ScientoBASE: a framework and model for computing scholastic indicators of non-local influence of journals via native data acquisition algorithms
Scientometrics(2016)
摘要
Defining and measuring internationality as a function of influence diffusion of scientific journals is an open problem. There exists no metric to rank journals based on the extent or scale of internationality. Measuring internationality is qualitative, vague, open to interpretation and is limited by vested interests. With the tremendous increase in the number of journals in various fields and the unflinching desire of academics across the globe to publish in “international” journals, it has become an absolute necessity to evaluate, rank and categorize journals based on internationality. Authors, in the current work have defined internationality as a measure of influence that transcends across geographic boundaries. There are concerns raised by the authors about unethical practices reflected in the process of journal publication whereby scholarly influence of a select few are artificially boosted, primarily by resorting to editorial maneuvers. To counter the impact of such tactics, authors have come up with a new method that defines and measures internationality by eliminating such local effects when computing the influence of journals. A new metric, Non-Local Influence Quotient is proposed as one such parameter for internationality computation along with another novel metric, Other-Citation Quotient as the complement of the ratio of self-citation and total citation. In addition, SNIP and international collaboration ratio are used as two other parameters. As these journal parameters are not readily available in one place, algorithms to scrape these metrics are written and documented as a part of the current manuscript. Cobb–Douglas production function is utilized as a model to compute Journal Internationality Modeling Index. Current work elucidates the metric acquisition algorithms while delivering arguments in favor of the suitability of the proposed model. Acquired data is corroborated by different supervised learning techniques. As part of future work, the authors present a bigger picture, Reputation and Global Influence Score, that will be computed to facilitate the formation of clusters of journals of high, moderate and low internationality.
更多查看译文
关键词
Journal Influence Score, Journal Internationality Modeling Index (JIMI), Web scraping, Feature extraction, Cobb–Douglas production function, Convex optimization, Supervised learning, Non-Local Influence Quotient (NLIQ), Source-Normalized Impact per Paper (SNIP), Other Citation Quotient (OCQ)
AI 理解论文
溯源树
样例
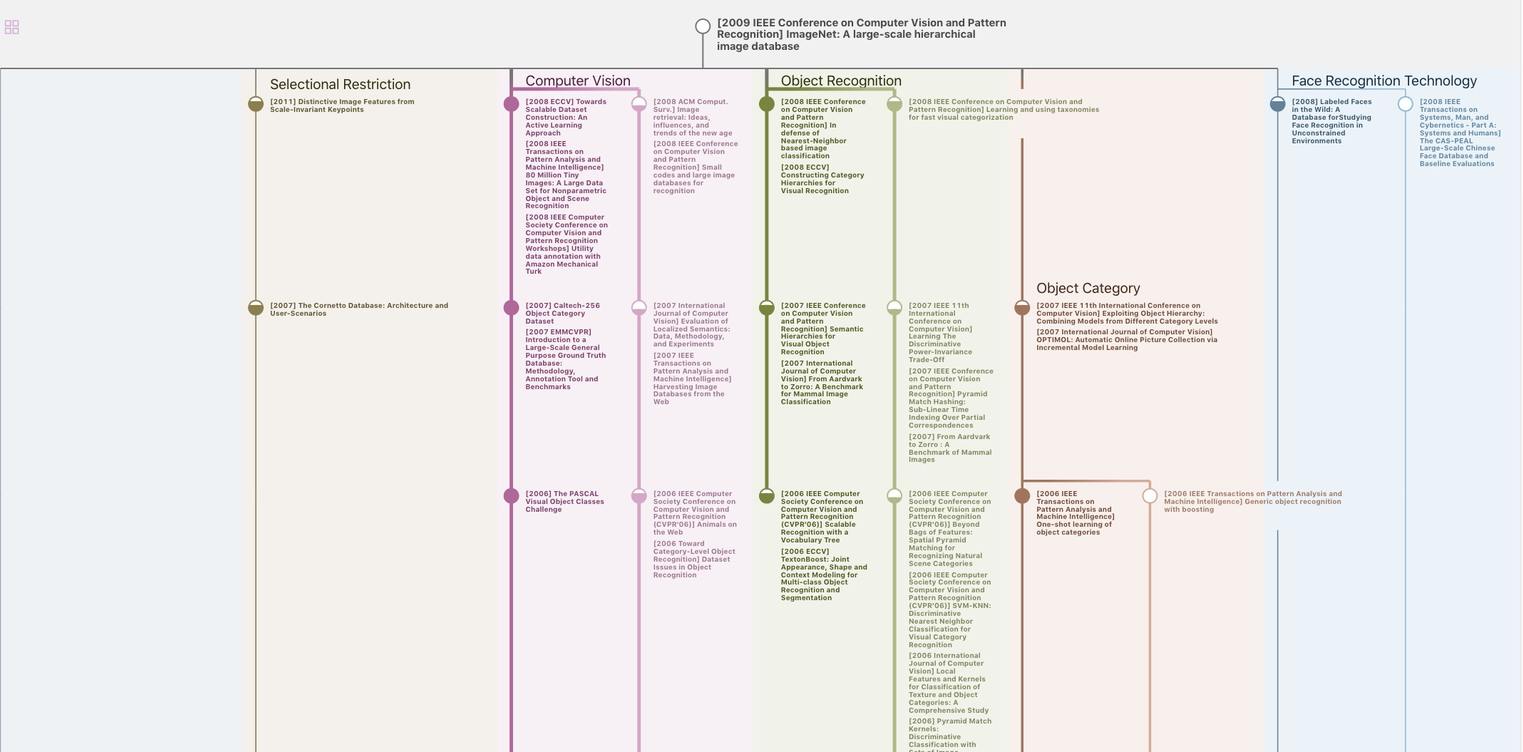
生成溯源树,研究论文发展脉络
Chat Paper
正在生成论文摘要