Modeling daily electrical demand in presence of PHEVs in smart grids with supervised learning
arXiv: Learning(2016)
摘要
Replacing a portion of current light-duty vehicles (LDVs) with plug-in hybrid electric vehicles (PHEVs) offers the possibility to reduce the dependence on fossil fuels together with environmental and economic benefits. However, charging a myriad of PHEVs will certainly introduce a huge new load to the power grid. In the framework of the development of a smarter grid, the primary focus of the present study is to propose a model for the daily electrical demand from the residential sector in presence of PHEVs. The expected demand from a PHEV is modeled by assigning certain probability distributions to the PHEV's required charging time and the starting time of charge. We assign a normal distribution for the starting time of charge which follows the real world practice. Furthermore, several distributions for the required charging time are considered: uniform distribution, Gaussian with positive support, Rician distribution and a non-uniform distribution coming from driving patterns in real-world data. We generate daily demand profiles by using real-world residential profiles throughout 2014 in the presence of different expected PHEV demand scenarios. Support vector machines (SVMs), a set of supervised machine learning models, are employed in order to find the best model to fit the data. SVMs with radial basis function (RBF) and polynomial kernels were tested. Model performances are evaluated by means of mean squared error (MSE) and mean absolute percentage error (MAPE). We show that the best results are obtained with RBF kernel: maximum (worst) values for MSE and MAPE are about 2.89 10
-8
and 0.023, respectively.
更多查看译文
关键词
Artificial intelligence (AI),energy demand,plug-in hybrid electric vehicle (PHEV),smart grids,support vector machines (SVMs)
AI 理解论文
溯源树
样例
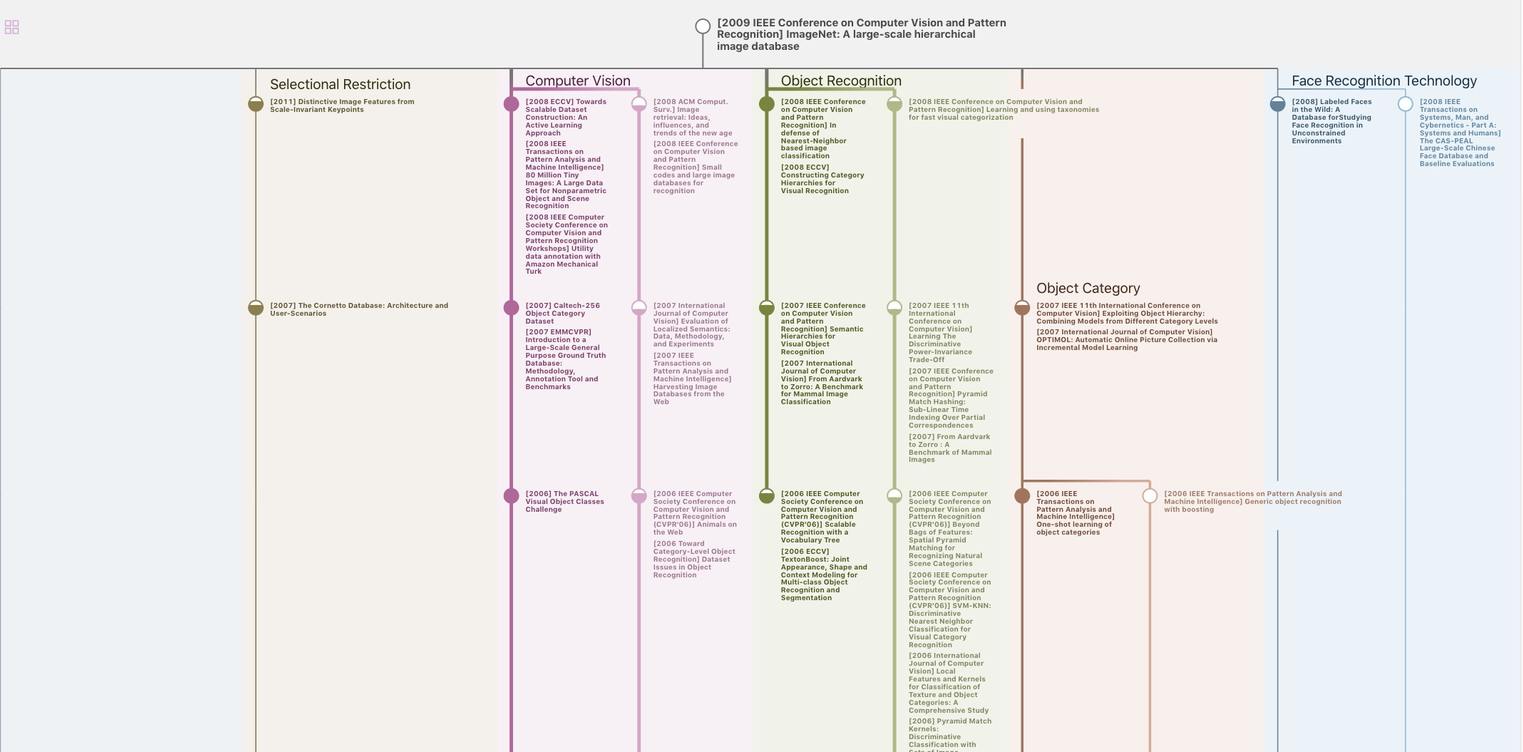
生成溯源树,研究论文发展脉络
Chat Paper
正在生成论文摘要