The Crossover Process: Learnability meets Protection from Inference Attacks.
arXiv: Learning(2016)
摘要
It is usual to consider data protection and learnability as conflicting objectives. This is not always the case: we show how to jointly control causal inference --- seen as the attack --- textit{and} learnability by a noise-free process that mixes training examples, the Crossover Process (cp). One key point is that the cp~is typically able to alter joint distributions without touching on marginals, nor altering the sufficient statistic for the class. In other words, it saves (and sometimes improves) generalization for supervised learning, but can alter the relationship between covariates --- and therefore fool statistical measures of (nonlinear) independence and causal inference into misleading ad-hoc conclusions. Experiments on a dozen readily available domains validate the theory.
更多查看译文
AI 理解论文
溯源树
样例
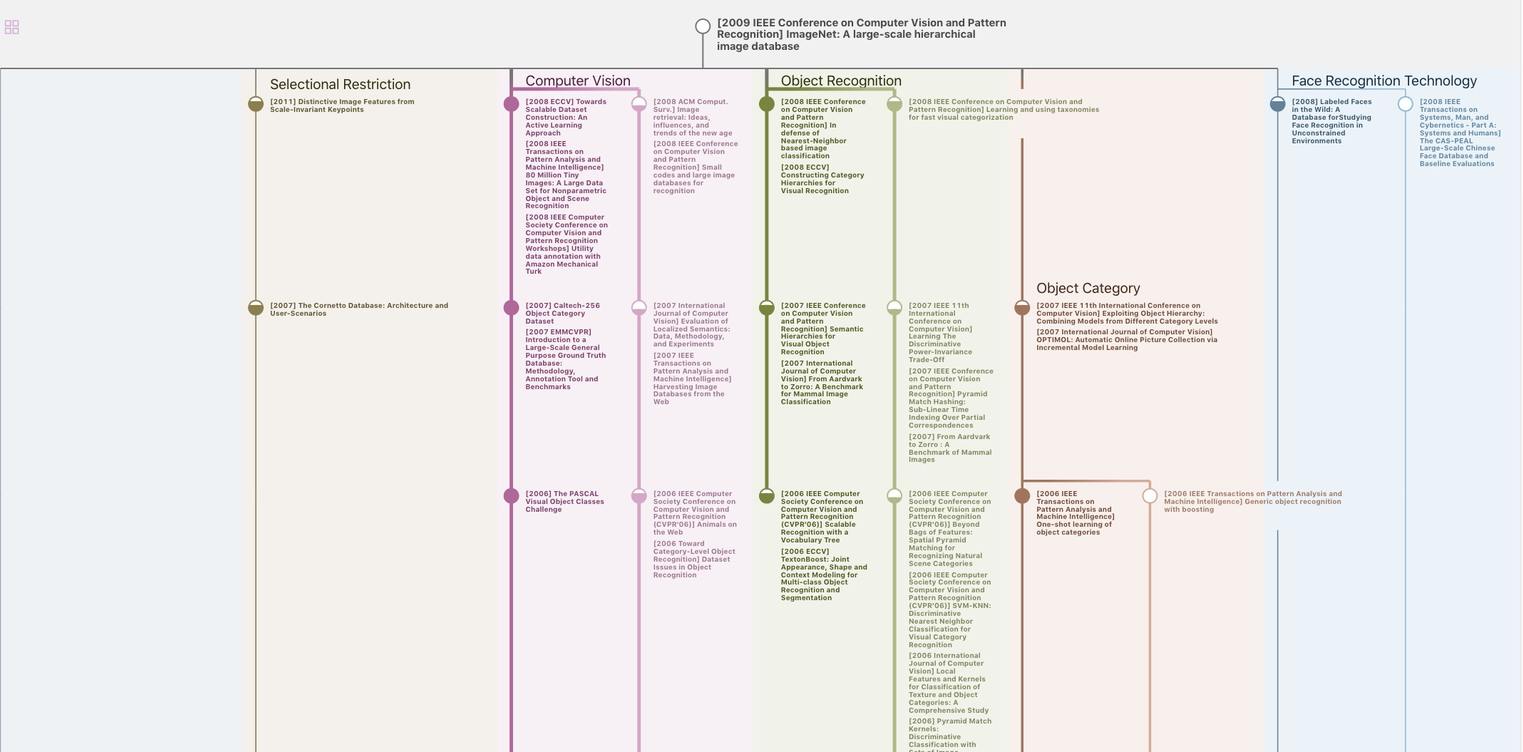
生成溯源树,研究论文发展脉络
Chat Paper
正在生成论文摘要