Finding the Topic of a Set of Images.
arXiv: Computer Vision and Pattern Recognition(2016)
摘要
In this paper we introduce the problem of determining the topic that a set of images is describing, where every topic is represented as a set of words. Different from other problems like tag assignment or similar, a) we assume multiple images are used as input instead of single image, b) Input images are typically not visually related, c) Input images are not necessarily semantically close, and d) Output word space is unconstrained. In our proposed solution, visual information of each query image is used to retrieve similar images with text labels (tags) from an image database. We consider a scenario where the tags are very noisy and diverse, given that they were obtained by implicit crowd-sourcing in a database of 1 million images and over seventy seven thousand tags. The words or tags associated to each query are processed jointly in a word selection algorithm using random walks that allows to refine the search topic, rejecting words that are not part of the topic and produce a set of words that fairly describe the topic. Experiments on a dataset of 300 topics, with up to twenty images per topic, show that our algorithm performs better than the proposed baseline for any number of query images. We also present a new Conditional Random Field (CRF) word mapping algorithm that preserves the semantic similarity of the mapped words, increasing the performance of the results over the baseline.
更多查看译文
AI 理解论文
溯源树
样例
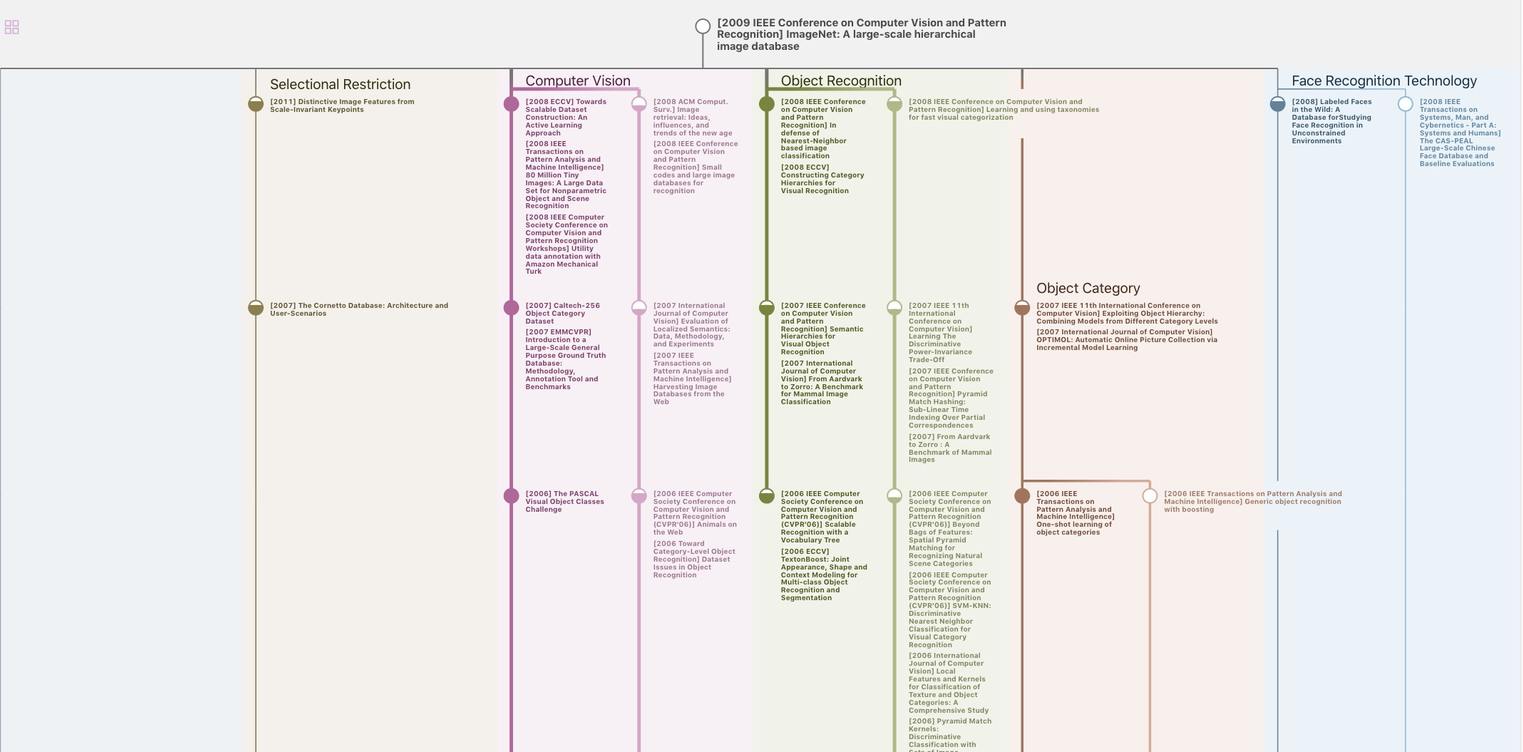
生成溯源树,研究论文发展脉络
Chat Paper
正在生成论文摘要