A novel multistart hyper-heuristic algorithm on the grid for the quadratic assignment problem.
Eng. Appl. of AI(2016)
摘要
Hyper-heuristics introduce novel approaches for solving challenging combinatorial optimization problems by operating over a set of low level (meta)-heuristics. This is achieved by an evolutionary selection mechanism that controls and combines the strengths of the low level (meta)-heuristics. In this study, we propose a high-performance MultiStart Hyper-heuristic algorithm (MSH-QAP) on the grid for the solution of the Quadratic Assignment Problem (QAP). MSH-QAP algorithm makes use of state-of-the-art (meta)-heuristics, Simulated Annealing (SA), Robust Tabu Search (RTS), Ant Colony Optimization (FAnt), and Breakout Local Search (BLS) that have been reported among the best performing algorithms for the solution of difficult QAP instances in standard benchmark libraries. In the first phase of the algorithm, the most appropriate (meta)-heuristic with its near-optimal parameter settings is selected by using a genetic algorithm optimization layer that uses a self-adaptive parameter setting method for the given problem instance. In the second phase, if an optimal solution cannot be found, selected best performing (meta)-heuristic (with its finely adjusted parameter settings) is executed on the grid using parallel processing and performing several multistarts in order to increase the quality of the discovered solution. MSH-QAP algorithm is tested on 134 problem instances of the QAPLIB benchmark and is shown to be able to solve 122 of the instances exactly. The overall deviation for the problem instances is obtained as 0.013% on the average.
更多查看译文
关键词
Hyper-heuristics,Assignment,Simulated annealing,Tabu search,Ant colony,Breakout local search
AI 理解论文
溯源树
样例
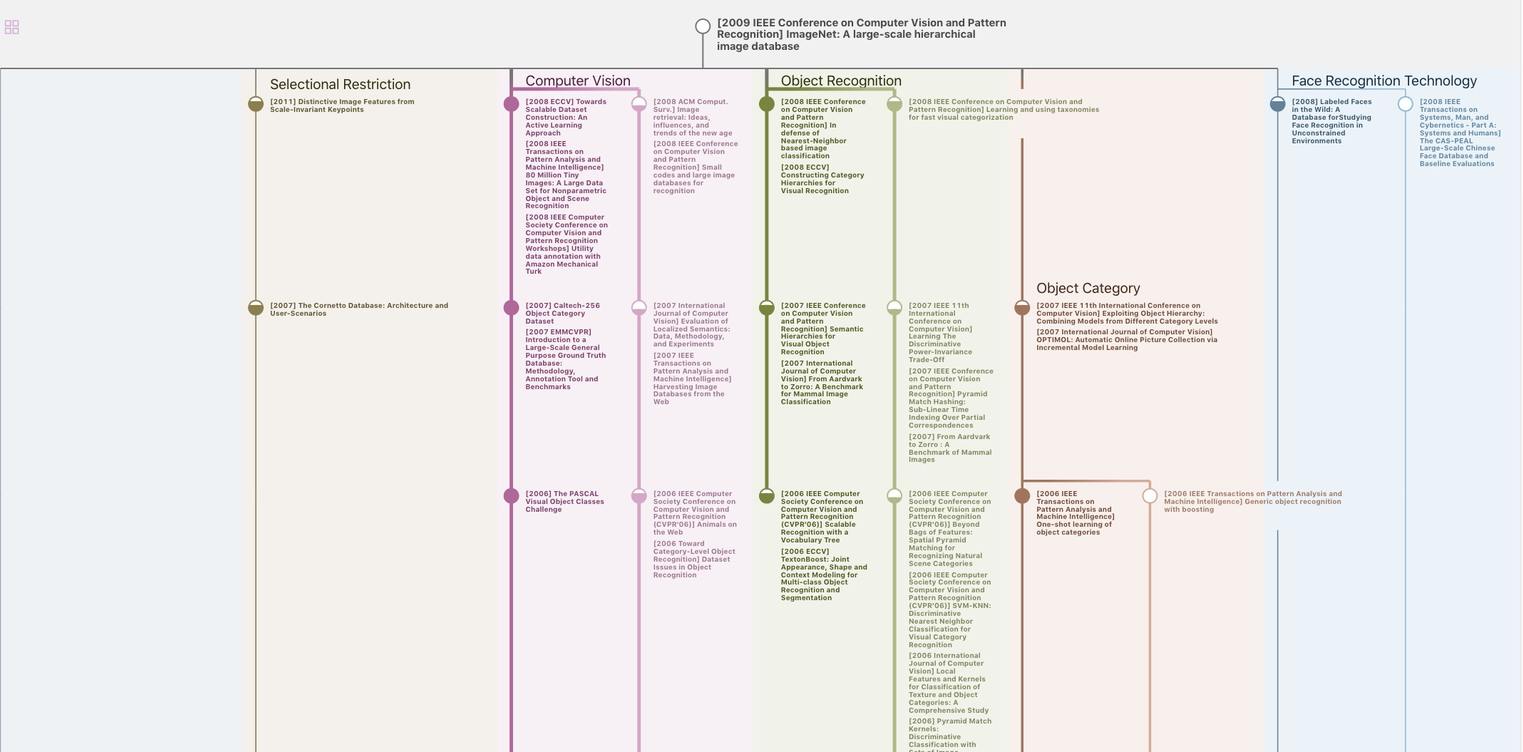
生成溯源树,研究论文发展脉络
Chat Paper
正在生成论文摘要