On Avoidance Learning with Partial Observability.
arXiv: Artificial Intelligence(2016)
摘要
We study a framework where agents have to avoid aversive signals. The agents are given only partial information, in the form of features that are projections of task states. Additionally, the agents have to cope with non-determinism, defined as unpredictability on the way that actions are executed. The goal of each agent is to define its behavior based on feature-action pairs that reliably avoid aversive signals. We study a learning algorithm, called A-learning, that exhibits fixpoint convergence, where the belief of the allowed feature-action pairs eventually becomes fixed. A-learning is parameter-free and easy to implement.
更多查看译文
AI 理解论文
溯源树
样例
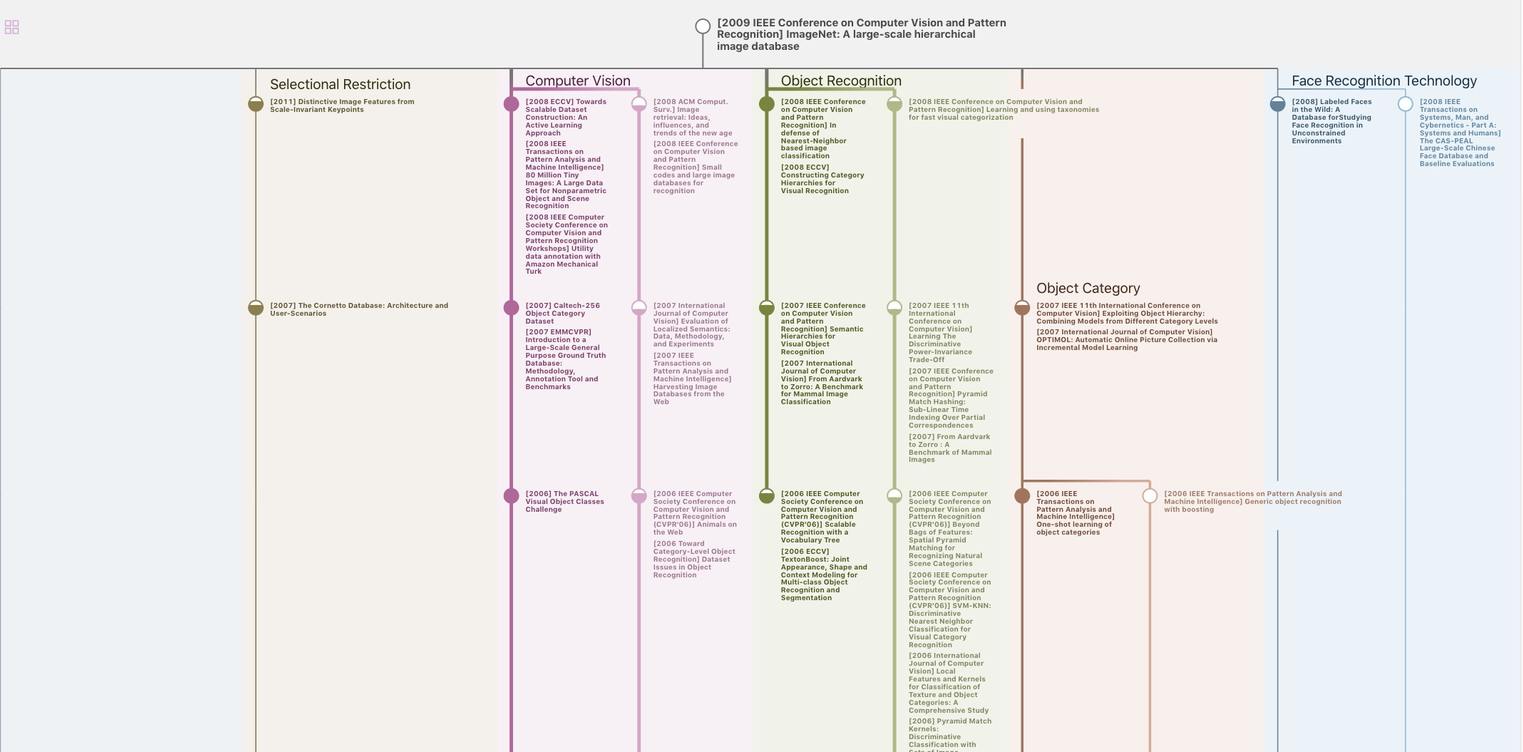
生成溯源树,研究论文发展脉络
Chat Paper
正在生成论文摘要