An incremental dependency calculation technique for feature selection using rough sets.
Inf. Sci.(2016)
摘要
In many fields, such as data mining, machine learning and pattern recognition, datasets containing large numbers of features are often involved. In such cases, feature selection is necessary. Feature selection is the process of selecting a feature subset on behalf of the entire dataset for further processing. Recently, rough set-based approaches, which use attribute dependency to carry out feature selection, have been prominent. However, this dependency measure requires the calculation of the positive region, which is a computationally expensive task. In this paper, we have proposed a new concept called the \"Incremental Dependency Class\" (IDC), which calculates the attribute dependency without using the positive region. IDCs define the change in attribute dependency as we move from one record to another. IDCs, by avoiding the positive region, can be an ideal replacement for the conventional dependency measure in feature selection algorithms, especially for large datasets. Experiments conducted using various publically available datasets from the UCI repository have shown that calculating dependency using IDCs reduces the execution time by 54%, while in the case of feature selection algorithms using IDCs, the execution time was reduced by almost 66%. Overall, a 68% decrease in required runtime memory was also found.
更多查看译文
关键词
Rough set theory,Feature selection,Dependency,Reducts,Genetic algorithms
AI 理解论文
溯源树
样例
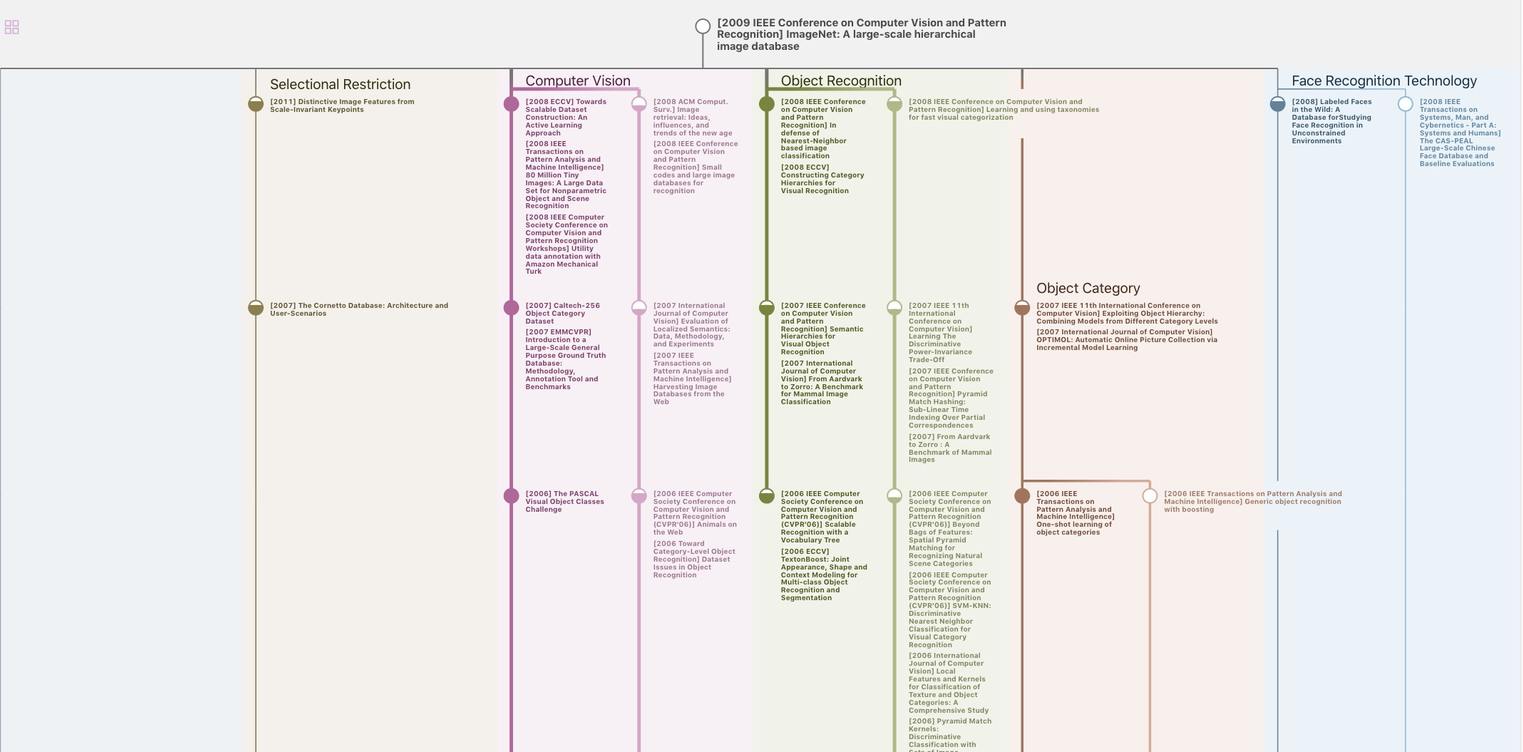
生成溯源树,研究论文发展脉络
Chat Paper
正在生成论文摘要