Natural Language Generation in Dialogue using Lexicalized and Delexicalized Data.
international conference on learning representations(2017)
摘要
Natural language generation plays a critical role in spoken dialogue systems. We present a new approach to natural language generation for task-oriented dialogue using recurrent neural networks in an encoder-decoder framework. In contrast to previous work, our model uses both lexicalized and delexicalized components i.e. slot-value pairs for dialogue acts, with slots and corresponding values aligned together. This allows our model to learn from all available data including the slot-value pairing, rather than being restricted to delexicalized slots. We show that this helps our model generate more natural sentences with better grammar. We further improve our modelu0027s performance by transferring weights learnt from a pretrained sentence auto-encoder. Human evaluation of our best-performing model indicates that it generates sentences which users find more appealing.
更多查看译文
AI 理解论文
溯源树
样例
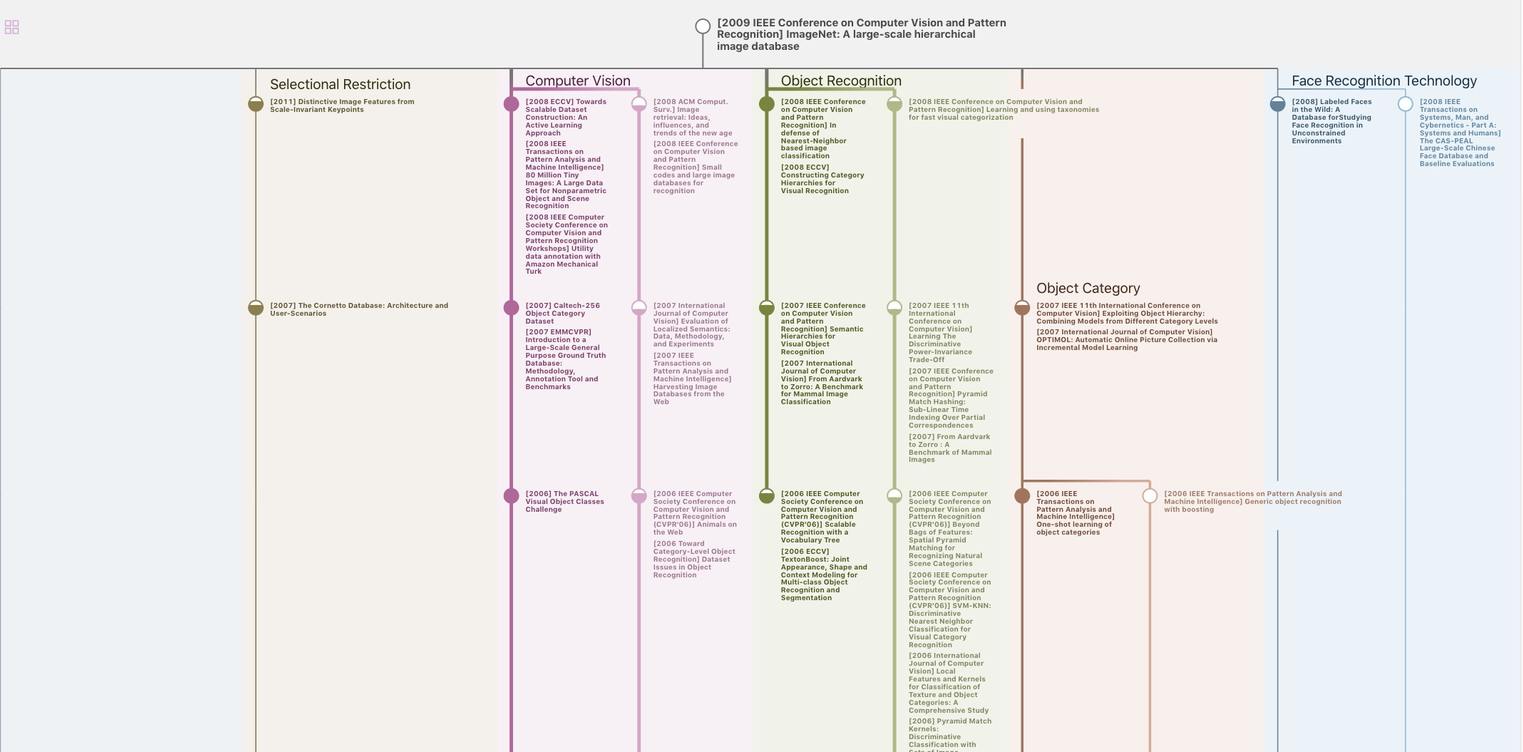
生成溯源树,研究论文发展脉络
Chat Paper
正在生成论文摘要