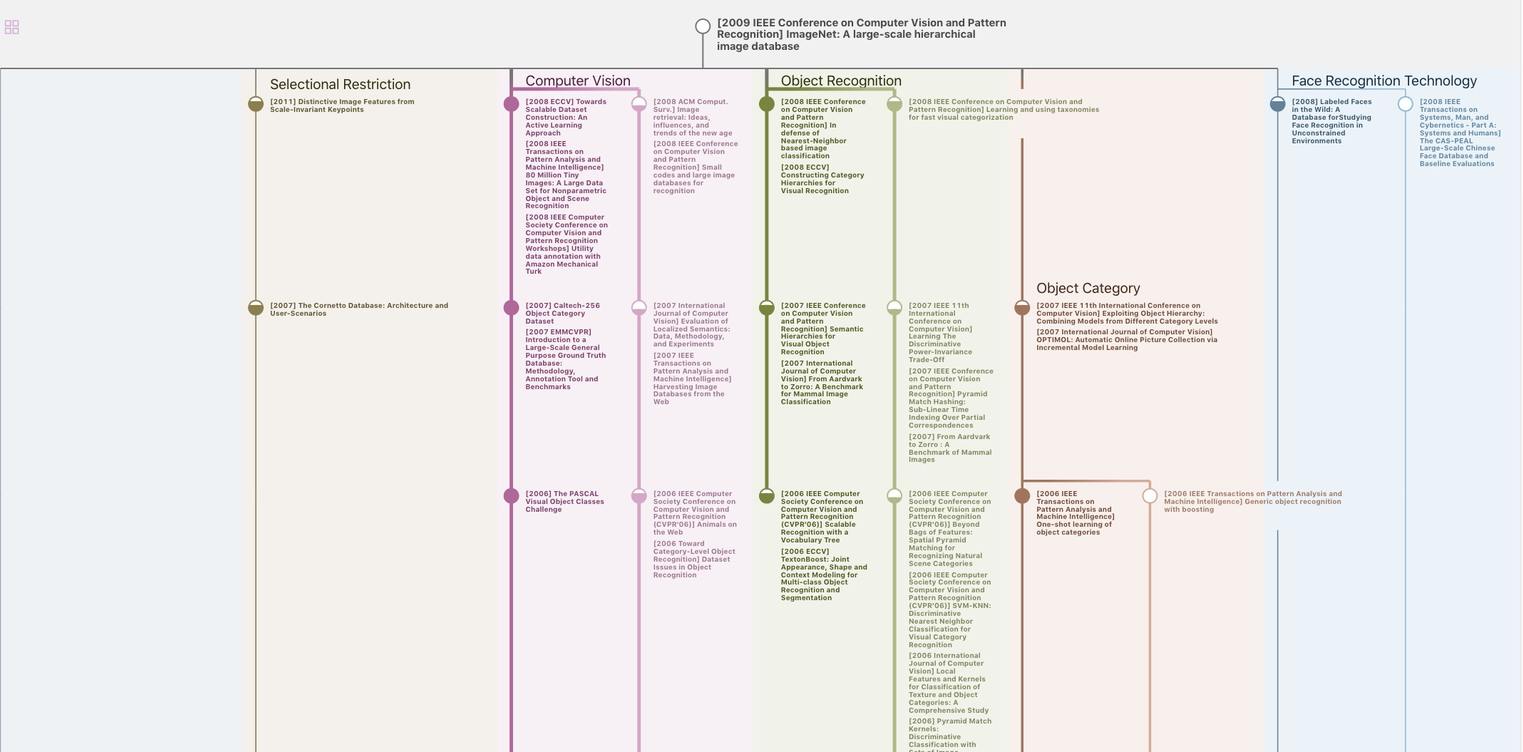
生成溯源树,研究论文发展脉络
Chat Paper
正在生成论文摘要