Learning Models For Actions And Person-Object Interactions With Transfer To Question Answering
COMPUTER VISION - ECCV 2016, PT I(2016)
摘要
This paper proposes deep convolutional network models that utilize local and global context to make human activity label predictions in still images, achieving state-of-the-art performance on two recent datasets with hundreds of labels each. We use multiple instance learning to handle the lack of supervision on the level of individual person instances, and weighted loss to handle unbalanced training data. Further, we show how specialized features trained on these datasets can be used to improve accuracy on the Visual Question Answering (VQA) task, in the form of multiple choice fill-in-the-blank questions (Visual Madlibs). Specifically, we tackle two types of questions on person activity and person-object relationship and show improvements over generic features trained on the ImageNet classification task
更多查看译文
关键词
Activity prediction, Deep networks, Visual Question Answering
AI 理解论文
溯源树
样例
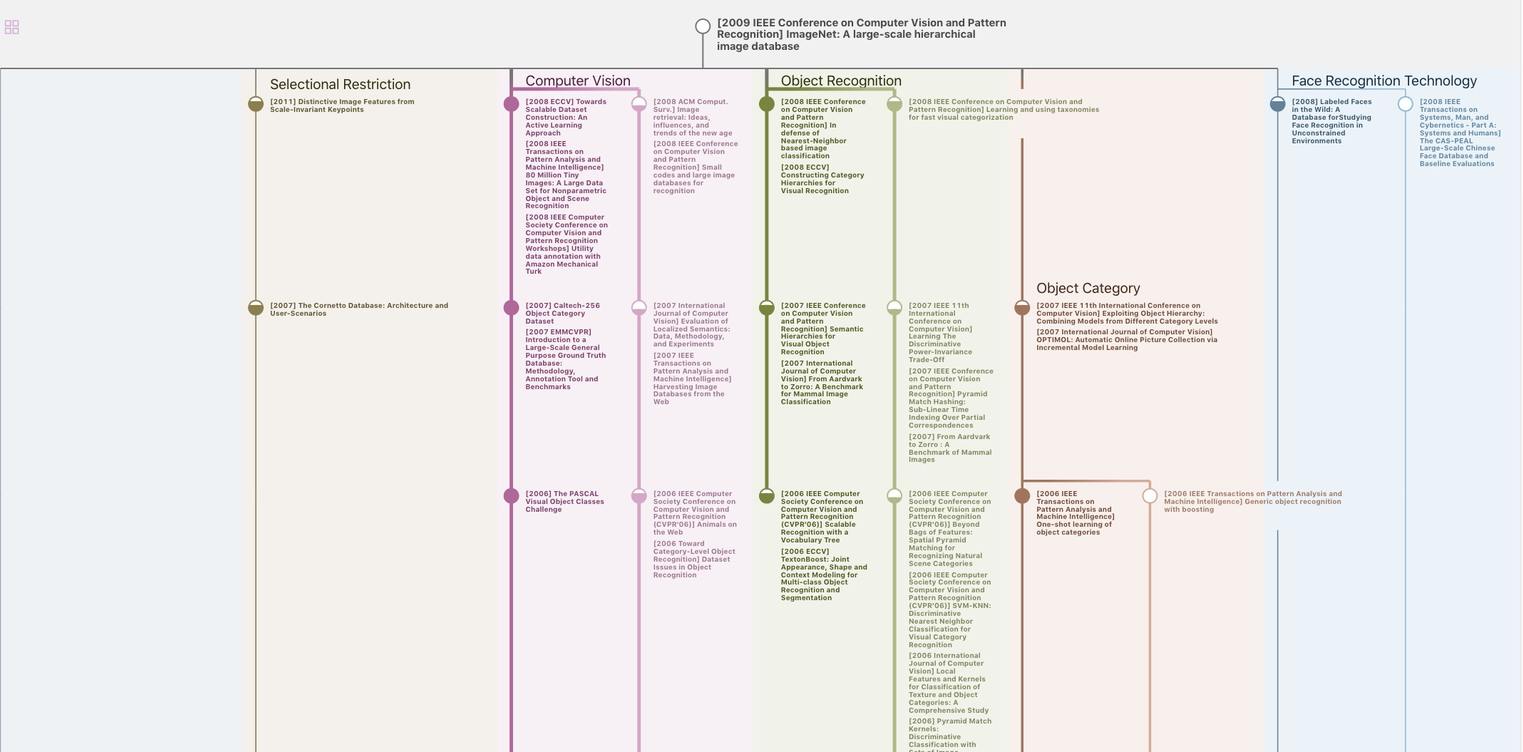
生成溯源树,研究论文发展脉络
Chat Paper
正在生成论文摘要