Annotation Order Matters: Recurrent Image Annotator for Arbitrary Length Image Tagging
2016 23rd International Conference on Pattern Recognition (ICPR)(2016)
摘要
Automatic image annotation has been an important research topic in facilitating large scale image management and retrieval. Existing methods focus on learning image-tag correlation or correlation between tags to improve annotation accuracy. However, most of these methods evaluate their performance using top-k retrieval performance, where k is fixed. Although such setting gives convenience for comparing different methods, it is not the natural way that humans annotate images. The number of annotated tags should depend on image contents. Inspired by the recent progress in machine translation and image captioning, we propose a novel Recurrent Image Annotator (RIA) model that forms image annotation task as a sequence generation problem so that RIA can natively predict the proper length of tags according to image contents. We evaluate the proposed model on various image annotation datasets. In addition to comparing our model with existing methods using the conventional top-k evaluation measures, we also provide our model as a high quality baseline for the arbitrary length image tagging task. Moreover, the results of our experiments show that the order of tags in training phase has a great impact on the final annotation performance.
更多查看译文
关键词
image captioning,machine translation,arbitrary length image tagging task,image annotation datasets,sequence generation problem,image annotation task,RIA,image contents,human annotate images,annotation accuracy improvement,learning image-tag correlation,large-scale image retrieval,large-scale image management,automatic image annotation,recurrent image annotator,annotation order matters
AI 理解论文
溯源树
样例
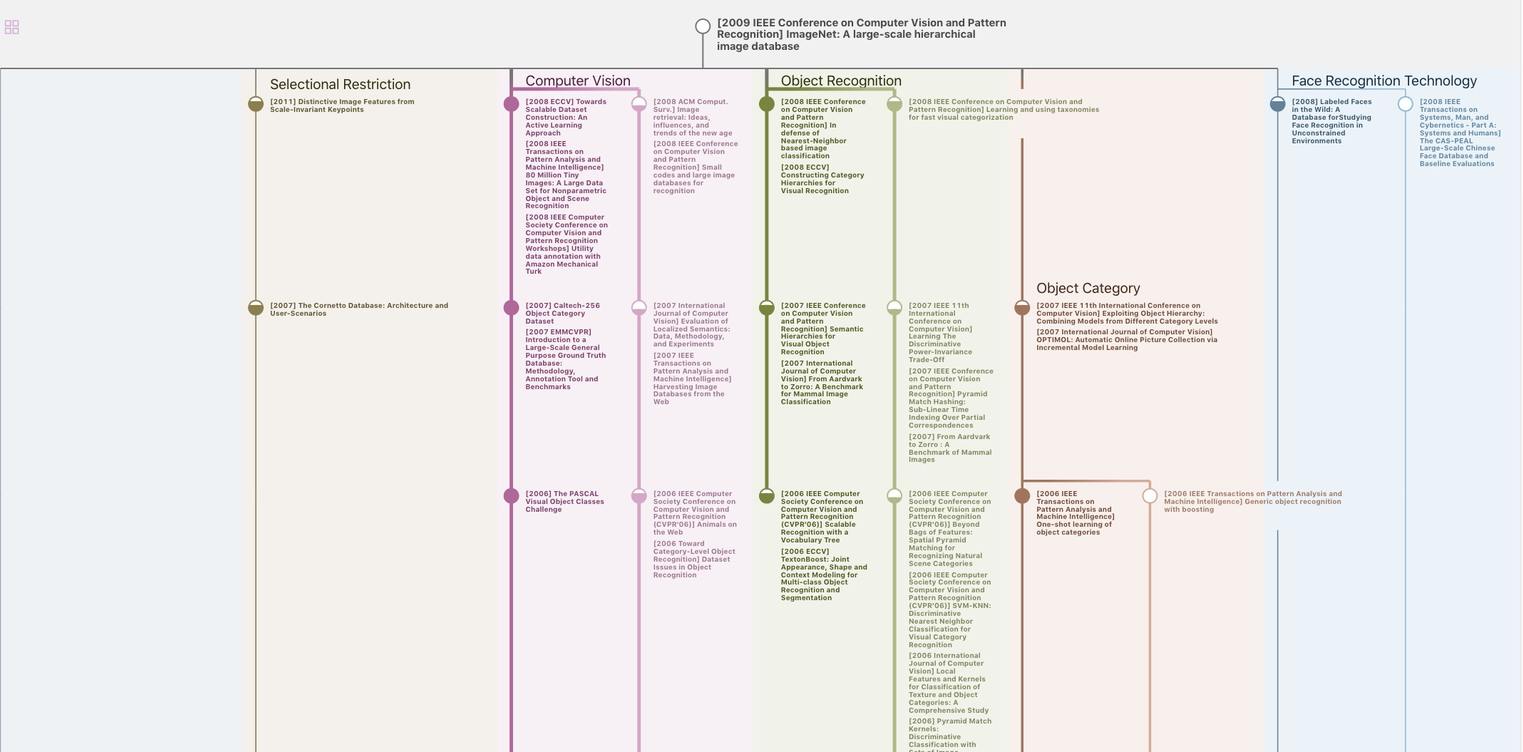
生成溯源树,研究论文发展脉络
Chat Paper
正在生成论文摘要