Latent Tree Models for Hierarchical Topic Detection.
Artificial Intelligence(2017)
摘要
We present a novel method for hierarchical topic detection where topics are obtained by clustering documents in multiple ways. Specifically, we model document collections using a class of graphical models called hierarchical latent tree models (HLTMs). The variables at the bottom level of an HLTM are observed binary variables that represent the presence/absence of words in a document. The variables at other levels are binary latent variables that represent word co-occurrence patterns or co-occurrences of such patterns. Each latent variable gives a soft partition of the documents, and document clusters in the partitions are interpreted as topics. Latent variables at high levels of the hierarchy capture long-range word co-occurrence patterns and hence give thematically more general topics, while those at low levels of the hierarchy capture short-range word co-occurrence patterns and give thematically more specific topics. In comparison with LDA-based methods, a key advantage of the new method is that it represents co-occurrence patterns explicitly using model structures. Extensive empirical results show that the new method significantly outperforms the LDA-based methods in term of model quality and meaningfulness of topics and topic hierarchies.
更多查看译文
关键词
Probabilistic graphical models,Text analysis,Hierarchical latent tree analysis,Hierarchical topic detection
AI 理解论文
溯源树
样例
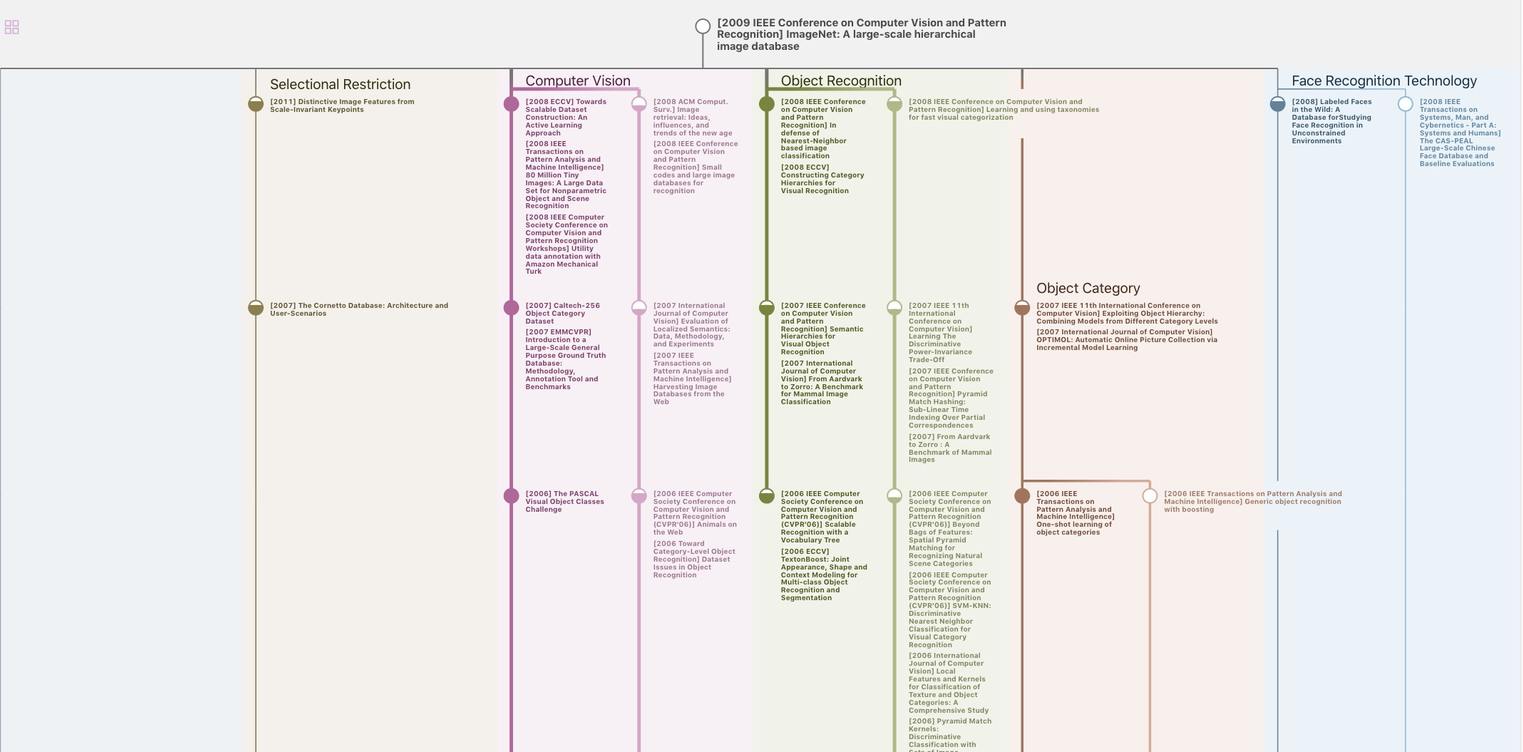
生成溯源树,研究论文发展脉络
Chat Paper
正在生成论文摘要