Unsupervised labelling of sequential data for location identification in indoor environments.
Expert Syst. Appl.(2016)
摘要
Presents indoor positioning as an unsupervised labelling task on sequential data.Forms a spatial classifier without resorting to pre-determined maps.Differentiates location between unknown closely spaced zones indoors.Presents a valuable working framework for real-world positioning problems.Extends literature studying applications of graphical models. In this paper we present indoor positioning within unknown environments as an unsupervised labelling task on sequential data. We explore a probabilistic framework relying on wireless network radio signals and contextual information, which is increasingly available in large environments. Thus, we form an informative spatial classifier without resorting to a pre-determined map, and show the potential of the approach using both simulated and real data sets.Results demonstrate the ability of the procedure to segregate structures of radio signal observations and form clustered regions in association to areas of interest to the user; thus, we show it is possible to differentiate location between closely spaced zones of variable size and shape.
更多查看译文
关键词
Unsupervised labelling,Sequential data,Indoor positioning,Ubiquitous computing,Graphical models
AI 理解论文
溯源树
样例
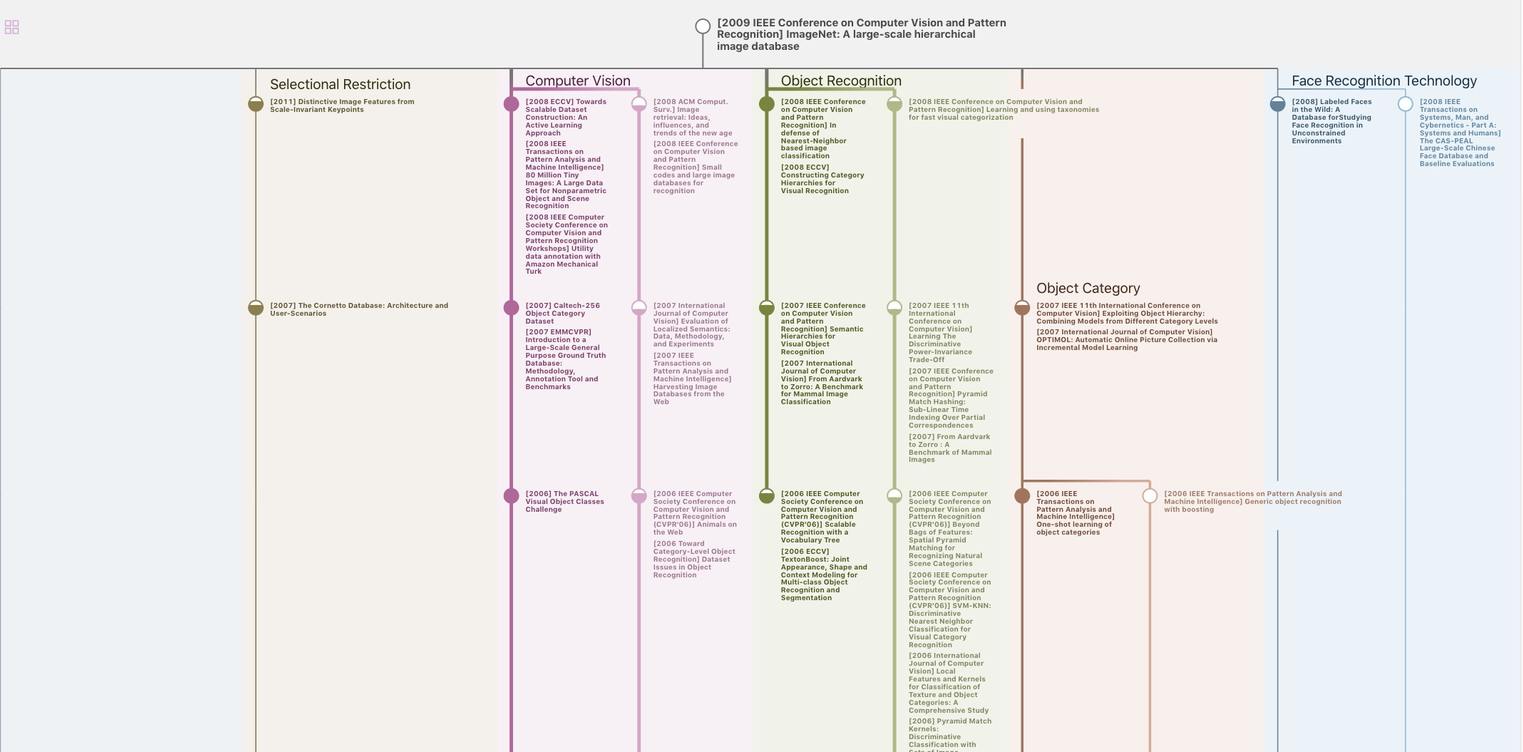
生成溯源树,研究论文发展脉络
Chat Paper
正在生成论文摘要