Nonlinear system identification based on a self-organizing type-2 fuzzy RBFN.
Eng. Appl. of AI(2016)
摘要
This paper presents a new self-evolving recurrent Type-2 Fuzzy Radial Basis Function Network (T2FRBFN) in which the weights are considered Gaussian type-2 fuzzy sets and uncertain mean in each RBF neuron. The capability of the proposed T2FRBFN for function approximation and dynamical system identification perform better than the conventional RBFN. A novel type-2 fuzzy clustering is presented to add or remove the hidden RBF neurons. For parameter learning, back-propagation with adaptive learning rate is used. Finally the proposed T2FRBFN is applied to identification of three nonlinear systems as case studies. A comparison between T2FRBFN and the conventional RBFN as well as the method ofźRubio-Solis and Panoutsos (2015) is presented. Simulation results and their statistical description show that the proposed T2FRBFN perform better than the conventional RBFN.
更多查看译文
关键词
Recurrent RBFN,Type-2 Fuzzy sets,Self-evolving,System identification
AI 理解论文
溯源树
样例
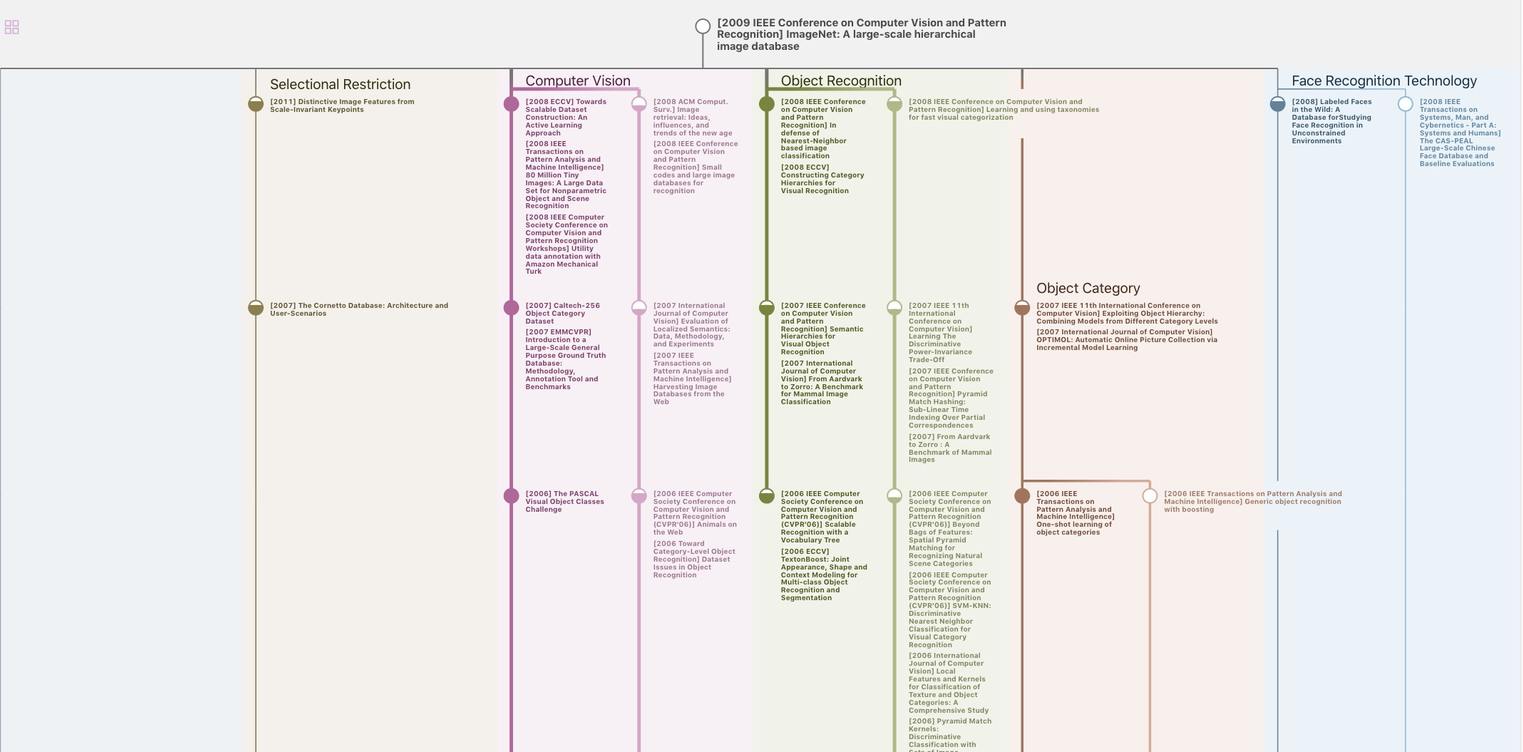
生成溯源树,研究论文发展脉络
Chat Paper
正在生成论文摘要