Demystifying Fixed K-Nearest Neighbor Information Estimators
2017 IEEE International Symposium on Information Theory (ISIT)(2018)
摘要
Estimating mutual information from independent identically distributed samples drawn from an unknown joint density function is a basic statistical problem of broad interest with multitudinous applications. The most popular estimator is the one proposed by Kraskov, Stogbauer, and Grassberger (KSG) in 2004 and is nonparametric and based on the distances of each sample to its kth nearest neighboring sample, where k is a fixed small integer. Despite of its widespread use (part of scientific software packages), theoretical properties of this estimator have been largely unexplored. In this paper, we demonstrate that the estimator is consistent and also identify an upper bound on the rate of convergence of the l(2) error as a function of a number of samples. We argue that the performance benefits of the KSG estimator stems from a curious "correlation boosting" effect and build on this intuition to modify the KSG estimator in novel ways to construct a superior estimator. As a by-product of our investigations, we obtain nearly tight rates of convergence of the l(2) error of the well-known fixed k-nearest neighbor estimator of differential entropy by Kozachenko and Leonenko.
更多查看译文
关键词
Information theory,nearest neighbor methods,mutual information,information entropy
AI 理解论文
溯源树
样例
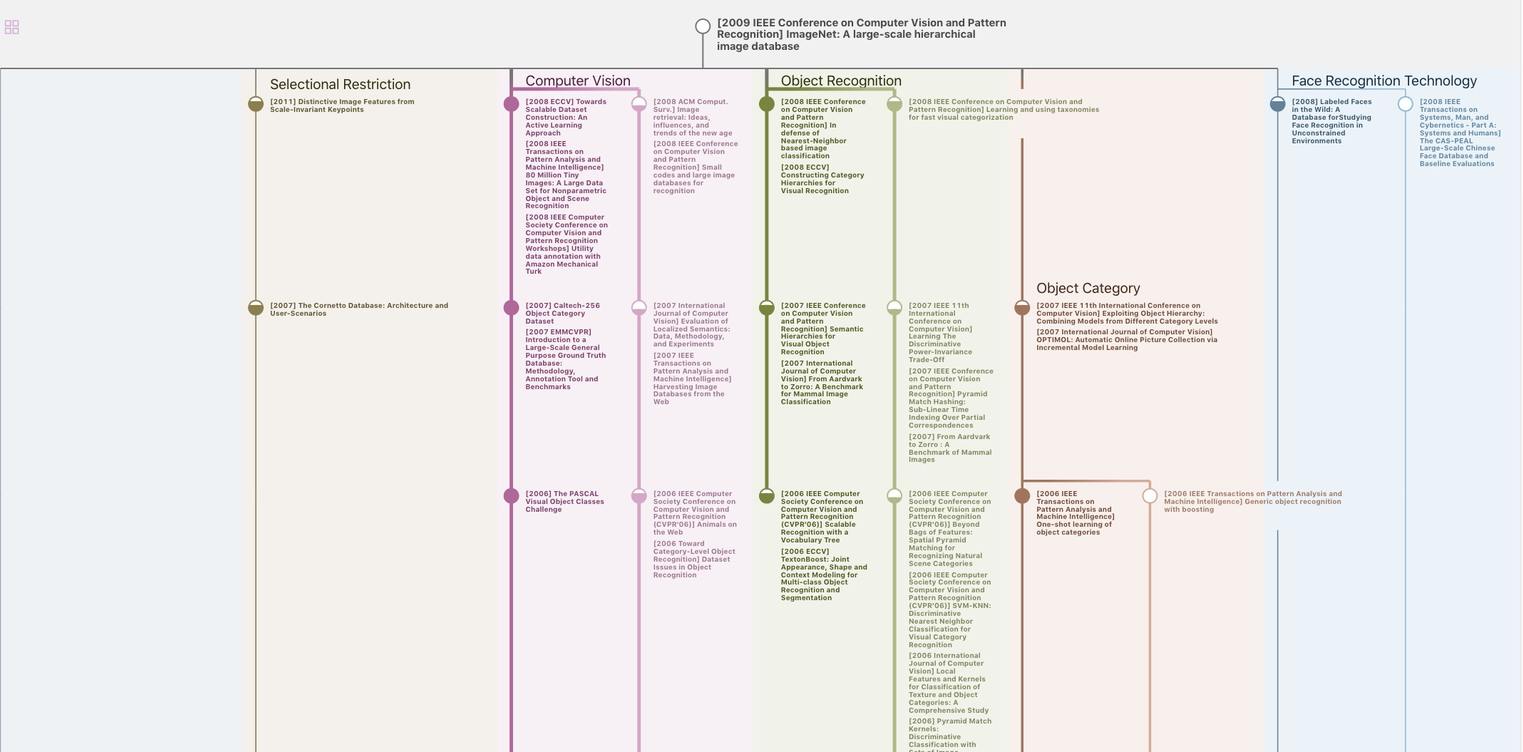
生成溯源树,研究论文发展脉络
Chat Paper
正在生成论文摘要