Learning to Rank Personalized Search Results in Professional Networks
SIGIR '16: The 39th International ACM SIGIR conference on research and development in Information Retrieval Pisa Italy July, 2016(2016)
摘要
LinkedIn search is deeply personalized - for the same queries, different searchers expect completely different results. This paper presents our approach to achieving this by mining various data sources available in LinkedIn to infer searchers' intents (such as hiring, job seeking, etc.), as well as extending the concept of homophily to capture the searcher-result similarities on many aspects. Then, learning-to-rank (LTR) is applied to combine these signals with standard search features.
更多查看译文
关键词
Learning-to-Rank,Personalization,Federation
AI 理解论文
溯源树
样例
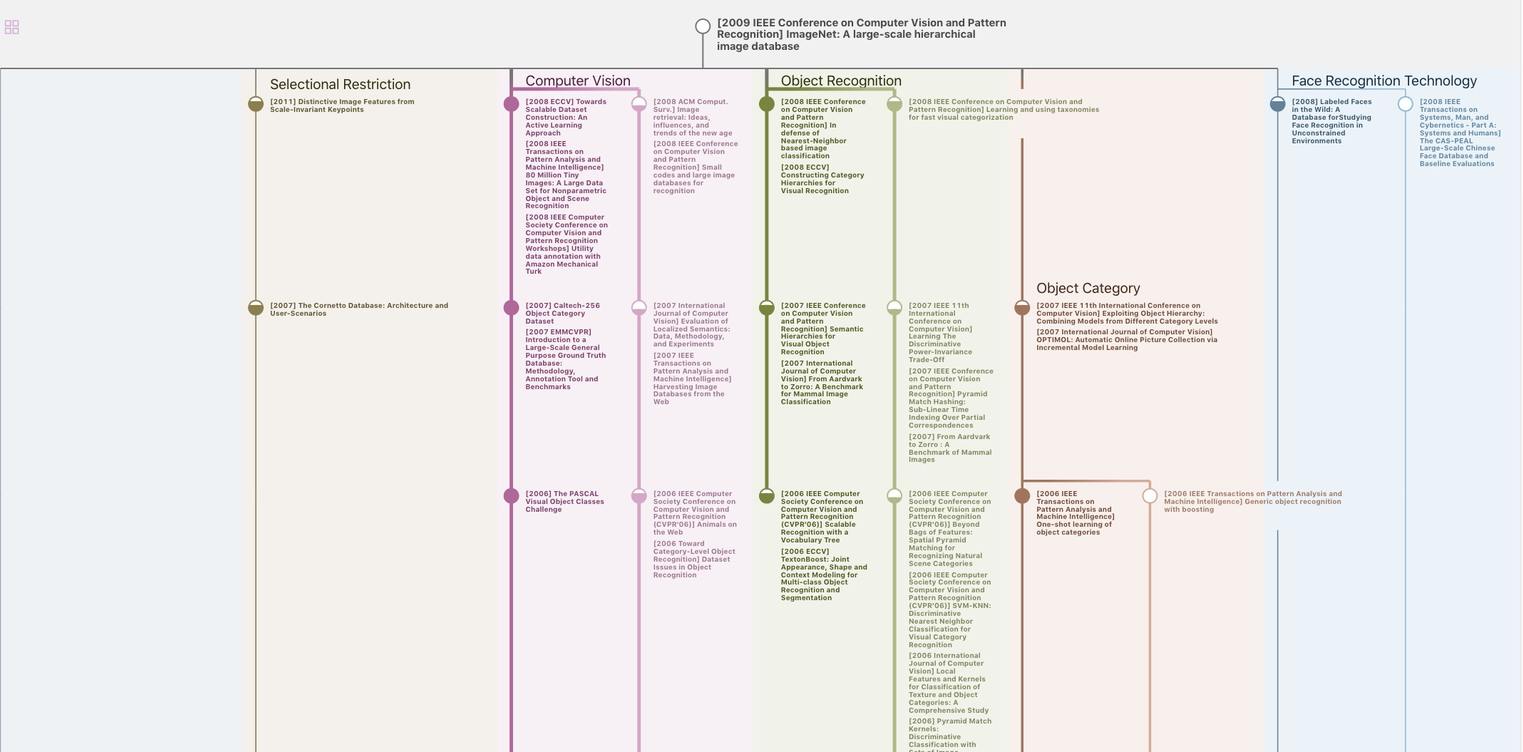
生成溯源树,研究论文发展脉络
Chat Paper
正在生成论文摘要