FLAG: Fast Linearly-Coupled Adaptive Gradient Method.
arXiv: Optimization and Control(2016)
摘要
The celebrated Nesterovu0027s accelerated gradient method offers great speed-ups compared to the classical gradient descend method as it attains the optimal first-order oracle complexity for smooth convex optimization. On the other hand, the popular AdaGrad algorithm competes with mirror descent under the best regularizer by adaptively scaling the gradient. Recently, it has been shown that the accelerated gradient descent can be viewed as a linear combination of gradient descent and mirror descent steps. Here, we draw upon these ideas and present a fast linearly-coupled adaptive gradient method (FLAG) as an accelerated version of AdaGrad, and show that our algorithm can indeed offer the best of both worlds. Like Nesterovu0027s accelerated algorithm and its proximal variant, FISTA, our method has a convergence rate of $1/T^2$ after $T$ iterations. Like AdaGrad our method adaptively chooses a regularizer, in a way that performs almost as well as the best choice of regularizer in hindsight.
更多查看译文
AI 理解论文
溯源树
样例
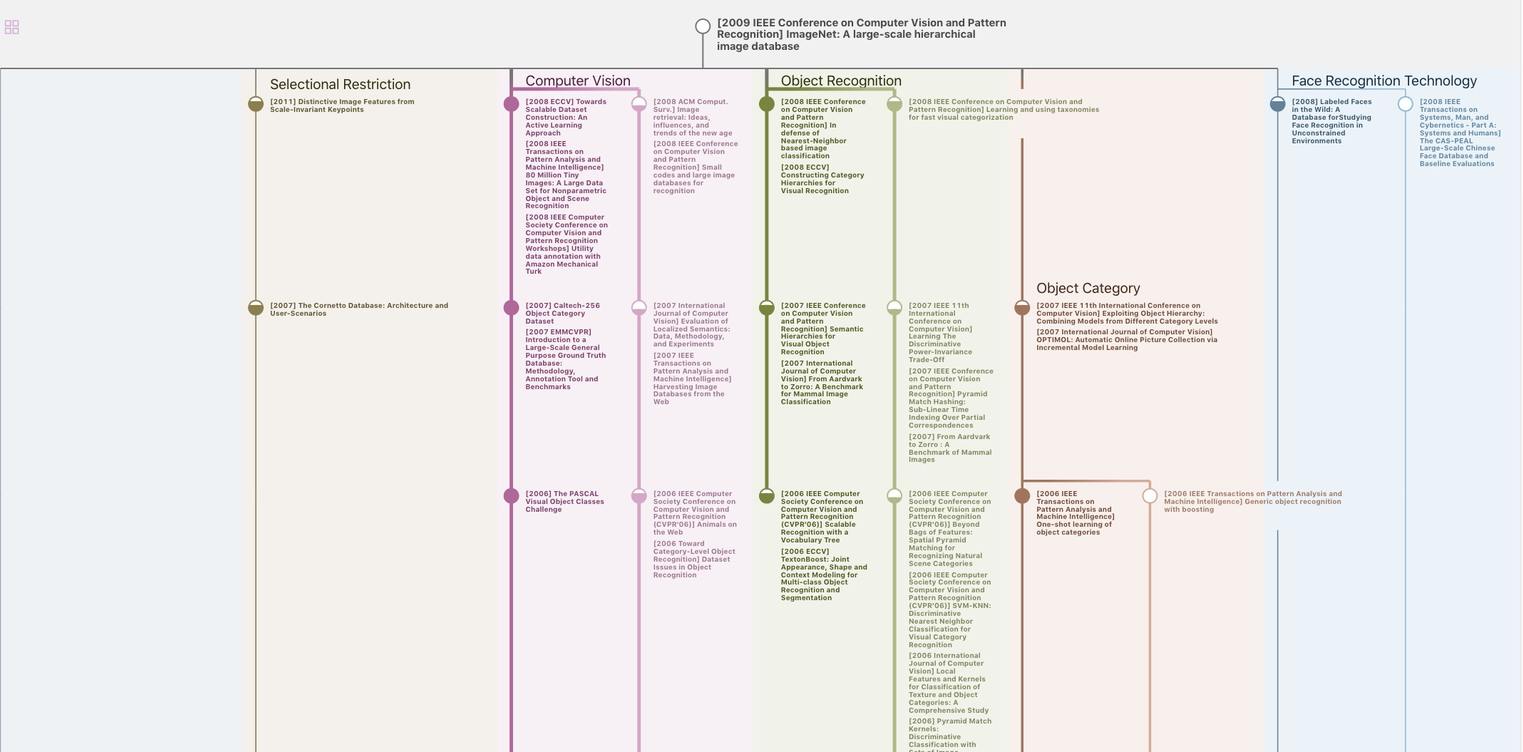
生成溯源树,研究论文发展脉络
Chat Paper
正在生成论文摘要