Guided Learning of Control Graphs for Physics-Based Characters.
ACM Trans. Graph.(2016)
摘要
The difficulty of developing control strategies has been a primary bottleneck in the adoption of physics-based simulations of human motion. We present a method for learning robust feedback strategies around given motion capture clips as well as the transition paths between clips. The output is a control graph that supports real-time physics-based simulation of multiple characters, each capable of a diverse range of robust movement skills, such as walking, running, sharp turns, cartwheels, spin-kicks, and flips. The control fragments that compose the control graph are developed using guided learning. This leverages the results of open-loop sampling-based reconstruction in order to produce state-action pairs that are then transformed into a linear feedback policy for each control fragment using linear regression. Our synthesis framework allows for the development of robust controllers with a minimal amount of prior knowledge.
更多查看译文
关键词
Character Animation,Motion control,human simulation,control graphs,guided policy search
AI 理解论文
溯源树
样例
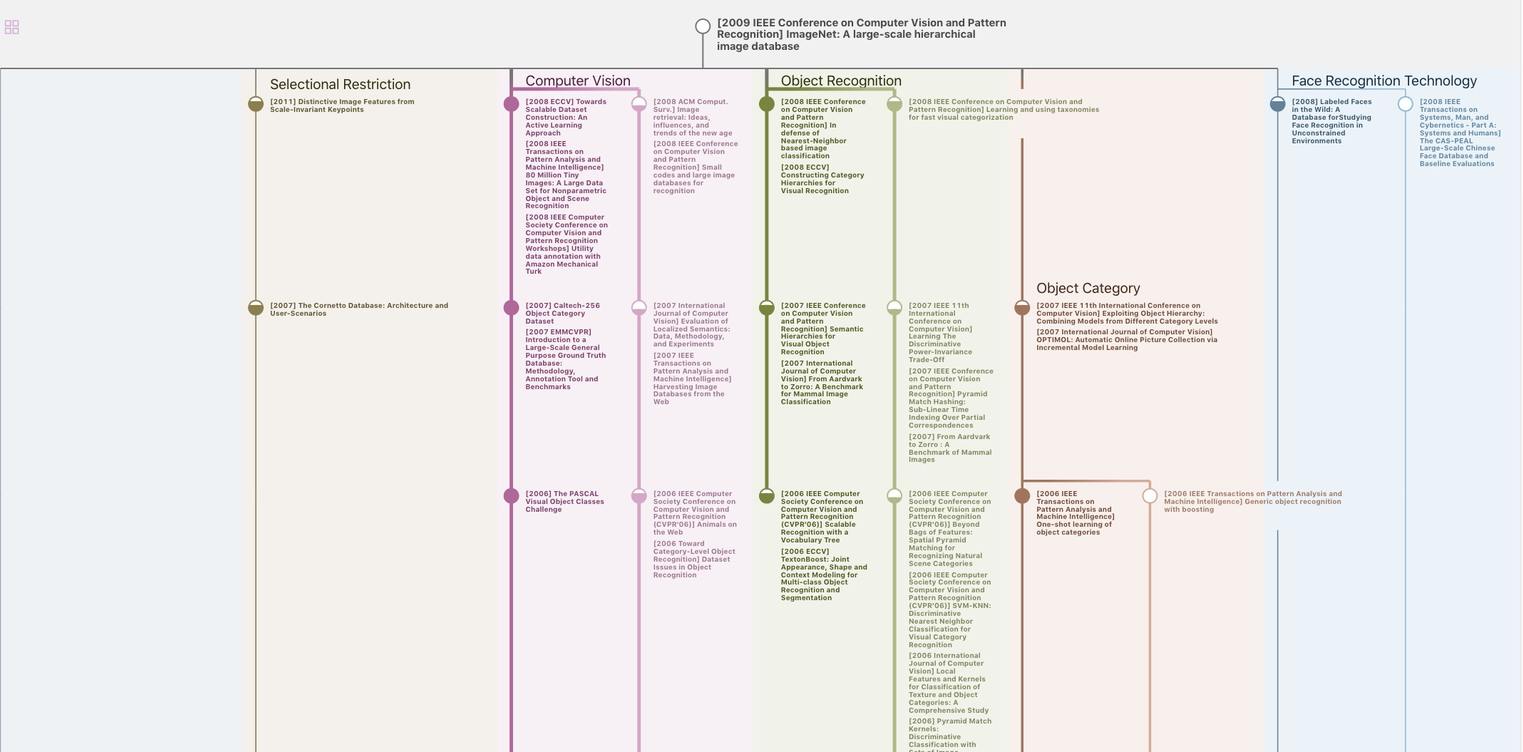
生成溯源树,研究论文发展脉络
Chat Paper
正在生成论文摘要