Fisher-regularized support vector machine.
Inf. Sci.(2016)
摘要
This paper proposes Fisher regularized support vector machine (FisherSVM).FisherSVM is a graph-based supervised learning method.FisherSVM has two regularization terms: maximum margin and Fisher regularizations.FisherSVM aims to maximize the margin and minimize the within-class scatter. Support vector machine (SVM) and Fisher discriminant analysis (FDA) are two commonly used methods in machine learning and pattern recognition. A combined method of the linear SVM and FDA, called SVM/LDA (linear discriminant analysis), has been proposed only for the linear case. This paper generalizes this combined method to the nonlinear case from the view of regularization. A Fisher regularization is defined and incorporated into SVM to obtain a Fisher regularized support vector machine (FisherSVM). In FisherSVM, there are two regularization terms , the maximum margin regularization and Fisher regularization, which allow FisherSVM to maximize the classification margin and minimize the within-class scatter. Roughly speaking, FisherSVM can approximatively fulfill the Fisher criterion and obtain good statistical separability. This paper also discusses the connections of FisherSVM to graph-based regularization methods and the mathematical programming method to Kernel Fisher Discriminant Analysis (KFDA). It shows that FisherSVM can be thought of as a graph-based supervised learning method or a robust KFDA. Experimental results on artificial and real-world data show that FisherSVM has a promising generalization performance.
更多查看译文
关键词
Fisher regularization,Support vector machine,Kernel trick,Maximum margin regularization,Fisher discriminant analysis
AI 理解论文
溯源树
样例
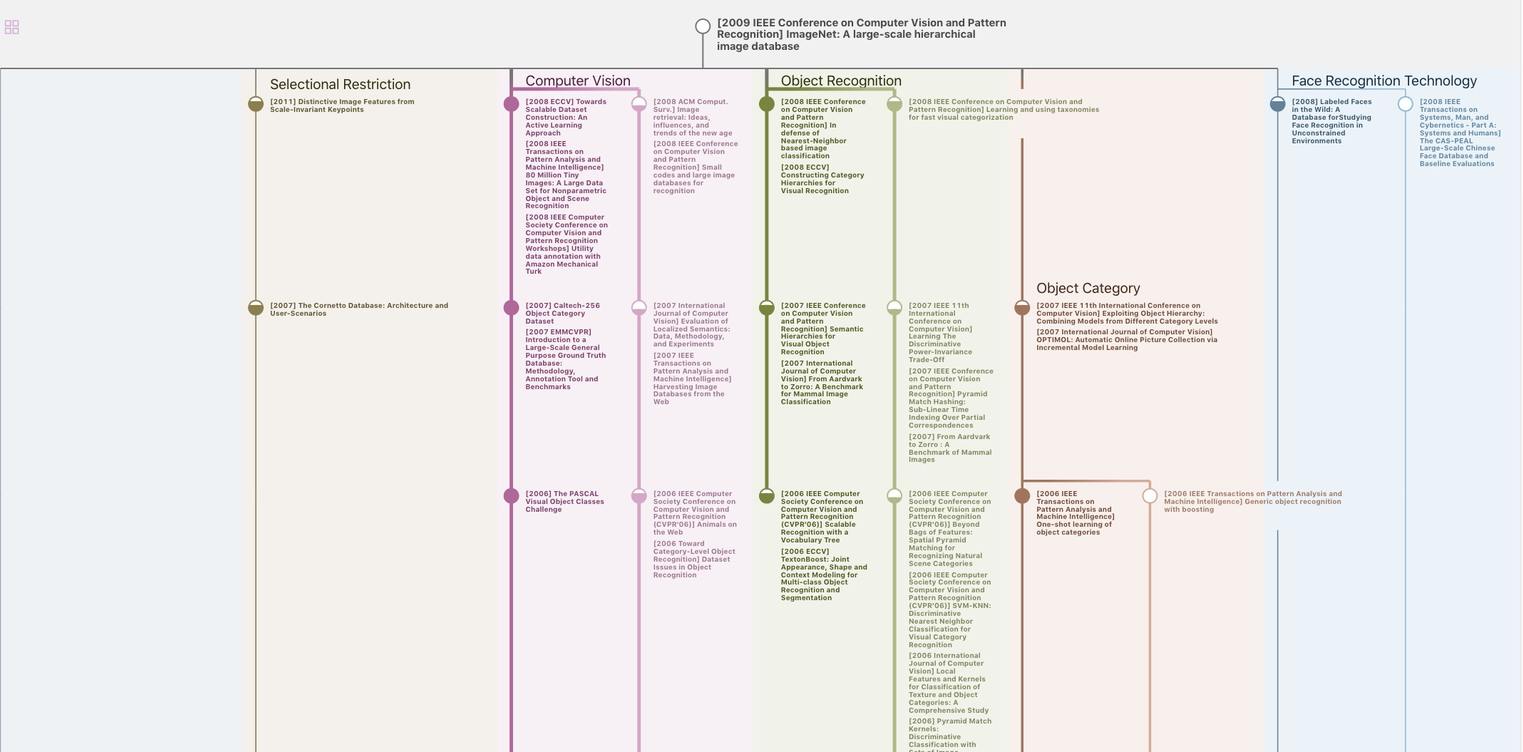
生成溯源树,研究论文发展脉络
Chat Paper
正在生成论文摘要