Micro-Expression Recognition By Regression Model And Group Sparse Spatio-Temporal Feature Learning
IEICE TRANSACTIONS ON INFORMATION AND SYSTEMS(2016)
摘要
In this letter, a micro-expression recognition method is investigated by integrating both spatio-temporal facial features and a regression model. To this end, we first perform a multi-scale facial region division for each facial image and then extract a set of local binary patterns on three orthogonal planes ( LBP-TOP) features corresponding to divided facial regions of the micro-expression videos. Furthermore, we use GSLSR model to build the linear regression relationship between the LBP-TOP facial feature vectors and the micro expressions label vectors. Finally, the learned GSLSR model is applied to the prediction of the micro-expression categories for each test micro-expression video. Experiments are conducted on both CASME II and SMIC micro-expression databases to evaluate the performance of the proposed method, and the results demonstrate that the proposed method is better than the baseline micro-expression recognition method.
更多查看译文
关键词
micro-expression recognition, local binary patterns on three orthogonal planes (LBP-TOP), group sparse least squares regression (GSLSR)
AI 理解论文
溯源树
样例
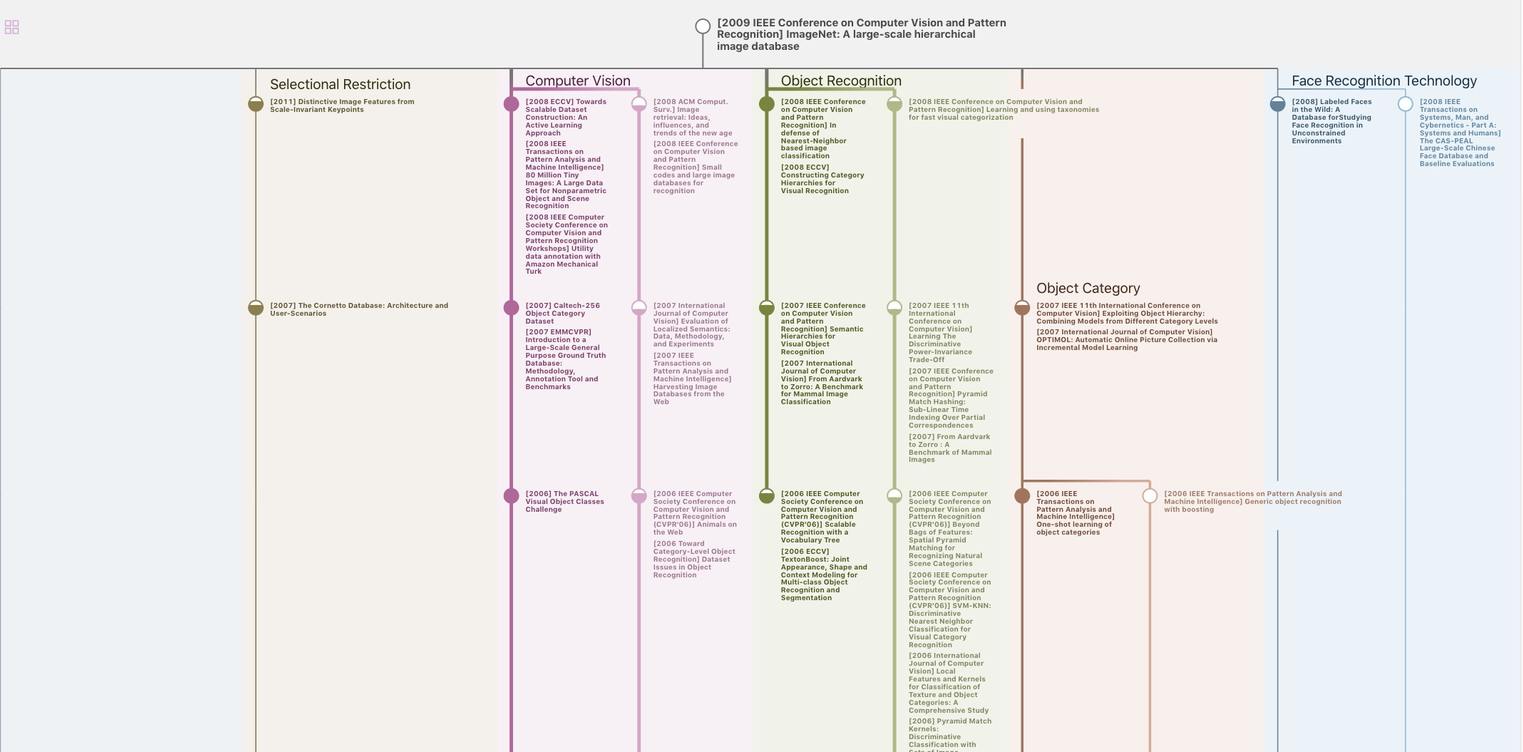
生成溯源树,研究论文发展脉络
Chat Paper
正在生成论文摘要