Real-Time Anomaly Detection for Streaming Analytics.
arXiv: Artificial Intelligence(2016)
摘要
Much of the worlds data is streaming, time-series data, where anomalies give significant information in critical situations. Yet detecting anomalies in streaming data is a difficult task, requiring detectors to process data in real-time, and learn while simultaneously making predictions. We present a novel anomaly detection technique based on an on-line sequence memory algorithm called Hierarchical Temporal Memory (HTM). We show results from a live application that detects anomalies in financial metrics in real-time. We also test the algorithm on NAB, a published benchmark for real-time anomaly detection, where our algorithm achieves best-in-class results.
更多查看译文
AI 理解论文
溯源树
样例
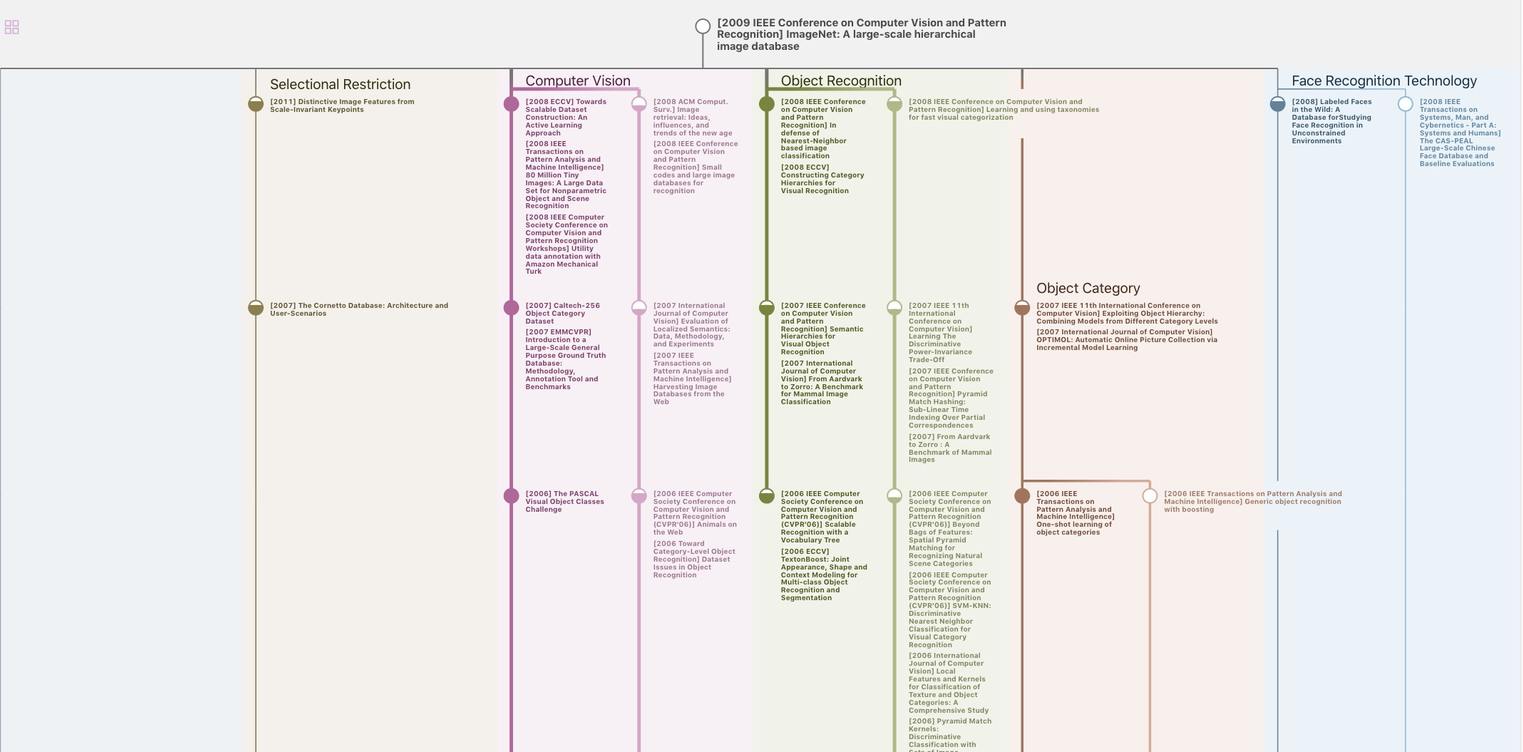
生成溯源树,研究论文发展脉络
Chat Paper
正在生成论文摘要