Training Deep Nets with Sublinear Memory Cost.
arXiv: Learning(2016)
摘要
We propose a systematic approach to reduce the memory consumption of deep neural network training. Specifically, we design an algorithm that costs O( √ n) memory to train a n layer network, with only the computational cost of an extra forward pass per mini-batch. As many of the state-of-the-art models hit the upper bound of the GPU memory, our algorithm allows deeper and more complex models to be explored, and helps advance the innovations in deep learning research. We focus on reducing the memory cost to store the intermediate feature maps and gradients during training. Computation graph analysis is used for automatic in-place operation and memory sharing optimizations. We show that it is possible to trade computation for memory giving a more memory efficient training algorithm with a little extra computation cost. In the extreme case, our analysis also shows that the memory consumption can be reduced to O(logn) with as little as O(n logn) extra cost for forward computation. Our experiments show that we can reduce the memory cost of a 1,000-layer deep residual network from 48G to 7G on ImageNet problems. Similarly, significant memory cost reduction is observed in training complex recurrent neural networks on very long sequences.
更多查看译文
AI 理解论文
溯源树
样例
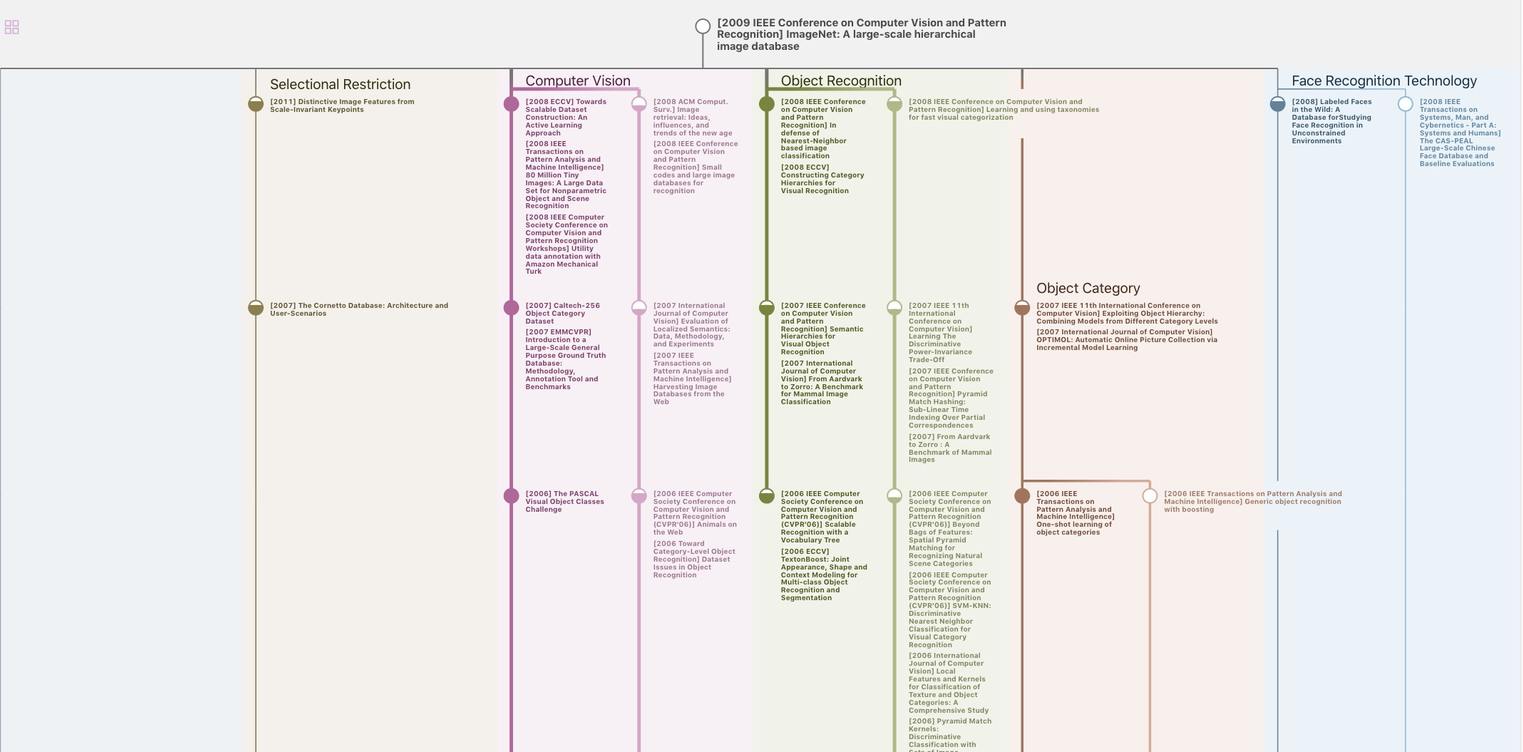
生成溯源树,研究论文发展脉络
Chat Paper
正在生成论文摘要